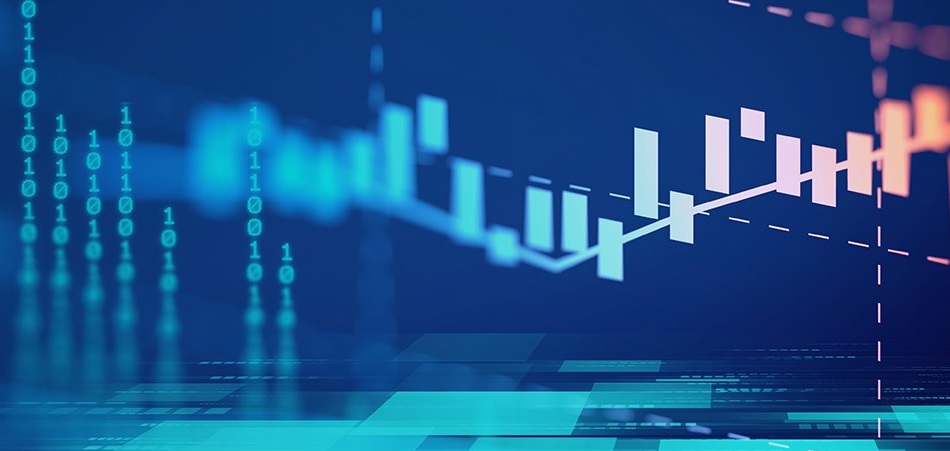
It can be difficult for financial institutions to keep up with the rapid changes in the digital marketing and advertising landscape. There are numerous factors which are susceptible to change, and they all have an effect on how useful certain marketing strategies are. As the internet and advertising evolve, some companies may find it important to consider an automated solution to driving efficiency in marketing.
In this article, we cover two examples of AI-powered marketing applications. Each vendor that offers these applications provides evidence of success with their digital platform, and so we can infer that at least some financial institutions benefited from the adoption of these systems. The solutions we cover are:
- IBM Cognos Analytics: Formerly IBM Watson Analytics, this is IBM’s AI business intelligence platform. It is purportedly capable of helping financial institutions analyze advertising data to find the most successful marketing campaigns.
- AI-powered Analytics for Card-Linked Marketing: Cardlytics’ AI platform that can utilize transactional data from credit and debit cards to drive efficiency in targeted digital ads.
The first AI marketing application we cover is IBM’s Cognos Analytics platform.
IBM Cognos Analytics (Formerly Watson Analytics)
Many AI applications for marketing agencies and internal marketing teams are advertised as business intelligence solutions. They focus on the ability to analyze large amounts of data at once and share the insights from that data across multiple user endpoints.
Additionally, offerings from larger companies may include the ability to take in data of various types and from various sources. A financial institution would simply need to run this type of analytics application using their advertising data, and they would be able to gain an understanding of which of their advertisements work the best.
Another way this type of application could drive efficiency is in advertising expense reporting. Analysts require reports using the most recent data in order to gain an understanding of how well marketing campaigns are engaging customers.
If these reports take a considerable amount of time to produce, business leaders will be waiting even longer for their insights from the analysts. Automating this process can help financial institutions make the most important decisions faster.
We spoke to Sasha Caskey, CTO of Kasisto, about this on our podcast, AI in Banking. When asked about where he believes AI is actually driving value in banking and financial tasks, Caskey said:
One of the places where AI has been the most impactful … broadly and specifically around banking is really in taking over some of those mundane repetitive tasks that people have to do. … it’s able to shave off a layer of work that people have to do by essentially boiling down the problem to a set of numbers that people can look at make an informed decision about.
This approach of freeing up human employees to make the kind of informed decisions marketing leaders need to daily could be an early driver of efficiency when adopting an AI solution.
IBM is a vendor that offers this type of analytics application to clients in the financial industry and marketing agencies that work with banking and financial clients. Its software, Cognos Analytics, is an AI platform capable of data mining and predictive modeling. This means it is likely built with predictive analytics and a machine learning algorithm trained on advertising and engagement data.
Below is a video that explains some capabilities available on the Cognos Analytics platform. The capabilities covered in this video include:
- Adding attributes to names of customers for more granular analysis
- Creating dashboards for readable visualizations of important insights
- Creating expense reports for review by management and human resources teams
IBM lists a case study on their website in which they claim to have helped IMM Marketing Agency utilize their clients’ numerous datasets in order to more accurately target their digital advertisements.
IMM purportedly buys thousands of internet advertisements every day, and so making sure they are properly targeted to the right demographics is a high priority.
Digital marketing teams may find difficulty in continually optimizing targeted advertisements because online customer behavior can be hard to keep track of and predict. This requires near-constant monitoring of responses to the advertisements and adapting their marketing styles for the next iteration.
IBM’s analytics solutions purportedly helped accomplish this by analyzing large amounts of data at a time and delivering records of conversion rates, impressions, and click-through rates for each digital advertisement.
This means analysts were able to gain a detailed understanding of the engagement rates of previous advertisements quickly before running their own more granular experiments.
According to the case study, IMM was able to start savings tens of thousands of dollars simply by how fast they could now determine if a given advertisement approach was succeeding. IMM also claims they can use IBM’s analytics platform to bring up incremental sales and add more value in the ads their clients spend money on.
Additionally, marketing reports that purportedly once took 12 hours a day became available within 45 minutes. This would allow IMM’s analysts to focus more on finding the best next step from these insights rather than having to produce the reports themselves.
AI-powered Analytics for Card-Linked Marketing
Credit card companies and other financial institutions can use spending behavior and other customer information for data science and machine learning projects for marketing and promotional offers.
One way they could accomplish this is to use historical credit and debit card data to better individualize targeted deals from marketing companies. Customers would then receive targeted ads for products they are more likely to purchase. One could broadly describe this technique as “card-linked marketing.”
Cardlytics is a vendor that sells this type of AI application to credit card companies and financial institutions. Their software uses customer segments called “purchasegraphics” to match customers with deals they are more likely to take. Purchasegraphics are customer segments, or “demographics,” based on spending data including when the customers spend money and on which products.
The company also claims their AI solutions are built to prioritize the privacy of customers’ sensitive information while maintaining accurate targeted ads. There are many factors that can affect which deals are matched with which customer or their associated purchase graphic. These include age, gender, time of year, geolocational data, the types of products purchased, and if these products follow previous purchase behavior.
These demographics are later analyzed for their shopping and financial habits which help the software create new segments of customers that are similar based on what they spend money on.
All of these factors are important to enabling card-linked marketing as targeted ads can perturb some customers especially while they are handling their funds.
If customers feel too bombarded with ads that don’t relate to their needs or desires, they may feel less comfortable with using the app or other platform the client financial institution is using. For example, this could contribute to banking customers losing loyalty to their current bank and opening accounts elsewhere.
Customer segmentation based on spending behavior can allow banks and credit card companies to focus on the most important criteria within customer data that point to effective targeted ads. This method enables more granular customer matching which can also utilize spending data from credit and debit card swipes.
Each transaction creates a data trail about where the customer spent money, at which merchant, and possibly the names of the products purchased. This may allow a machine learning algorithm to better match customers with offers based on their most recent spending behavior.
A financial institution will not need to build any hard rules for their software to recognize differences in individuals when analyzing demographic signifiers. This is because a machine learning model for these applications would not necessarily need prior training to discern that some items are family products such as baby food and diapers.
This is the same for products marketed toward a specific gender such as soaps and razors. The software can come to these conclusions through the implications of their spending habits. However, many people shop for others that they live with. This is why a machine learning model does not make hard determinations about gender, and instead moves individual customers toward an implicit gender.
It is apparent that Carldytics aims to never share personal information from a banking customer with the retailers from which they are getting their marketing information from. Cardlytics’ platform sends performance reports from the client bank’s database to the company’s front-facing marketing team. This team then communicates that information with the retailers.
The retailers most likely use this data to create new marketing strategies, which they can submit back to Cardlytics and be matched to the best customer segment.
Cardlytics lists a press release on their website which refers to a case study Celent conducted regarding Bank of America’s “BankAmeriDeals” marketing program. The case study purportedly states that Bank of America became a user of the Cardlytics platform which uses spending data from about 70% of American households.
The study also indicated how retailers can reliably dedicate a portion of their marketing budgets on card-linked marketing initiatives and see significant ROI. This could mean that companies looking to target their digitally literate customers in a profitable way may want to look further into use cases for these types of AI applications.
Header Image Credit: Altran