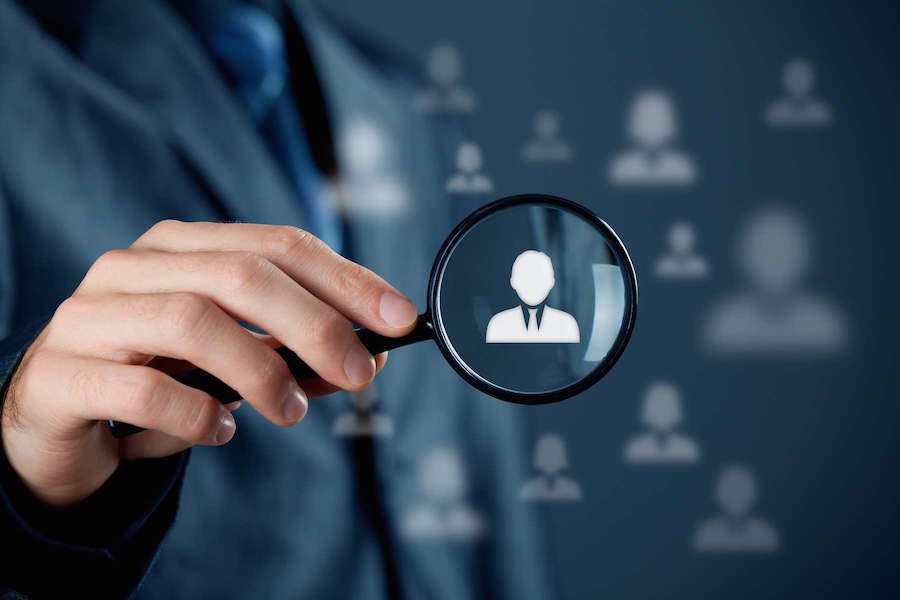
In a study from Researchscape International, 74 percent of 300 marketers surveyed said personalization in this field has strongly helped their clients or companies in advancing customer relationships. It also found that 54 percent said their clients or companies experienced a 10 percent increase in sales, while 13 percent of marketers surveyed reported a more than 30 percent lift.
As a result, 97 percent of those surveyed say they will continue to allot and invest in personalized marketing efforts. Additionally, 77 percent of respondents believe personalization should be prioritized in their organization.
What are the advantages that personalization offers to the businesses?
According to the above-noted research, AI-driven personalized marketing applications could make it easier for businesses to communicate with target markets by using data gathered by behavior-based algorithms and predictive analytics. As a result, the messaging for one customer could also applied to a segment, possibly allowing for personalization at scale.
AI-powered multisensory applications — those that analyze voice, image recognition and behaviour — could potentially help businesses learn more about customers’ needs and expectations, deliver the right recommendations, and open more business opportunities.
In this report, we have classified industry applications of personalized marketing into four clusters:
- Ad Targeting – Placing ads based on demographics, as well as consumer buying history or behavior
- Personalized Messaging – Using subscriber data to tailor email content to the individual
- Product Recommendation – Software that analyzes data to make suggestions for something that might interest a user
- Dynamical Websites – Sites where webpage content changes depending on a visitor’s purchase data and other live or past behavior on the site
With this report, we aim to give business leaders a insights on AI-driven personalized marketing applications through case studies, leadership background and other information.
Ad Targeting
The below applications use AI for the purposes of targeting ads to a specific user based on his or her online shopping behavior, which could include clicking on, viewing or purchasing various products.
ReFUEL4
ReFUEL4’s Ad Analyzer claims to use computer vision to “predict” an advertisement’s performance. This Ad Analyzer comes packaged for free with the ReFUEL4’s overall marketing service. The company says the Analyzer can be used by clients to develop new ads when an existing ad’s performance declines. This type of decline, called fatigue, happens when the target audience becomes too familiar with the ads and stops paying attention to them, according to the company.
To start using the app, the client-side user, such as a company owner or marketing head looking to promote their business, may log into ReFUEL4’s online site and create their campaign brief, which includes their goals and what they are aiming to market.
This information will be sent to ReFUEL4’s human design team who will submit multiple news ads to the client within 48 to 72 hours. To test how ReFUEL4 ads, or client-created ads, will perform, the client can use Ad Analyzer by dragging and dropping the ad into it’s section. The company claims its predictive analytics program, called SHAKA, works to forecast the ad’s success based on design, such as styling or color; target audience data; and historical data of similar ads.
The analyzer’s computer vision algorithms will also test the new ads for adaption to mobile, tablet or PC screens, resolution and operating systems, according to the company.
Once the testing and ad selection is done, the client can request final revisions for the ads they plan to use, as explained in the 1-minute video below.
The company claims that, if a client needs no ad revisions, the Ad Analyzer can also be used to automatically send the new ads to the sites they will be presented on, while replacing the underperforming versions. ReFUEL4’s website reports that the ads can be used on the Google Display network, Youtube, Facebook, Snapchat and Instagram.
According to the company website, the company’s predictive algorithms have an 87 percent accuracy and provides more precise results in A/B testing.
According to a case study, Yes.Fit, a ReFUEL4 client, needed to produce a high volume of ad creatives for successive advertising campaigns that spanned several months. When its team had run out of ideas, the client turned to ReFUEL4 to deliver new ads aligned with the campaign objectives. The new ads underwent Ad Analyzer testing, and were ranked based on predicted performance.
ReFUEL4 reports that the new ads selected to be deployed delivered an 84-percent increase in click-through rates on Facebook campaigns, although the study did not specify previous numbers or how long the ads were deployed.
The company claims that the campaign achieved a 69-percent reduction in cost per install (CPI) from $13 to $4. CPI metrics are specific to mobile applications and calculated by dividing the ad spend by the number of new installs or downloads within the campaign period. For instance, if the company spent $100 on ads to promote the mobile app, which was installed on 100 phones, the CPI is $1.00.
Aside from Yes Fit, The company also lists Spotify, PayPal, Ebay, Lyft, Alibaba, BestSecret, Onnit, Vivint and EnjoyCompare as clients.
HanSheng Chiu is the Head of Data ReFUEL4. He holds two Master’s degrees: one in Knowledge Engineering from the National University of Singapore, and another in electrical and electronics engineering from the National Taiwan University of Science and Technology.
While we could not find any other leadership with an AI background, the staff includes data scientists, data engineers, and deep learning engineers with Masters or Doctoral backgrounds in computer science. One deep learning engineer, Zhi Lu, has received his Master’s and PhD in Computer Science from the City University of Hong Kong.
Match2One
Match2One is an advertising application that is deployed on eCommerce sites and used to prospect and retarget customers. Match2One claims it uses machine learning algorithms to target people that are likely to become customers. It also claims its engine is trained to generate leads and find new customers using a combination of site visitor behavior and historical data.
According to the company, Match2one displays ads to a customer after analyzing his or her on-site behavior which could indicate interest in a product. This could involve data such as the products they’ve browsed, clicked on or purchased during their session on the eCommerce site.
The company claims the algorithm analyzes data points that also include geography; type of device and browser used; duration and frequency of user sessions; time of day; and sites visited. The algorithm will find patterns and make comparisons of the type of people who convert to customers by making purchases. The ads will then be shown similar audiences who the program determines are most likely to convert. The better the ads perform and the higher the program’s accuracy and training quality will grow over time, according to the company.
In retargeting, Match2One also collects information about site visitors who checked the eCommerce site’s products or services but did not convert, and can be commanded to display ads only to these people. In doing this, the solution aims to remind the visitors about the product or service and to convince them to purchase or sign up for an account.
The Match2One application will also collect data about the users who checked, signed up for, or purchased the fashion site’s products. The algorithm will note the similarities among the users and keep prospecting individuals with the same attributes. The application will also show the ads again to individuals who visited the site but did not convert.
Match2One brings this information to its website, where users can log in and see a dashboard of organized information, such as customer demographics and data of groups that are considered most-likely to buy products.
To get started, the client connects their website with the platform by generating Match2One tracking links for each ad or product listing on Match2One’s website. Similarly to generating a bit.ly or Google tracking link.
A user can then place the ads hyperlinked with the tracking URLs on their website using their existing CMS platform, such as WordPress. They can also place or display them on other websites.
Once the banner ads are placed, the client can create the campaign brief within the Match2One site, provide details such as product category, name, target countries, campaign start and end dates. The application will ask the client to choose prospecting, retargeting or a mix of the two approaches, and other details such as budget or targets.
When clicked, the ad’s tracking link will begin to collect data on prospective customers as they browse the retailer’s website. Match2One’s website also provides clients with basic guidance on how to create banner ads with the use of in-apps.
The company claims it is also capable of deploying ads from within its own dashboard, as shown in this 4-minute video:
Online gaming company Better Collective wanted to create brand awareness for its platform called PariuriX in Romania, and approached Match2One to assist. The aim was to achieve a click-through rate (CTR) above 0.1 percent and a cost per click (CPC) below 30 cents.
Match2One claims that it deployed Better Collective ads to its inventory of gaming websites, but did not mention specific site names.
The study did not provide previous campaign figures, but reports that within one month of Match2One prospecting, the campaign achieved 512,000 impressions, a CPC of $0.33, and a cost per mille (CPM) of 96 cents. The client also achieved a click-through rate by 0.35 percent.
Match2One lists clients that include Epiroc, One Asia, Heymo and MotoDirect.
Match2One’s CTO Michael Dewhirst, leads the company’s AI, machine learning and machine vision efforts. His education does not specify AI-associated degrees, but he claims to have AI-based delivered projects for Deutsche Bank, JP Morgan, Vodafone and BAE
Personalized Messaging
In personalizing messages today, contextual marketing is vital. To know if the messages are resonating with the audience and deliver results, marketers need to gather data about their target audience such as behavior, demography, preferred content, pages viewed, and even social media posts, to ensure relevant messaging is delivered.
Dynamic Yield
Dynamic Yield’s email solution claims to use behavioral data — including email clicks, purchase histories and other online activities on a client’s website or mobile app — to personalize emails. Part of the solution is the capability to create dynamic email templates that will contain the relevant messages.
The application can be used by eCommerce, media, travel, business-to-consumer and gaming companies, among others, according to the company.
To setup personalization, marketers must first specify the parameters that will prompt the application to send emails. These parameters include time of day and publishing of new content. Emails could also be sent during trigger events, described by the company as events such as browser and cart abandonment within a specific site.
For example, if a site visitor decides to stop an ecommerce page from loading and abandons the browsing session, the company claims that the email application will automatically fill in a template with specific details taken from the user’s browsing session on that site and remind them to complete the action.
The company website reports that personalized emails triggered by abandonment behavior and messages containing product recommendations have the potential to move customers into purchasing and earn the business more revenue. The application also claims to conduct A/B testing where customers create variations of a message and upload these to the Dynamic Yield application.
In the 2-minute video below, CTO Liad Admon demonstrates how the machine learning algorithms will decide which message to send to which customer based on an individual’s data attributes.
The technology will eventually learn to predict the customer’s preference, in the long run potentially maximizing revenue, according to Admon.
Luisaviaroma (LVR) wanted to increase customer retention and revenue and provide both machine learning-powered recommendations and flexible merchandising rules.
Dynamic Yield used a three-pronged approach:
- Serve personalized add-to-cart recommendation emails – As shoppers browsed the LVR site, Dynamic Yield claims its software suggested other products that would match the apparel a customer had carted.
- Optimized recommendations on LVR’s “Thank You” page – After a shopper completed a purchase, Dynamic Yield reported that they added personalized recommendations for similar products to LVR’s already-existing “Thank You” page, which appeared after purchases were made.
- Deployed urgency emails across cart pages – The company claims to have sent LVR customers email messages about popular items that were running out of stock.
The study did not specify figures from previous campaigns, but claims that following this approach, LVR achieved a 15-percent average revenue per user (ARPU) increase from personalized add-to-cart recommendations. Dynamic Yield says LVR also gained a 14-percent ARPU boost on their “Thank You” pages with the add of recommendations, and a six-percent APRU increase from targeted urgency messaging.
Sephora, Hallmark and Jewelry.com are some of the company’s other clients.
Vladi Rozhavsky is the Chief Data Scientist at Dynamic Yield. Prior to this, he was a machine learning team lead and big data specialist at myThings and the Israeli Defense Force. He earned his Master of Science in applied statistics from the Tel Aviv University.
Yoochoose
Yoochoose’s is a company that provides eCommerce services that help online retailers generate a personalized a shopping experience for their customers through personalized emails that contain product recommendations and newsletters.
According to the company website, these “Targeted Notifications” are automatically triggered by customer behavior. The company reports that the solution’s behavioral machine learning algorithms can recognize if the customer recently purchased something or has been inactive for a period. The company claims that these behaviors trigger the application to send reminder and after-sales emails, respectively.
Target Notifications is part of a personalization suite that includes a recommendation engine and a personalized search. The algorithms are trained to determine who the frequent buyers are, prompting the solution to offer personalized incentives such as an additional free service, a gift, or coupon, based on the customer’s past behavior and preferences.
The client will be asked to register their eCommerce site into the YooChoose web application. Once registered, Yoochoose will as the user to configure recommendation engine by choosing the size of boxes that will appear at the bottom of the eCommerce pages, and the content (product title, image, price).
The application then creates a script that the user will apply at the bottom of the ecommerce page master template. Recommendations will appear at the bottom of the web pages.
The 4-minute video below explains how the recommendation feature can be used:
The company had no listed case study around personalized messaging, but featured a recommendation engine client success story of Nestle Schöller, a business-to-business online store.
In the study, Nestle Schöller required the recommendation engine to achieve a significant cross-sell and up-sell effect by suggesting products to customers. The engine also needed to have the capacity to collect purchasing behavior data which the marketing team could analyzed and use for personalized newsletters or the automatic generation of shopping lists.
The client success does not reveal how the recommendation engine nor the collection of data has helped marketing in its personalized newsletter. The client only claims that the engine enables them to cross-sell and up-sell recommendations to be displayed in the online store. The study claims that Yoochoose application programming interface offered a simple, quick and low-cost implementation. But no details were provided.
Yoochoose lists Janvanderstorm, Triveni Ethnics, Wein-Konzept, Heyder Exclusiv, and Etecture as its clients, among others.
The company was acquired by content technology company eZ Systems for an undisclosed amount in 2011 and has since integrated the recommendation engine into joint projects. Under eZ Systems, the personalization suite is offered as eZ Recommender Engine, which will offer eCommerce services to clients while collecting data about users.
Yoochoose retains its brand name, with Michael Friedmann continuing his role as CEO while fulfilling the role of Head of eZ Personalization at eZ Systems. He holds a Master of Science degree in Computer Science from the Karlsruhe Institute of Technology, but his AI career background is unclear.
The company claims to operate Yoochoose Labs in Israel, which conducts research and development around recommendation methods, personalization solutions and data mining technologies.
In a blog post, Michael wrote that “Larger online shops can quickly accrue millions of data records per month. NoSQL databases have been increasingly used for storage. These are helpful for collecting huge amounts of data quickly and cost-efficiently. With a long storage period, they enable powerful comparative data analysis. This wealth of historic data is the fuel that drives machine learning, and it can offer a glimpse into the future.”
Product Recommendations
Recommendation applications offer customers products tailored to their preferences, cutting the time spent finding and comparing products, and possibly saving money from buying the wrong products.
For eCommerce businesses, recommendation engines offer the potential to reduce product returns as well as increase revenue through retargeting, repeat purchases, new products, as and creating active buyers and loyal customers.
Recombee
Machine learning algorithms are at the core of Recombee’s recommendation engine, according to the company, claiming that the platform is able to deliver recommendations within 200 milliseconds of the user’s action. The company also claims that its platform can deliver more than 500 recommendations per second.
The company claims that the technology uses a combination of collaborative filtering algorithms that analyze human behavior such as views and purchases, as well as content-based algorithms for titles and descriptions. These algorithms are refined as the site visitor continues to use the site.
The company claims that the AI is capable of deciding which algorithm to use based on available data. For instance, if a regular or frequent site visitor builds a profile of interactions, the platform will utilize collaborative filtering algorithms, which trigger better recommendations.
For new visitors, content-based algorithms will be used. As the visitor builds a history of interactions within the site, the algorithms are refined.
The platform is applicable to real estate, classified ads, jobs boards/human resources, ecommerce, videos, articles, cultural events, gaming, e-learning, music, travel and writing, among others, according to the company.
The short video below shows how the Recombee recommender engine is applied to Konga.com’s product detail view and shopping cart.
When a shopper clicks on the image of a long-sleeved shirt, the selected product and its attributes are displayed on the main window of the page.Below the main image, three items under the heading “Recommended for you” also appear. When the shopper adds this shirt to the shopping cart, four other products are recommended the chosen item to be purchased.
Retailer Design Group, based in Denmark, aimed to personalize Moodings.com’s, its brand of furniture offerings. This was part of the business strategy to fully personalize the offerings and help shoppers to efficiently discover products.
The furniture line consisted of more than 10,000 products, and ecommerce personalization required a system that could recognize individual preferences, allow users to view similar and new products, and respond in real-time amid high traffic.
Recombee offered the client an engine that applied automated personalized recommendations when viewing products as well as the shopping cart. For instance, when a client clicks on a preferred product, the algorithms search for three other similar products and displays them on the right navigation with a “Also See” heading.
The algorithms search and recommend similar products in the shopping cart section under the “Others Have Also Bought” section.
The company did not provide exact figures and timelines in the study, but claims that since applying Recombee’s recommendation engine, purchases have increased 52 percent.
Aside from the Design Group, Recombee also lists as clients other brands such as Konga, Much Better Adventures, Chico Rei, Showmax, Keyflow, NewsBytes, and E.Conomia.
CEO Pavel Kordik earned his Doctorate in Data Mining from the Czech Technical University (CTU) in Prague, where he worked as a Vice Dean at the Faculty of Information Technology for more than 14 years prior to Recombee. While at CTU, he lectured about the fundamentals of artificial intelligence, recommender systems, data mining, and evolutionary computation.
Sentient Aware
Sentient Aware offers a recommendation engine which it claims uses the customer’s visual and behavioral interactions to drive the deep learning algorithms within the eCommerce site.
In turn, the intent and curation-driven algorithms search similar products in its catalog to predict what the shopper likes and make product recommendations within the ecommerce site. Each customer interaction within the website trains the deep learning technologies in real time, according to the website. The site claims it does not need historical data to make recommendations, so that the algorithms also work even for first-time visitors.
As the customer clicks on the products they like, the AI extracts that behavioral data, and connects the customer’s preference with the kind of style they may be looking for.
This action enables the algorithms to recommend products similar to the customer’s initial selection until the shopper finds exactly what they is looking for, according to the company.
The 1-minute video below demonstrates how the application works once the shopper begins a product search on the website.
Our quick test of the website for the first time showed that if a shopper adds a sundress to the cart, the recommendation engine recommended jackets and pants. Immediately, a second test for running shoes turned up other models and colors from the same brand.
A third, more specific test for trail running shoes also turned up more specific recommendations that mixed road running and trail running shoes. When an item is added to the cart, other products that the shopper might like are also displayed at the bottom.
The engine can be integrated into a variety of channels such as eCommerce sites, social media and mobile applications in different industries. In retail eCommerce, the engine can make movie recommendations and is able to predict what a person wants to watch. In travel sites, the algorithms can identify the vacation package a person is most likely to choose.
Luxottica Brands, which distributes Sunglass Hut products, integrated Sentient Aware on their website branded as “My Frame Finder.”
According to the case study, the eCommerce which site sells eyewear to “thousands of shoppers throughout the United States every day,” integrated the Sentient Aware engine for nine months on its site.
Over that period, the client reported a 21-percent higher conversion rate and an 8 percent increase in the average amount per purchase among shoppers who used the recommendation engine compared with the traditional search and filtering experience.
Sentient’s Co-founder and CEO Babak Hodjat was senior director of engineering at Sybase iAnywhere. He was also a co-founder, CTO and board member of Dejima Inc., acquired by Sybase in April 2004. At Dejima, he was the primary inventor of the technology applied to intelligent interfaces for mobile and enterprise computing, the technology behind Apple’s Siri.
Hodiat holds a PhD in Machine Intelligence from Kyushu University, in Fukuoka, Japan.
Moving forward, Sentient Aware says they plan to add more sensory receptors to its recommendation engine, enabling it to recognize more content types such as video, voice, music and text.
Dynamic Websites
Dynamically changing websites are driven by script to generate the changing content based on a site visitor’s preferences. This allows developers to delegate repeatable tasks such as rendering or tagging photos to AI technologies such as machine learning or image recognition. The software offered by AI companies enable less technical-minded individuals or smaller companies to develop websites on their own.
Bookmark
One company that claims to apply machine learning to web design is Bookmark. According to the company site, Bookmark’s AI Design Assistant (AIDA) builds client sites by predicting what sections, elements, images and design style the should be featured. These decisions are powered by information that is relevant to the business owner’s specific industry.
AiDA is trained to scan the web to find and learn more about the client by searching its name, location, and type of business. AIDA’s algorithms also gather the information from social channels to determine what elements, colors and layout would be relevant to the client’s eCommerce website while taking into account customer behavior such as what they’re saving, editing, and publishing on social media.
The 2-minute video below shows how the dynamic web pages are created using AIDA. the application will ask what kind of website the designer wants to create, the name of business or website and other details.
Similarly to WordPress or other website builders, a user begins by selecting the type of eCommerce site they want to build from a drop-down menu. These types of sites include auto, restaurant, and retail. The user is then asked to fill in company information, such as the name, operating hours and address.
After filling in this information, AIDA can search the web and ask the user immediately if its business is associated with found social accounts. If the business is, AIDA can pull information, such as brand colors from these channels, according to the company. A user can also upload a logo and check a box if they would like to host an online store on the site.
After the user fills in the setup information, AIDA uses text, colors and brand information it finds from the company online to generate a number of branded website themes, with similar color and styles. The theme is also customizable, allowing the user to swap out photos, text and product information.
While the company does not list its clients, it says it offers a two-tier program of this product.
The first tier is for affiliates who refer clients directly to Bookmark. Affiliates earn $120 for each conversion, and Bookmark itself will manage the client and design the website.
The other tier is for design agencies who want to create fully functional websites for their own clients. For agencies, AIDA claims to make designs available for 750 business types, which can be used for unlimited sites and pages. These pages claim to adapt to web and mobile platforms.
Pricing for small design agencies and entrepreneurs start at $11 monthly, inclusive of unlimited bandwidth and storage and domain. Other pricing details can be found here.
AIDA claims its software can build a website in two minutes. They also claim it can recognize and extract colors from a company’s logo and apply those colors to the design elements.
As the shopper keeps using the website, its algorithms gather more context about them, and improve at making relevant recommendations, according to the company.
For instance, a design agency could use AIDA to create five to 10 different website design studies that will be presented to clients. Once the client selects the site design and the client provides specific feedback, the designer could apply the final touches to further customize the site. AiDA clients have not made any case studies available to date.
Atanas Krangov is the CTO of Bookmark. Before joining Bookmark, he led the team that designed MD.com, a site that helps patients find a doctor in the United States. He has a Master’s degree in software engineering from the Faculty of Computer Science and Engineering in Skopje.
LiftIgniter
LiftIgniter offers a recommendation system that claims to be based on a machine-learning algorithms called “true parallel multivariate algorithms infrastructure.” The company explains that the application is integrated into eCommerce sites online on the web or on mobile.
Once integrated, the application’s algorithm works by allowing the program to learn what each shopper is looking for, and recognize additional items that they might be interested in through their onsite behavior.
The application then sorts through the eCommerce site’s content such as photos, videos and articles, and displays product recommendations in 150 milliseconds or less. It also displays related products each time the user visits the site.
In the short video below, the company claims that when LiftIgniter’s application is integrated into an ecommerce site, its machine learning capability predicts individual intent to match the content to each user in real time, and how each website or app reacts differently to each user impression. [[]]
The company also claims that the AI can recognize a site visitor’s browsing speed and recommends content immediately, while it predicts demographics or other segments that could be associated with the site visitor.
Listia wanted to keep up with leading eCommerce sites which were using machine learning personalization to drive traffic and engagement. They turned to LiftIgniter to train recommendation algorithms using specific product attributes in the Listia’s eCommerce site, along with metadata and user behaviors.
Because the products were purchased very quickly, real-time speed of the system was critical.
With the integration LiftIgniter on Listia, the application recommended ‘Things that You Might Like’ to shoppers throughout the online site and on both Android and iOS mobile apps. Every time a user views an item, they are shown personalized recommendations either on the side or below the product that the customer is viewing.
The application is also able to track how often people clicked on related items and other interactions such as bidding on and purchasing items (click-through rates). The company did not provide a timelines and previous numbers for comparison, but the study reports that click-through rates improved by 200%, bids by 125%, and purchases by 50%. No other
Among the company’s other clients are the Vevo, Telegraph and NTT Docomo.
Indraneel Mukherjee, Founder and CTO at LiftIgniter, was a research scientist at Google and had internships at Morgan Stanley and Microsoft. He earned his PhD in Computer Science from Princeton University.
Concluding Thoughts
The AI technology noted above claims to drill deeper into each customer’s unique preferences, through features that track online behavior, analyze demographics and note product preferences. Many of these companies claim that their algorithms are able to learn more about regular visitors and become better at predicting and recommending what regular visitors are likely to buy.
To ensure that the unique customer’s context is better captured, many of the applications claim to utilize not just one kind of AI subset, but several at the same time. This includes computer vision, image recognition, machine learning and deep learning among others.
In the past, marketers would group or segment potential customers who are likely to respond similarly to a marketing tactic, based on comparable attributes. Today, the process is seemingly reversed with the help of AI, as marketers are able to collect and analyze data to personalize and contextualize the messages and customer experiences.
With many of the above applications, messaging claims to be custom-tailored to one person’s needs. The companies claim that this strong communication with a customer could eventually prompt regular purchases.
While AI technology has enabled the automation and personalization of the repetitive part of the marketing process such as sending emails and conducting searches, humans continue to perform what is believed to be intelligent thought such as problem solving, setting strategies for the campaign, defining the messaging and the voice, and even deciding on the final dynamic website designs and putting the unique or final touches to the pages.
Despite the successes some marketers have experienced using AI to personalize marketing, research suggests that many continue to face challenges in connecting the data sources, the very foundation of effective AI. In the above-noted Researchscape International survey of marketing professionals, 55 percent said they don’t have sufficient data and insights to drive personalization. In the survey, many marketers reported that data is stored in siloed or disparate systems such as the web, mobile and email with no means to connect these channels.
The survey also reports that 88% of marketers believe customers will expect more personalized marketing to save time and more quickly help them find what they need.
Header image credit: MyMobileLyfe