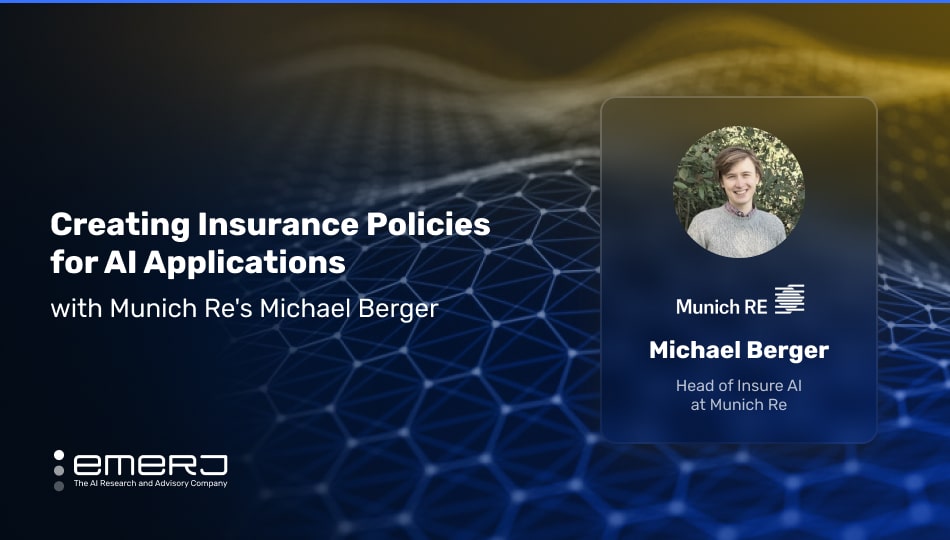
As AI becomes increasingly interwoven in the fabric of our everyday lives, the insurance sector is finding new business challenges and opportunities in all of the ways these emerging technologies bring increased risk – and security – to our lives.
Founded in 1890, Munich Re is one of the world’s leading reinsurers. In addition to reinsurance, they also provide primary insurance and insurance-related risk solutions. Munich Re also provides solutions to artificial intelligence providers.
The AI solutions that Munich Re insures are not limited to self-driving cars and medical diagnostics. MunichRe offers aiSure™ as a way for AI providers to offer their clients an AI insurance-backed performance guarantee. They also offer aiSelf™, a tailor-made insurance product for AI vendors guaranteeing the reliability of their solution.
Emerj CEO and Head of Research Daniel Faggella recently sat down with Michael Berger, Head of Insure AI at Munich Re, on the ‘AI in Business’ podcast to discuss insuring AI products and the challenges involved.
This article will examine three key insights from their conversation:
- Guaranteeing efficiency of clients’ AI solutions: Creating new products like structure guarantees for AI products using client involvement.
- Identifying risks inherent with large AI deployments: Monitor risks in AI products to price insurance products for AI accurately.
- Creating incentives to report results truthfully: Aligning payout functions with potential shortfall.
Listen to the full episode below:
Guest: Michael Berger, Head of Insure AI at Munich Re
Expertise: Insurance, technology, data management, technology-based risk assessment.
Brief Recognition: Michael has spent the last 15 years at Munich Re, helping to mold their Insure AI operations. He holds a Master’s degree in Information and Data Science from UC Berkeley and a Master’s in Business Administration from the Bundeswehr University Munich, PhD in Finance.
Guaranteeing Efficiency of AI Solutions for Clients
Michael begins by explaining how his company’s clients have a guaranteed return on the investment of an AI project.
He explains how Munich Re establishes trustworthiness in terms of how robust their client’s AI solution is, starting by working with AI providers to structure guarantees. Companies that build AI models and sell the predictions of those models are focused on how well their AI is performing, usually through third-party validation or some form of certification.
Predictive performance, in particular, matters when there is a substantive financial downside for the user. Berger tells Emerj guarantees must include the following provisions for end users:
- They should benefit the end user.
- The end user should expect that the error rate is below a certain threshold.
- End users are made aware they will receive financial compensation if the error rate exceeds the predetermined threshold.
Munich Re insures products that involve a loss of life, as well as products where there is an investment big enough that there is a substantial economic consequence when things don’t go smoothly. Companies can benefit from structured guarantees even if they’re not working on technologies where lives are at risk, such as autonomous vehicles or medical diagnostics.
Berger elaborated that Munich Re can create guarantees around purely operational scenarios or optimized processes.
Costs are incurred if the AI doesn’t work as expected, whether by shutting down the AI or if additional workers are needed to do the tasks the solution was performing. The basic process of devising a realistic risk threshold and agreeing to payment and terms involves looking at the scope of validity regarding where the AI model is valid.
Berger also notes that there are limitations to the guarantees Munich Re offers. Establishing guarantees requires establishing specifications for which use cases are valid. If a client uses a machine learning model outside those specifications, Munich Re cannot guarantee performance.
Berger mentions, “Ultimately, where the machine learning model can be used, and where it really delivers a robust predictive performance, that’s something we figure out together with the AI provider.”
Munich Re is interested in how their clients look at the performance of their machine learning models. Then Munich Re takes a statistical measurement that they use to determine the metric’s probability distribution. They base their determination on the following:
- Their risk assessment of the data science process.
- Their evaluation of a statistically-sound testing procedure for the machine learning model.
Ultimately, they are interested in how the probability distribution for this metric varies. The guarantee threshold they set depends on finding a good representation of this probability distribution.
Identifying Risks Inherent with Large AI Deployment
Some of the risks inherent in large AI deployments include the following:
- Business damage
- Reputational damage
- Legal risks
- Physical risks
Berger explains that Munich Re has a team of research scientists at their headquarters in Palo Alto, CA, that works with client data science teams and different domain experts depending on the specialty that is being insured. When Munich Re is working with a client that offers machine learning models to predict malware, they involve their cybersecurity experts.
Munich Re creates a living document by updating risks based on changing, deployed AI applications. On an enterprise level, deployed applications change for multiple reasons, including:
- Exposure to data types
- Changing popularity of data types
- The way algorithms are used
Berger describes a Munich Re client that conducted toxic content moderation. They built machine learning models to classify harmful content on social media platforms, including posts for weapons or drug sales. The client needed their solution to prioritize critical posts for their moderation team and implemented an AI solution to deliver that.
The economic benefit of a high-performing AI model comes in the form of reduced work for the social platform’s moderation team. If the AI model doesn’t perform as promised, more moderators might be needed, and those moderators might need to work longer hours. In such cases, the guarantee would pay out and compensate for the resulting economic shortfall.
Creating Incentives to Report Results Truthfully
Ground truth refers to real-world data used to train and test AI models. Establishing ground truth enables Munich Re’s clients to perform a check to determine how a model’s performance is evolving.
Berger explains to Faggella on the podcast that Munich Re collaborates with their clients to determine where their machine learning model can be used. The resulting assessment requires looking into every step of the data science process, including:
- Data generation
- Data annotation
- Ensuring the annotation process remains stable throughout the life of the model
- Training and testing procedures
- Monitoring and retraining
Establishing ground truth is often the first way insurers can fight fraud. According to the National Association of Insurance Commissioners, fraud costs consumers and businesses more than $300 billion annually.
Insurance companies devote a substantial amount of financial resources to combat fraud. Following the onset of the COVID pandemic, companies saw increased fraud. Fortunately, Munich Re is well-versed in identifying fraud.
Berger explains that one way to address the potential for fraud or exaggerating a model’s failure is that the payout function is a function of the shortfall. Munich Re aligns these by considering how well the AI performs and how far it falls below expectations.
Another way, Berger continues, is how providers approach testing in production. Munich Re’s clients are the AI providers, and those providers will lock a model’s predictions and also get access to the ground truth.