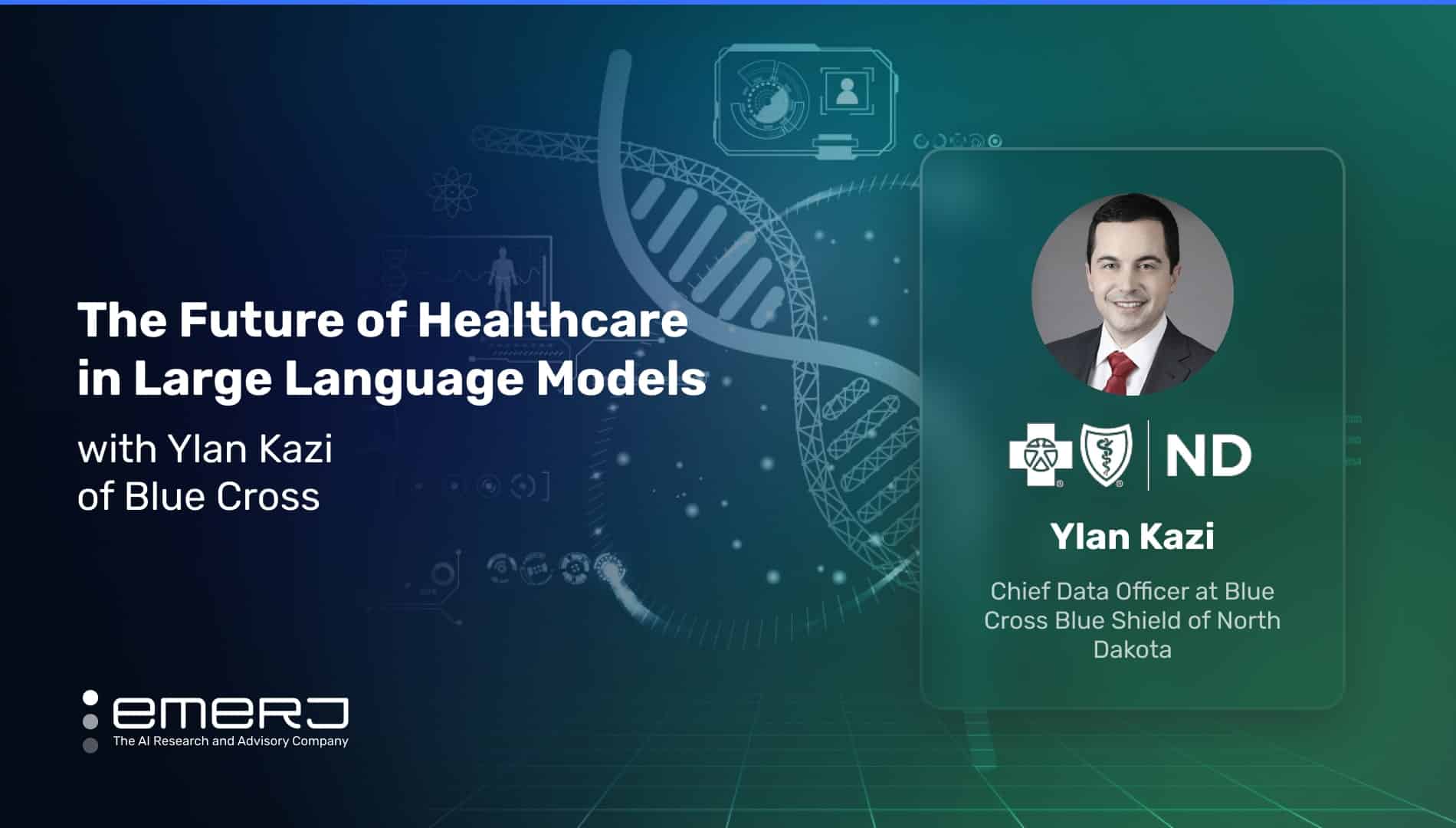
In just the last year, rapid advancements in AI technologies, particularly in natural language processing (NLP), have dramatically impacted virtually every industry. Most recently, large language models (LLMs) have become front and center – driven by the overwhelming popularity of OpenAI’s ChatGPT.
These advanced models, rooted in NLP, have captivated business leaders across various industries and left individuals awestruck by their capabilities. According to data compiled by the UBS, ChatGPT users grew by 9,900% in 60 days, setting the record for the fastest-growing platform in history.
From finance to retail, entertainment to education, LLMs like ChatGPT have, in a concise time frame, incited paradigm shift-level changes to these sectors.
Understandably, healthcare remains one of the more cautious industries to jump feet first onto the AI adoption train. When in 2016, Houston’s MD Anderson Cancer Center scrapped its $62 million partnership with IBM Watson after the cognitive computing system produced incorrect and unsafe recommendations to patients, the setback for AI in healthcare was significant – and long-lasting.
Even still, healthcare is an industry that many in the field believe is painfully overdue for transformation. Dr. Arta Bakshandeh, Chief Medical Informatics Officer for Alignment Health, summarizes the current state of the industry as far as digital transformation is concerned:
“Healthcare, unfortunately, has sat idle for years in SQL servers, I mean, there’s still some people working off a DOS – so think about a blinking cursor sitting there… what are you really going to do with that? Nothing,” Bakshandeh tells Emerj.
Most recently, the inevitability of AI in healthcare has begun to shine through, with practices discovering they can unlock valuable insights from vast amounts of clinical data in a fraction of the time, improve diagnostics, tailor treatment plans, and empower patients with personalized and accessible information.
Emerj recently sat down on the ‘AI in Business’ podcast with Ylan Kazi, Chief Data Officer for Blue Cross Blue Shield, to explore how LLMs hold the potential to extract valuable insights from medical literature to expedite traditionally lengthy discovery processes, improve diagnostic accuracy, enable personalized treatment plans, and streamline administrative tasks.
- Accelerating and personalizing drug discovery and distribution: Expediting the processing and bringing drugs to market using LLMs to filter out drug target candidates with low potential for market success.
- LLMs for collaboration with clinicians: Utilizing LLMs for platforms that serve as practitioners’ assistants, automating administrative tasks and augmenting decision-making processes toward results-based outcomes in patient care workflows.
Listen to the full episode below.
Guest: Ylan Kazi, Chief Data Officer, Blue Cross Blue Shield ND
Expertise: healthcare data science, AI, NLP, LLMs, data governance, patient outcomes
Brief Recognition: Ylan Kazi is a seasoned data scientist with extensive experience in leveraging AI technologies to drive innovation in healthcare. As the Director of Data Science at Blue Cross, he spearheads initiatives to harness the power of LLMs for improving patient outcomes and advancing healthcare practices.
Accelerating and Personalizing the Drug Discovery and Dispensing Process with LLMs
As much of the world has observed in recent months with ChatGPT, the capabilities of LLMs extend much further than the ability to provide rudimentary answers, mere translation or content creation. When utilized in healthcare, LLMs can offer valuable insights, analyzing patient symptoms expressed in their own words, identifying patterns in medical reports, or predicting potential health risks based on personal health data.
These advances offer a palpable shift from broad-brushed, reactive treatment strategies to precision medicine. This approach considers the complex web of genetic, environmental, and lifestyle factors that distinguish one person from another. These tools provide healthcare practitioners with the means to parse intricate web and predict health outcomes with an accuracy that was once beyond our reach.
According to Kazi, one area of particular interest is in the drug development process. Traditionally, the drug discovery journey takes seven years to a decade, often yielding minimal success rates:
According to Kazi, the current reality of the drug discovery process is a demanding and protracted journey, typically lasting between seven to 10 years and occasionally even longer. Additionally, the results frequently fail to meet expectations, with Kazi estimating that only about one out of every 100 drugs discovered proves successful.
Kazi’s insights shed light on the formidable challenges faced by researchers in the pharmaceutical industry. The significant investment of time and resources, coupled with such a low success rate, highlights an urgent need for continuous innovation and advancements in research methodologies.
With recent advances in machine-learning models such as ChatGPT, researchers have the potential to change this long-standing healthcare narrative on several critical fronts completely. By focusing on ongoing innovation and modernizing their methodologies, the industry can work towards improving the odds of discovering drugs that meet the stringent requirements for success in the field.
Additionally, a drug discovery’s likelihood of being beneficial and therapeutic can also be increased since impossibly large bodies of data can be analyzed in far less time than it would take humans to process.
Kazi points to the potential of AlphaFold, developed by DeepMind and Google, to predict critical factors such as molecular interactions, enabling researchers to expedite drug discovery and filter out candidates that may not meet market requirements to deliver the desired therapeutic benefits.
“AlphaFold can actually predict how many of these interactions occur, which is pretty fascinating,” says Kazi.
“By being able to do that by plugging in these solutions as part of that drug discovery, it really has the potential to not only shorten the time to drug discovery, but also to weed out much earlier, some of those potential drugs that may not have the right market, or may not deliver on the research.”
Kazi further delves into drug research and delivery, highlighting the need to move away from the one-size-fits-all approach. He questions the current practice of prescribing the same dose of medication to individuals with different body sizes, asking, “If I need an antibiotic, why am I given the same dose as maybe somebody twice my size?”
LLMs, combined with genomic information, can potentially identify individual characteristics that influence the efficacy of specific therapeutics, paving the way for individually tailored treatment plans:
“There could be things within our genes or genome that would make certain therapeutics more effective or less effective, depending on just those individual characteristics,” he says.
Harnessing LLMs as a Collaborative Tool – Rather Than Replacement – for Clinicians
When considering the recent reticence about the widespread adoption of AI and machine-learning technologies in healthcare, Kazi draws parallels to the time when personal computers began to saturate the workplace.
Initially, the prevailing fear was that computers would take people’s jobs. Instead, Kazi says,” It made people a lot more productive – it also took away some of the tasks that would take hours, days, even months to do,” he adds.
Similarly, Kazi maintains that the recent advances and the integration of LLMs in healthcare point not to the imminent replacement of human doctors or researchers but instead offer the potential for healthcare providers to focus on the most critical aspects of their job by automating some of the most repetitive tasks, or in researching vast bodies of medical literature.
While these changes in the industry may feel to outsiders as if they happened overnight, Sisense Chief Strategy Officer Scott Castle tells Emerj that the sector has been squarely focused on finding more efficient ways to put the correct data in the hands of the right healthcare professionals for the better half of a decade.
The challenge, he tells Emerj, is to develop systems that bring out the best in what humans can offer the process:
“Going back to 2017, that shift has been away from getting analytics to users and towards getting insights to users so they can explore. And there’s an important distinction: the right analytics will bring me a whole lot of data, aggregate it and process it to some extent so it becomes practical. But those analytics will also let me figure out what’s interesting, what’s important, and what I should pay attention to. Then I’ll go through and explore it. Humans on their own are bad at that.”
– Sisense Chief Strategy Officer Scott Castle
The potential for integrating AI technologies such as LLMs in healthcare reaches much further into personalizing the patient experience beyond drug development.
Kazi recognizes the ubiquity of mobile technology and the role it could play in improving diagnostics and enhancing the overall healthcare experience. He explains how smartphones can become even more intelligent healthcare devices equipped with sensors and diagnostic capabilities.
“From the providers’ side, wouldn’t it be great to have more of that information so that when the patient comes in, you have a full profile of that individual and can make better informed clinical decisions?”
Kazi also acknowledges the ever-more complex challenges of governance and privacy regarding patient health data. While emphasizing the need to protect patient information, he advocates for increased patient access to their own data. He continues to state plainly that he believes that hospitals and healthcare institutions need to evolve in their approach to data management:
“I think we’ll get to a point where enough people are demanding it that it’s going to create more of that pressure for hospitals to change how they think about data while also balancing the fact that this is individual patient data – it just seems strange to me that you can’t get access to your own data like that,” Kazi says.
“I think we are going to hit that peak of having enough people demanding it that hospitals and the healthcare industry will have to think differently about data.”