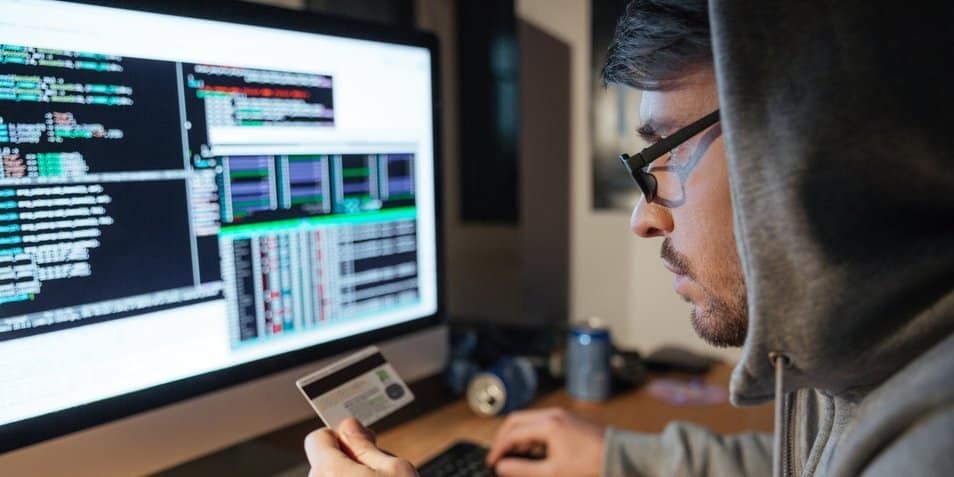
In 2017, Equifax’s systems were compromised by hackers, and the data of over 143 million Americans was exposed. Other incidents, such as the WannaCry and Petya ransomware scams, have highlighted the vulnerabilities in financial cybersecurity globally. According to the Global Banking and Finance Review, such cyber attacks have cost nearly USD 360 billion per year in losses for each of the last three years.
We researched the use of AI for cybersecurity in finance to better understand where AI comes into play in the industry and to answer the following questions:
- What types of AI applications are currently in use for cybersecurity in finance?
- What tangible results has AI driven in financial cybersecurity?
- Are there any common trends among these innovation efforts? How could these trends affect the future of banking and finance?
This report covers vendors offering solutions in the following application areas:
- Money Laundering Security and Fraud Detection
- Aggregating Cybersecurity Data
- Monitoring and Preventing Cyber Threats
This article intends to provide business leaders in the finance space with an idea of what they can currently expect from AI in their industry. We hope that this report allows business leaders in finance to garner insights they can confidently relay to their executive teams so they can make informed decisions when thinking about AI adoption. At the very least, this report intends to act as a method of reducing the time business leaders in finance spend researching AI-powered financial cybersecurity vendors companies with whom they may (or may not) be interested in working.
For more information on how AI can facilitate cybersecurity and other aspects of banking and finance, download the Executive Brief for our AI in Banking Vendor Scorecard and Capability Map report.
Money Laundering Security and Fraud Detection
Feedzai
Feedzai offers software solutions which they claim can help banks, acquirers, and merchants with detecting and preventing money laundering and fraud.
Feedzai claims that their data science software, OpenML Engine, can help data scientists employed by banks to build their own custom fraud detection models using the fraud-specific models already provided in the software. Below is an image demonstrating how it works:
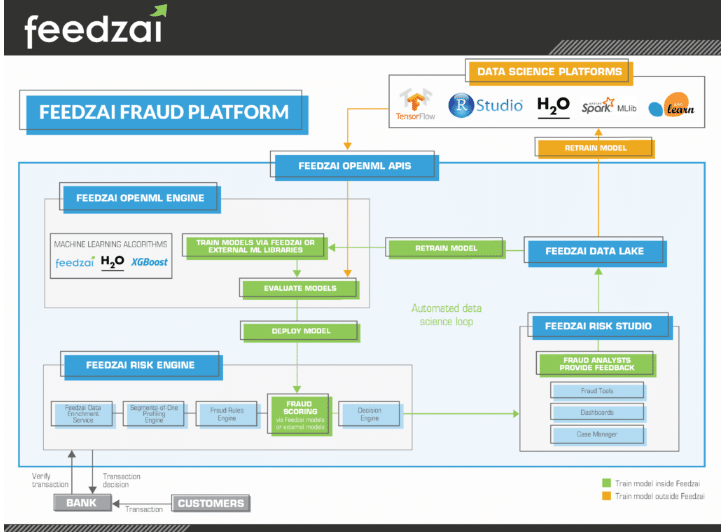
The company claims their software can be integrated with a bank’s existing systems using data stored internally in the bank’s data centers. Feedzai’s system can potentially analyze these data streams and gain fraud insights such as identifying a fraudulent transaction from a customer by creating granular risk profiles for customers in the form of a fraud score for them.
Some experts consider the adoption of AI cybersecurity applications to be somewhat difficult for IT and cybersecurity departments to handle given all the changes it implies We spoke to Adam Hunt, CTO of Risk IQ about this on our podcast, AI in Banking. When asked about how IT and cybersecurity departments in banks and financial institutions will have to change, Hunt said,
“As [cyber] threats evolve, it’s very easy to break these rules and evade detection. All they have to do is change a few characters and … their threat is now a threat again. What AI allows us to do in the security realm is allows us to generalize a lot broader. The issue that we’re facing where a small renovation in the threat can avoid detection with these rules is no longer the case. … It’s more difficult to deploy, you have to have a different set of skills, so it’s not as easily adopted as some … traditional techniques.”
It is important to note that having dedicated AI talent in a business could help the cybersecurity team become more familiar with the AI applications they would be working to help leverage during and after adoption.
Feedzai’s machine learning algorithms then process new events and transactions to continuously update the fraud scores gained from the risk engine, which are presented to the bank’s employees through dashboards.
Feedzai’s platform is usually integrated within a bank or merchant’s systems and can alert human fraud and risk analysts with only the cases that are truly considered high-risk (based on predefined factors), thereby speeding up fraud detection processes and reducing false positives.
From 22:08 to 25:10 in the video below from StanfordEuropreneurs, Nuno Sebastiao, CEO and co-founder of Feedzai, explains how Feezai’s software could help banks with fraud detection using machine learning:
Case Study with an Established Bank
Feedzai claims to have worked with a top 10 US retail bank in a fraud detection project. The bank decided to offer its core checking account via an online sign-up process and found that their existing fraud and risk screening process was rejecting more than half the online applicants, causing them to lose business to competitors.
The bank needed a risk-assessment system that could sift through new account applications and only accept customers with a low likelihood of committing fraud. The system needed to ensure that only the truly risky applications could go into manual review and that risk factors were clear for easy decision-making in order to reduce the time spent by human security experts in reviewing each case.
Feedzai’s platform was deployed at the core of the bank’s existing enterprise systems using the bank’s own data centers. This enabled the Feedzai platform to be the central decision engine for the bank’s online customer onboarding process and verify identity, check eligibility, and assess fraud risk in real time. In cases where the software did not have enough data to make a decision, Feedzai claims the software could automatically trigger customer-specific follow-up questions, predefined by the bank’s onboarding team, during the online application process itself.
Feedzai claims that the bank saw the following improvements after integration:
- A 70% increase in new application approvals after the integration,
- Reduced manual verification.
- Zero increase in fraud losses despite the increase in approved applicants. Details on how long the integration took or tangible results on what other measures were taken by the bank to improve customer onboarding at the same time were unclear at the time of writing.
Feedzai lists clients such as Capital One and Citi on their website. Feedzai’s Chief Science Officer Pedro Bizzaro holds a PhD in computer science from the University of Wisconsin-Madison, and he is a visiting professor at Carnegie Mellon University. Pedro is a member of the Forbes Technology Council, European AI Alliance, and Algorithmic Bias Working Group at IEEE.
Aggregating Cybersecurity Data
DefenseStorm
DefenseStorm was founded in Alpharetta, Georgia in 2014 and has 32 employees. The company claims their software can help banks and financial institutions automate cybersecurity and cybercompliance using machine learning.
The company’s software also offers tools like PatternScout and Threat Match, which can potentially help banks with increasing visibility in their networks and monitoring internal systems in real-time for anomalies in the network.
The software tools can reportedly help banks detect and identify cybersecurity threats in their networks, enabling them to save on long-term security costs and avoid data leaks. Using machine learning-based pattern recognition on historical network data, the company claims their platform can support company-wide security and operational activities.
DefenseStorm claims that their SaaS solutions can help IT security personnel at banks gain access to security event-related data in one place through a single dashboard. IT personnel can log into the dashboard and rapidly respond to security threats identified by the software.
Below is a 2-minute video giving a demonstration of the Threat Match tool from DefenseStorm:
DefenseStorm has collaborated with multiple banks, such as the Live Oak Bank and the Washington Trust Bank in cybersecurity projects.
Live Oak Bank Case Study
In a case study with Live Oak bank, DefenseStorm claims the bank had many data centers around the US using multiple technologies and applications to support their small business lending and deposit platforms.
The bank was facing a challenge in aggregating all logs and event data (routers, firewalls, and intrusion prevention systems) into one dashboard where their IT security personnel could then easily search and manage incidents. In short, they needed to increase the visibility of security threats and reduce their reaction time to high risk, high-threat activities, without large-scale increases in headcount.
DefenseStorm claims to have integrated their SaaS analytics solution to upgrade Live Oak Bank’s existing data management and analytics systems over the course of a couple of months. After the integration, DefenseStorm claims that Live Oak Bank was able to optimize big data searching and saw a 50–60% improvement in their incident discovery.
According to the case study, older tools used by the bank meant that the average time spent working on any given event by human security personnel was 15–60 minutes. After the integration of the DefenseStorm platform, employees were able to determine the scope of an event in one to five minutes to determine if the event needed to be escalated as a genuine incident.
DefenseStorm also lists Public Service Credit Union’s (PSCU), Peach State Bank, Lusitania Savings Bank and Citizen’s National Bank of Texas as clients.
We could not find any C-level executives on the team with an AI background. We caution readers to be wary of companies that claim to do AI without any C-level AI experts on their team.
Monitoring and Preventing Cyber Threats
Darktrace
Darktrace offers a cybersecurity software called the Enterprise Immune System, which the company claims uses machine learning to detect and respond to cyber threats across digital environments such as cloud, virtualized networks, IoT, and industrial control systems.
The Enterprise Immune System software can be integrated into the networks of financial institutions and offers tools like the Darktrace Threat Visualizer, which is a dashboard that can be used by IT security personnel to monitor cyber threats in real-time.
We could not find a demonstrative video showing how Darktrace works.
Ipreo Case Study
In a case study with financial services firm Ipreo, Darktrace claims to have developed a cyberdefense solution for the company. According to the case study, Ipreo’s security team understood that the traditional rules-based software and tools that it was using were no longer sufficient for meeting its security needs.
Ipreo decided to deploy Darktrace’s Enterprise Immune System technology, which the company claims uses machine learning and mathematics developed by specialists from the University of Cambridge. The technology can reportedly monitor the patterns in the data for users, devices and the network specific to Ipreo’s IT environment.
According to the case study, Ipreo also uses Darktrace’s Threat Visualizer interface, which gives the company a 3D graphical visualization of all activity occurring within its network across its global offices. Additionally, a weekly threat intelligence report is created by Darktrace’s security analyst team to allow Ipreo’s IT security engineers to better monitor vulnerabilities within its network.
Darktrace’s Other Work
We found evidence of Darktrace having worked with over 40 clients in cyberdefense projects across many industry sectors. Some of their clients include the Legislative Governing Body of the City of Las Vegas and the Birmingham Airport.
We were unable to find any C-level executives with AI experience on the company’s team although the company claims that their software uses machine learning algorithms developed by specialists from the University of Cambridge. We must state here that it was unclear what the relationship between the company and the University of Cambridge was.
PatternEx
PatternEx was founded in 2013 in San Jose, California. The company offers an artificial intelligence software it claims can identify malicious user intent and enable businesses to predict and prevent cyber attacks.
The company offers Virtual Analyst Platform, which was developed along with MIT’s Computer Science and Artificial Intelligence Laboratory (CSAIL). The company claims the Virtual Analyst System can analyze data (such as IP addresses, users, or sessions) from millions of users and detect suspicious activity, such as transactions from IP addresses with a history of fraud-related events using machine learning.
The patterns coaxed out by the platform are then presented to human information security analysts who confirm which events are actual attacks and which ones are false positives. The system then incorporates the feedback from the human analysts into its models for the next set of data to be analyzed.
Below is 2-minute video demonstrating the virtual analyst system and how it helps enterprises with cybersecurity using inputs from human analysts:
Patternex does not make available any case studies reporting success with their software. We could not find evidence of PatternEx selling to any prominent banks. As of writing this article, the company has raised $7.8 million in Series A funding. In other words, they seem to be just getting off the ground.
Kalyan Veeramachaneni is co-founder of PatternEx. He is a CSAIL Principal Research Scientist at MIT.
Takeaways for Business Leaders in Finance
As of right now, companies offering fraud detection or anti-money laundering solutions seem the most viable for businesses of various sizes.
Of all of the companies we covered, Feedzai seemed to have the most clout. The company has over 60 clients where they have worked in cybersecurity projects including banks like Capital One and Citi. We would expect more AI vendors to offer real-time fraud and threat detection for banking and financial institutions in the next three to five years.
In the near term, banks should not expect to easily be able to automate their information security processes using AI without embarking on a lengthy integration process requiring discussion with vendor support representatives and a large upfront cost. The largest enterprises may have the budget and staff to purse the technology, but based on our research, it is as of right now too nascent to be accessible to companies that would be able to afford other AI applications.
Banks must also be aware of the fact that any such AI endeavor would first involve data collection and organization, ensuring that security-relevant data, such as IP addresses, firewall data, and data from intrusion prevention systems, are collected in a similar format.
Header Image Credit: GoodCall