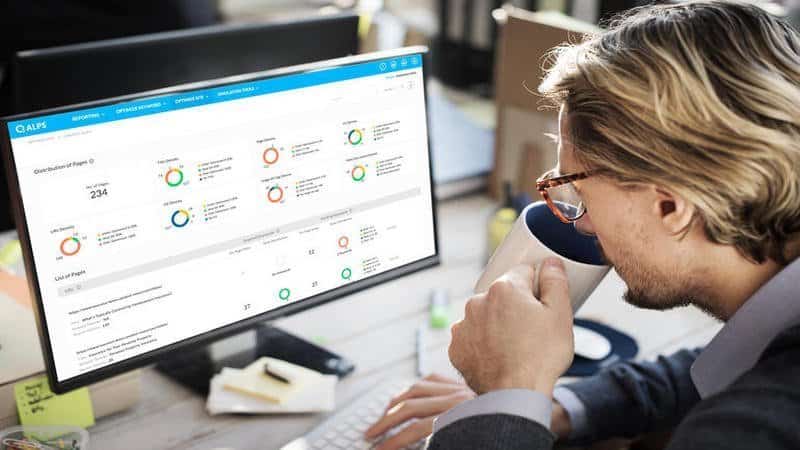
Episode Summary: Facebook and Google’s advertising complex is founded on machine learning, allowing people to self-serve their data needs across a broad audience. India-based InMobi is a company in the advertising technology space that delivers 10 billion ad requests daily.
Today, we speak with Avi Patchava, Vice-President of Data Sciences and Machine Learning at InMobi, which operates in China, Europe, India, and the US. Patchava explains how machine learning plays a role in appropriately matching advertising requests to the right audience at scale, whether on mobile, desktop or different devices and media.
Patchava paints a robust picture of what this technology will look like moving forward and how it will change the game for marketers and advertisers, especially with the emphasis on data and machine learning.
Subscribe to our AI in Industry Podcast with your favorite podcast service:
Guest: Avi Patchava – Vice-President, Data Sciences & Machine Learning, InMobi
Expertise: Machine Learning, Advanced Analytics
Brief Recognition: Avi Patchava leads strategy and design in InMobi’s Data Sciences, Machine Learning and Artificial Intelligence teams. Prior to this, he helped deliver 31 projects for clients of Advanced Analytics, Strategy, Operations and Organisation projects at McKinsey & Co. as their Analytics Expert, Senior Engagement manager or Associate/Analyst. He also has designed and managed more than 20 web and mobile applications, interfaces, and digital performance dashboards at McKinsey. His project experience spans automotive, financial services, manufacturing, IT services and technology, energy and materials, pharmaceuticals and health, public infrastructure and development, and consumer goods.
He earned his Master of Science degrees in Economics (Research) and Philosophy of Economics, Advanced Logic and Set Theory from the London School of Economics and Political Science. He also holds a Master’s Degree in Philosophy, Politic, and Economics from the University of Oxford.
Affiliations: Co-founder, Entrepreneurs’ Club London
(This interview was one of many one-to-one interviews with AI researchers and executives in India. Interested readers can explore our in-depth “AI in India” research article.)
Interview Highlights
(3:00) Can you give an idea in nontechnical terms of how machine learning plays a role in the bulk of operations of your business?
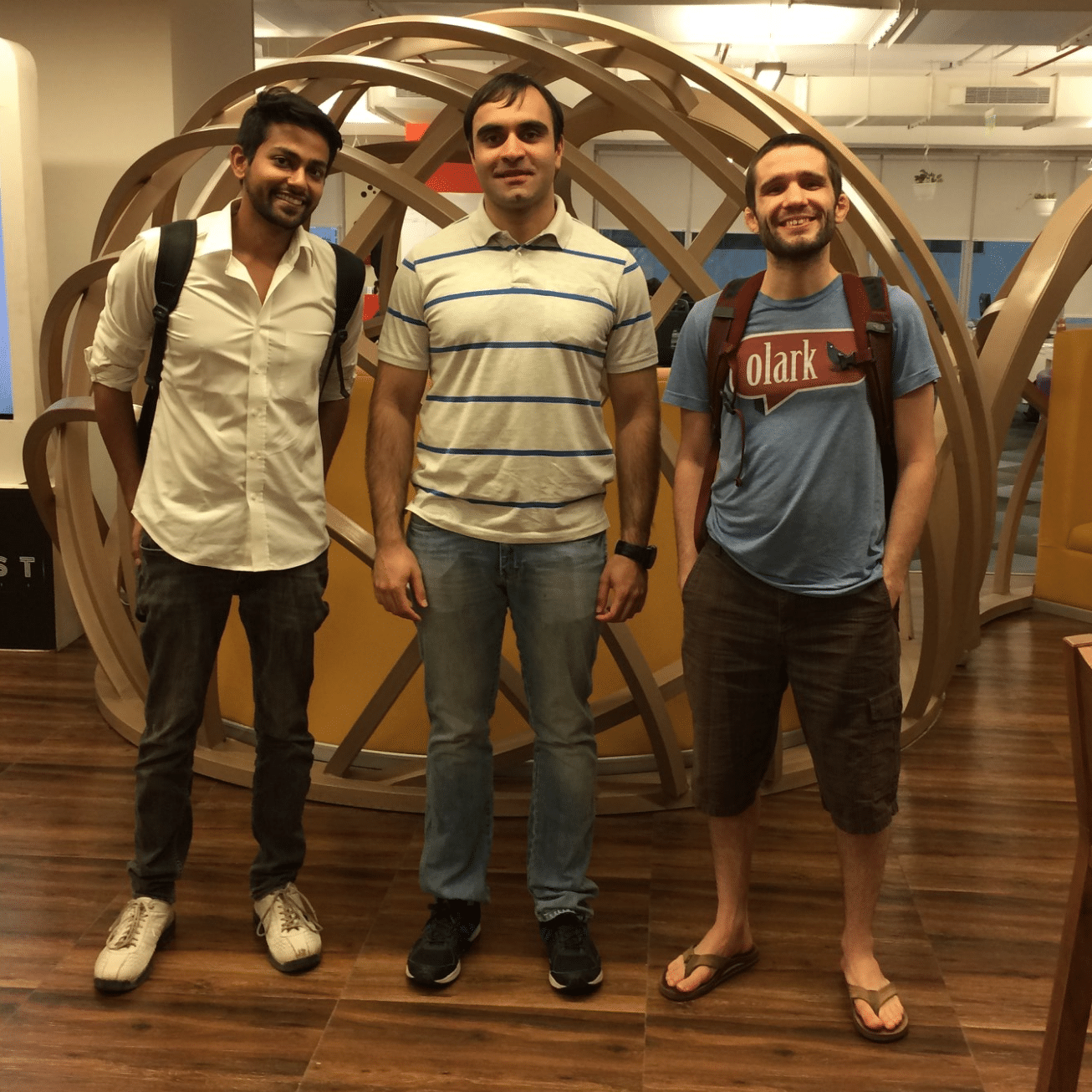
Avi Patchava: A big part of an advertising network is understanding where the opportunities lay for advertisers. To help advertisers and publishers alike achieve their objectives in a high-frequency marketplace, advertising technology (adtech) companies such as InMobi have to match the right advertiser with the right sites for both to achieve their KPIs (key performance indicators).
To do that, the company determines the value of a single ad request and decides which specific profile or audience will complete the CTA. Because ad requests often total more than 10 billion daily, quick decision making is imperative, a skill brought by a machine learning system. The ad is then run through a bidding mechanism, helping the advertiser to ultimately achieve their KPI.
This process happens potentially millions of times in every given advertiser campaign for every single request. The model acts on the advertiser’s behalf, valuing the potential of that request. A bid is run across that is either won or lost depending on the market mechanisms.
Adtech companies are committed to finding the best equation and bringing them together in an effective way to ensure that both advertiser and publisher are successful. On a scale of more than 10 billion ad requests, only automated algorithms with matching intelligence in your data can do that. Increasingly, the company brings in experts to share insights, ways of building algorithms, and how they are testing and learning.
(6:42) To paint a clearer picture of how the process goes, let’s take an example of an ad with a call to action (CTA) that targets a particular audience in a specific location. Your application can potentially predict how this ad will respond to a specific person, giving us a decent starting point for exposures. Then, the application would need to test the audience. Is that how the process goes?
AP: Matching the advertisement with the right audience entails two mechanisms, both of which involve machine learning. Firstly, the ad network must determine what it already knows about this profile and the likelihood that the audience will complete the CTA based on the historical data gathered from the billions of requests every day. Machine learning helps put a price on that specific ad.
For new categories or new types of applications that do not have the data, adtech companies use a machine learning system that allows them to learn, take feedback, and further invest or divest, all happening at a high level of data and speed for decisions to happen.
(8:48) Can you explain what targeting is?
AP: To target correctly, the company must be able to determine the audience and location of that audience. An audience can be defined by any variable. For instance, the advertiser wants to take its product to 25-year-old women living in suburban cities across the west.
You need to know how many people there are with those similar attributes. On the ad network, how many people like that do you have access to with that profile? Targeting helps you achieve that, building its different audience segments. Understand the scale of them, and you can maximize the potential of each person that meets this profile. This will give them the best chance of achieving their objective at scale.
(10:05) Do you sometimes need to find proxies or look-alikes when you target an audience? Is that part of building an audience quickly?
AP: Look-alike models are a big part of InMobi’s work because not all audiences are fully defined or variables may be incomplete. In those cases, the company needs to make inferences based on other types of data. You have two of three variables to define your audience. You will have to confidently infer the last variable based on other types of data. That variable could be a device or app that the audience may be using, or the location. When you know clearly what you are looking for and have good data to identify that audience, the look-alike helps you achieve scale.
(12:30) With regards to changes in the individual business functions of marketing and advertising. how are those coming together? What are those functions and what is bringing them together? And how does that bode for the future of your industry?
AP: Moving forward, marketing and advertising functions will be disrupted in a way that unifies them with the help of data.
In a world where that value chain—from marketing to the last mile of your advertising dollar—is integrated and the data and feedback is flowing well with the help of speed and scale of automation, you have a world where these two functions become one.
(15:10) So this is how the ad network world is affecting marketing. Instead of being the execution, it becomes part of the strategic function.
AP: Let’s take the example of a chief marketing officer (CMO) with ideas on how to drive user acquisition or reduce the user attrition. The CMO can quickly learn from the data related to that last mile of advertising spend and decide to adjust the strategy and spend. This helps the company achieve acquisitions at the lowest possible cost.
With data available at speed and scale, we are driving insights, testing, coming up with hypotheses and competitor analysis and insight. Ultimately, we can maximize intelligence and learning to help CMOs make decisions.
Subscribe to our AI in Industry Podcast with your favorite podcast service:
Header Image Credit: Zdnet.com