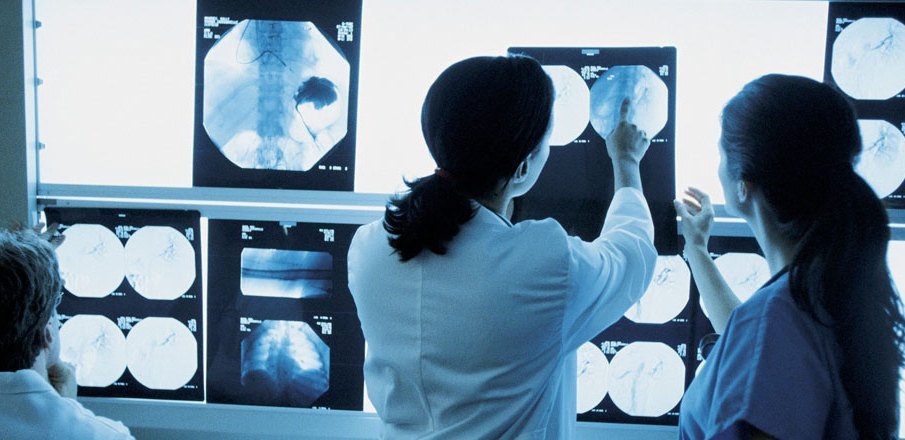
It should come as no surprise that AI has found its way into radiology in a similar fashion to most other medical fields. Many AI vendors selling into the radiology field are just beginning to gain regulatory approval. We researched the use of AI in radiology to better understand where AI comes into play in the industry and to answer the following questions:
- What types of AI applications are currently in use in radiology?
- What tangible results has AI driven in radiology?
This report covers vendors offering software across two applications:
- Diagnostics
- Medical Imaging
This report intends to provide business leaders in the medical space with an idea of what they can currently expect from AI in their industry. We hope that this report allows business leaders in healthcare to garner insights they can confidently relay to their executive teams so they can make informed decisions when thinking about AI adoption. At the very least, this report intends to act as a method of reducing the time business leaders in healthcare spend researching AI companies with whom they may (or may not) be interested in working.
Diagnostics
It should be noted that none of the companies listed in this report claim to offer diagnostic tools, but their software could help radiologists find abnormalities in patient scan images that could lead to a diagnosis when interpreted by a medical professional.
Aidence
Aidence offers a software called Veye Chest, which they claim can help radiologists detect, track, and report on pulmonary nodules using machine vision. According to the company, the machine was initially trained on 45,000 labeled scans and independently validated by radiologists.
Aidence claims Veye Chest can be integrated with a hospital’s existing reading and reporting software. According to Aidence, the pre-trained algorithm detects the presences or warning signs of pulmonary nodules within patient CT scans. This could help radiologists determine if the nodules are a threat or not.
Then, Veye Chest produces text and annotated picture indicators on the computer screen. A radiologist can then use the text and annotations to more accurately diagnose their patient’s condition and track the size of their patient’s pulmonary nodules during the course of their treatment.
Aidence does not provide a video showing how its software works. However, below is an image of what the software might display on a computer screen:
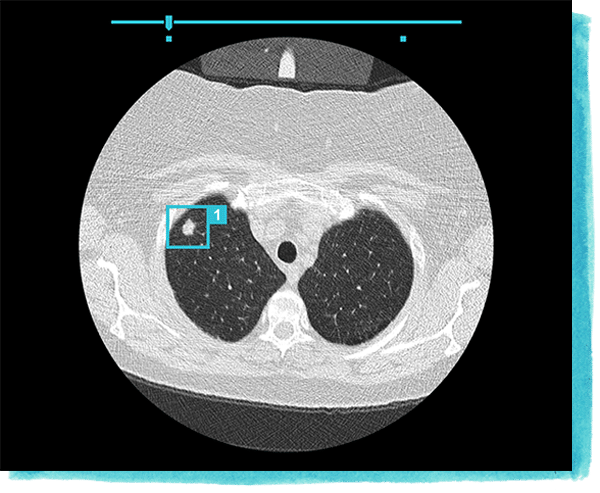
Veye Chest has received the CE marking, allowing its use in hospitals across the European Union. The CE marking is a certification mark that indicates conformity with the medical device standards of the European Economic Area. Aidence does not make available any case studies reporting success with their software, but that it received a CE marking might suggest the claims it makes on its website could be valid.
Aidence lists several hospitals in the Netherlands as clients, including Tergooi and Albert Schweitzer hospitals. Aidence also has a development agreement with the British SBRI Healthcare organization.
Mark-Jane Harte is co-founder and CEO of Aidence. He holds an MS in Computer Science from Eindhoven Institute of Technology. Previously, Harte served as co-founder and CTO at a few smaller Dutch marketing and tech companies.
Aidoc
Aidoc offers a software which they claim can help radiologists and their hospitals prioritize patients for care, detect acute abnormalities in patients’ bodies, and increase the speed of that detection using machine vision.
Radiologists can input a patient’s head, chest, spine or abdomen CT scans into the software and the machine learning model behind it identifies and displays all fractures, nodules, and hemorrhages in the scans. We can infer that Aidoc’s software searches its database for images similar to that of the patient’s scans in order to determine what could possibly be abnormalities in comparison to scans labeled “normal” in the software’s database.
Then, Aidoc compares the patient’s scans with those of other patients at the hospital that are logged in its system and in need of care. It uses that comparison to reprioritize the patient in terms of the urgency with which they should be treated compared to other patients at the hospital. This reprioritization is displayed on a computer screen for hospital staff to view.
Aidoc does not provide a demonstration video showing how its software works; however, below is an image of what the software might display to radiologists on their computer screens:
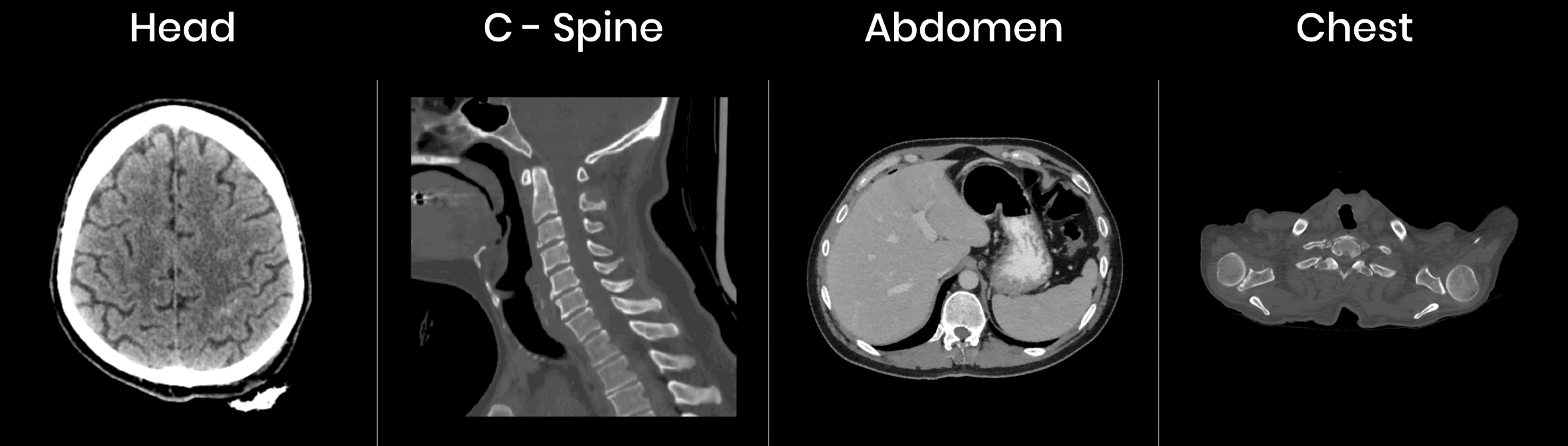
Aidoc’s software was cleared by the FDA and the Israeli Ministry of Health. Aidoc conducted a clinical study comparing a workflow aided by Aidoc’s software and a First in, First Out (FIFO) workflow on the average time it took for a patient to be scanned and receive a report containing their diagnosis. The workflows were benchmarked using standards outlined by The Joint Committee on Turnaround Times. According to the study, its software reduced head and neck report turnaround time by 60.3%.
It should be noted, however, that the clinical study was to our knowledge not published in any academic journal. In addition, there are only three names attached to the study: Aidoc’s CEO, Chief Medical Officer, and Head of Regulatory and Clinical Affairs. In other words, the study does not seem to have been independently conducted, nor did it involve anyone outside of the company. We believe this warrants skepticism, but Aidoc’s FDA clearance does lend its software credibility.
Radiologists at Cedars-Sinal Medical Center and the University Hospital of Basel currently use Aidoc’s software, according to the company. Elad Walach is the CEO and co-founder of Aidoc. He holds an MS in Computer Science with a focus on machine learning. Previously, Walach served as a research team leader in the Israeli air force, where he worked on computer vision and other machine learning projects. Michael Braginsky is the CTO and co-founder of Aidoc. He was head of R&D at the Israeli Ministry of Defense and earned an MS in Computer Science and Medical Image Processing from the Hebrew University.
Kheiron Medical
Kheiron Medical is a UK-based company that offers a software which it claims uses machine vision to help radiologists conduct mammograms and reduce the number of false positives and false negatives from those mammograms.
Kheiron Medical claims radiologists can input a patient’s mammogram scans into their software. We can infer that the company’s software then searches its database for images similar to that of the patient’s scans in order to determine what could possibly be abnormalities in comparison to scans labeled “normal” in the software’s database. The system then identifies possible abnormalities on the mammogram scans by displaying colored circles on the scans to which radiologists can focus their attention.
We could not find a video that demonstrated how Kheiron Medical’s software works.
Kheiron Medical received a CE marking in 2018.
Tobias Rajken is the CTO and co-founder of Kheiron Medical. He holds an MSc in Computational Science and Machine Learning from the University of London. Previously, Rajken served as a cohort member at Entrepreneur First.
Medical Imaging
Arterys
Arterys offers a software called ArterysAI. The machine learning model behind the software was reportedly trained to focus on detecting abnormalities in the heart, although the company claims its software is able to detect abnormalities in the lungs and liver to some degree. Arterys claims their software can help radiologists to see and garner a better understanding of a patient’s heart without the need for invasive procedures and radiation using their cloud-based machine vision software.
Artersy claims hospitals can dramatically reduce the time radiologists spend scanning patients. For example, Artersy’s CardioAI, a software under the ArterysAI umbrella, uses what the company calls 4D Flow. Aidoc claims 4D Flow is installed on a standard MRI. 4D Flow reportedly allows radiologists to see a three-dimensional full scan of a patient’s heart that they can manipulate on a computer screen after an MRI scans a patient. The company claims these scans allow radiologists to gain a more genuine understanding of the patient’s heart without requiring time-consuming, invasive surgery.
Then, 4D Flow takes the images, heartbeat patterns, and blood flow data gathered from the patient and compares that data to pre-labeled data of regularly functioning hearts and dysfunctioning hearts on which the model behind 4D Flow is trained. Anomalies within the patient’s heart are identified on a dashboard.
Below is a short 3-minute video demonstrating 4D Flow’s visual scan display:
ArterysAI was cleared by the FDA, and it claims its software has been validated in seven different peer-reviewed medical journals, including the Journal of Cardiovascular Magnetic Resonance. We could not find available case studies reporting success with the company’s software.
Arterys was one of the original companies to collaborate on the Siemens Healthineers Digital Ecosystem alongside Dell, SecondOpinion.com, and others in the field.
That said, Arterys does not list any major hospitals as clients on their website. The company has raised $43.7 million and is backed by Emergent Medical Partners and 14 other investors.
John Axerio-Cilies is co-founder and CTO at Arterys. He holds a PhD in Flow Physics and Computational Engineering from Stanford University. The rest of the C-team at Arterys hold PhDs from Stanford University with the exception of their CEO, Fabien Becker, who earned his PhD in Physics from the University of Cambridge. Such a roster bodes well for Arterys and lends credibility to their software.
Takeaways for Business Leaders in Healthcare
AI in radiology is just getting its barrings in the market. Only in the last few years have AI radiology vendors been able to put products to market. For many AI companies that are not in an FDA regulated field, product research and development can run alongside the marketing and sales efforts of their company to some degree, allowing a small amount of revenue to trickle in from early adopters while the finishing touches or more advanced features are rolled out and made public.
However, we suspect that the software discussed in this report are offering software that is of reasonable legitimacy considering most of them were approved by the FDA or similar government bodies. Much like how venture capital firms in Silicon Valley scrutinize companies that seek their funding, including the AI models at the core of those companies, some government bodies exhibit a similar deal of rigor in determining which companies win approval. Companies that do win approval are therefore likely to offer legitimate software.
That said, business leaders should not take government body approval as the end-all-be-all when it comes to determining from which AI vendors to buy. We recommend business leaders read our article on Separating AI Hype from Reality to give them a better idea of how to determine if an AI vendor is legitimately offering an AI solution or not.
Arterys appears to have the most traction and is the most developed of all the companies highlighted in this report. It also holds multiple FDA approvals allowing its software to aid radiologists in diagnosing conditions in various parts of the body. Additionally, Arterys claims to be mentioned in multiple peer-reviewed medical journals. Arterys has also secured $43.7 million dollars in funding, which is a considerable amount more than the other companies discussed in this report.
Patients might expect to be administered faster quality care with less diagnostic inaccuracies in the next 2 to 5 years. Improved scanning procedures could save hospitals and patients on costs associated with conducting unnecessary biopsies.
Businesses should not expect an all-in-one solution for radiology when it comes to AI software, in contrast to the way an MRI or X-Ray machine might be able to provide imaging for numerous parts of the body. The machine learning models behind AI software for radiology must be trained and taught on each specific part of the body it intends to scan and “understand,” and it must be fed data specific to the abnormalities it intends to detect. At least in the near future, this means hospitals will may need to purchase one AI software per part of the body they intend for it to scan.
One element of AI in radiology that was surprising to see is the integration of the AI software we reported seems relatively easy, compared to other markets. This may be as a result of most software working with MRIs and MRI’s themselves having limited outputs. As a result, it may be easier to build AI around those known outputs compared to other medical fields in which there are many different devices used to diagnose one problem.
Aidence, Arderys, and Aidoc referenced their software would work with PACS systems. Arderys and Aidoc mentioned their software would work with RIS systems as well.
In general, radiology appears to have the desirable trust symbols of an industry genuinely working with AI. Each company had PhD-level talent on their C-team, further indicating legitimacy with regards to their AI models.
Header Image Credit: Penn Medicine