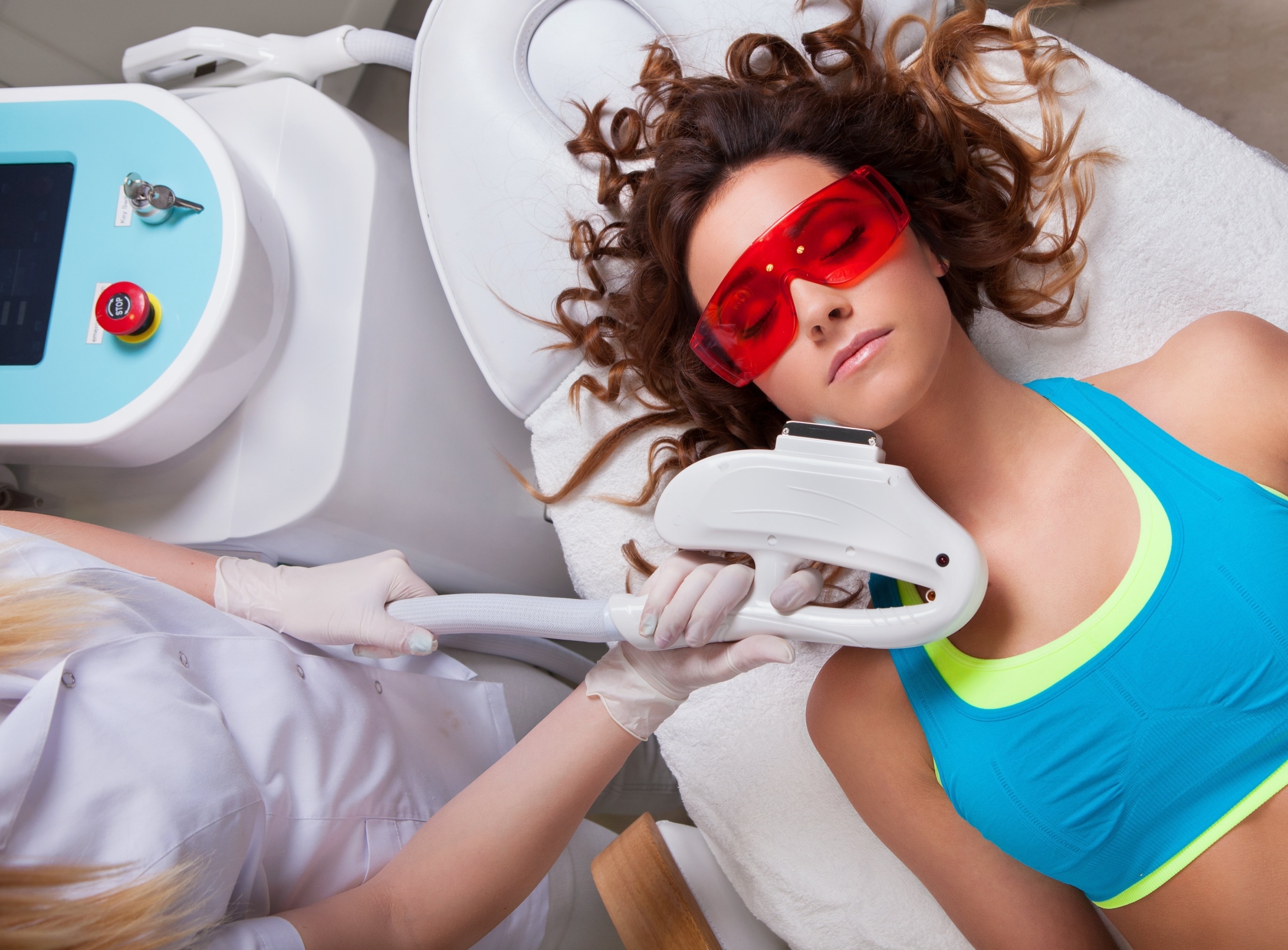
Dermatology is defined as a branch of medicine primarily focused on the evaluation and treatment of skin disorders, including hair and nails. Market research firm BCC Research projects that the global market for skin disease treatment technologies will reach $20.4 billion in 2020. Based on $17.1 billion in market revenue in 2015, this anticipated increase represents a five-year compound annual growth rate (CAGR) of 3.6 percent.
However, the field of dermatology is experiencing a workforce shortage at that same time that skin cancer rates are increasing. The annual economic burden of skin cancer treatment in the U.S. has been estimated at $8.1 billion. Skin cancer treatment options may include surgery, radiation therapy and/or topical medications.
In an effort to increase early-detection of skin diseases and to more effectively increase clinician capacity, researchers are exploring how AI could potential meet these challenges.
Some of the questions that need answering to better understand the role of artificial intelligence in the diagnosis and treatment of skin diseases:
- What types of AI applications are emerging to help treat skin diseases?
- How is the healthcare market responding to these AI applications?
Dermatology AI Applications Overview
The majority of AI use-cases and emerging applications in dermatology appear to fall into two main categories:
- Skin Image Analysis: Companies are developing applications and devices using machine learning and computer vision to analyze images to predict and prevent the onset of skin disease.
- Skincare Treatment Personalization: Companies are developing recommendation engines to tailor skincare treatment recommendations to user skin type.
In the article below, we present representative examples from each each category, as well as the current progress (funds raised, pilot applications, etc) of each example.
(Readers with a more broad interest for AI in healthcare can read our full article about healthcare machine learning applications.)
Skin Image Analysis
SkinVision
Founded in 2011, and based in the Netherlands, SkinVision claims that its mobile app uses machine vision to check skin lesions (moles) for risk of cancer via photo analysis.
Trained on a database of over 1 million images of skin lesions, the algorithm learns to recognize specific features such as size, color and shape and which ones may indicate a higher risk of melanoma.
When a user takes a photo of a lesion through the app, the algorithm categorizes the lesion as low, medium or high risk and provides recommendations for seeking treatment. Users who receive a high risk assessment also have the option of having their results reviewed by the company’s senior dermatologists.
The 30 second walkthrough below provides an overview of the application including how it captures and stores user data to track skin health over time.
SkinVision appears to primarily market its services to consumers. The company offers two free risk assessments before requiring the purchase of one of 3 user plans including a 12 month, unlimited-access package for 25 euros (approximately $31 USD). The company features client and dermatologist testimonials on its website, however results on the impact of the app on early detection is not currently available.
It is important to note that SkinVision provides a disclaimer that its “results are not diagnoses.” This pertains to results from the app and those provided by the company’s medical experts. SkinVision advises users with any serious concerns to consult with a physician.
A 2017 study was published on the SkinVision app in the journal of the American Telemedicine Association. The study was conducted by researchers in the Department of Dermatology at Catharina Hospital Eindhoven in the Netherlands, and found that “the algorithm scored 80 percent sensitivity and 78 percent specificity in detecting (pre)malignant conditions” based on a sample of 108 images.
The researchers concluded that app was “less accurate than the dermatologist’s clinical eye” but can still serve as effective tool to help non-dermatologists differentiate between benign and malignant lesions. To date, SkinVision has reportedly raised $6.7 million in seed funding and according to its website claims it has 1 million users worldwide.
Skin IO
Launched in 2008, Chicago-based company ECD-Network has developed Skin IO, an app that reportedly uses deep learning technology to screen for skin cancer through mobile devices.
The app’s algorithm is trained on an extensive database of clinical images of skin conditions. It is unclear as to where the database images were sourced.
Patients begin by downloading the Skin IO app and signing up with the Skin IO’s network.
Users take photos of the region of their body that they wish to have analyzed. The system is reportedly designed to also process entire body regions. Such scans appear to be integrated using an overlay feature in the app camera.
Within predetermined time frames (i.e. monthly) users will be instructed to submit follow-up photos to track any potential growths or changes on the skin that could be symptomatic of skin cancer and other skin diseases.
Examples of skin conditions that the app is designed to detect include Solar lentigo, Lipoma as well as malignant Melanoma.Submitted photos are analyzed by licensed dermatologists within the Skin IO network.
Users may be instructed to submit further photos or to schedule an appointment immediately, which can be done through Skin IO’s platform. The platform also provides users with reminders of when to submit photos for analysis.
To encourage sign ups, the company allows for the upload of one set of baseline photos for free. SkinIO charges $150 for an annual subscription allowing users to take follow-up photos every 3 months. Dermatologists who use SkinIO’s platform would be able to bill patients for the initial full-body region scan taken in their office. SkinIO would compensate physicians $25 per review session of the follow-up photos taken by the patient on a quarterly schedule.
The company claims that 1000 dermatologists using the SkinIO system, (enrolling 1 new patient per working day on the $150 annual subscription) would generate $39 million in annual revenue and $19.6 million in compensation payouts to participating dermatologists.
The company’s Image Processing Specialist, Nicolas Longo, has expertise in computer vision and leads the work on development of the image processing algorithm. Here is a short 2 minute video explaining how the Skin IO system works for patients:
MoleScope
Founded in 2012, Canada-based company MetaOptima claims that its MoleScope app uses machine learning to analyze and track the changes and developments of moles on the skin.
The machine learning technology of DermEngine, the analytics software that powers MoleScope, was reportedly developed by a group of researchers, scientists and physicians from the Simon Fraser University and the University of British Columbia.
The company’s website suggests that the device can assist in the early detection of some serious skin conditions such as melanoma. Users enable the app by attaching the MoleScope apparatus to their smartphone.
Once attached, the device allows users to scan their moles and send high definition images to their dermatologist for analysis. The device also claims to provide a guide for step-by-step self-check of moles so that users can analyze their own images from home before sending any information to their doctor.
Once their scans have been submitted, users can also communicate with their dermatologist through MoleScope for further instructions.The app comes with the option to access to DermEngine, MoleScope’s affiliated web platform. It also assists care providers in managing their work flow through its scheduling platform.
This 1:33 minute video explains how the app works to detect possible skin diseases or developments of existing conditions.
The company uses a patient and physician annual subscription model at $150 (including physician consultations) and $500 respectively. The company projected $4,117,750 in 2016 annual revenue based on 23,000 patients, 46 physicians and 89,000 case submissions.
Skincare Treatment Personalization
HelloAva
Founded in 2016, New York-based HelloAva Inc. claims that its chatbot called AVA uses AI to assess user skin type and to recommend cosmetic skincare products.
The chatbot’s algorithm was reportedly trained using dermatology resources from Mt. Sinai Hospital in New York. The questions were designed to reflect those used during a patient visit in a clinical setting.
The platform is accessible via both Facebook Messenger and SMS text messaging and begins with a series of 12 question prompts, allowing the chatbot to categorize the user’s skin type among 30 different variations. The information ascertained is then used to make specific skincare product recommendations.
Users also have the option of being connected with a human advisor to gain deeper insight on the recommendations offered by AVA and can also send a photo of their face to the advisor. However, it is unclear if the advisor would be a licensed dermatologist.
At the end of the interaction, users are provided with a direct link to purchase the products. The shipping process is handled by HelloAva.
The 1:18 minute video provides a demo of how the chatbot gauges user skin type and provide specific skin care recommendations.
The company’s current team includes three data scientists; one as an employee and two on the advisory board. California-based venture capital fund DHVC (Danhua Capital) is one of three angel investors who contributed an undisclosed sum to help establish HelloAva Inc.
PROVEN Beauty
Launched in 2017, California-based emerging startup PROVEN Beauty claims that it leverages machine learning to develop customized cosmetic skin care products.
The company’s algorithm is trained on a large skincare information database, known as the Beauty Genome Project, which was developed by the company reportedly includes 8 million customer reviews, 100,000 skincare products and 20,000 ingredients. However, the data sources are not specified on the companies website.
Through the company’s website, users begin by completing a series of questions to help develop their unique skin profile. Examples of data points collected include, ethnicity and skin type, level of water intake, and skincare goals.
Upon completion of the short questionnaire, users sign up to receive their results and personalized skincare products. A three week trial is currently available as part of the company’s launch for $39.00 and includes trial-size versions of 4 personalized products including toner and face creams.
Based on a subscription model, PROVEN charges a bi-monthly rate of $120. Clients received 4 full-sized products every two months.
Amy Zaoshi Yuan, PhD, co-founder and CTO of PROVEN is a data scientist by training educated at Stanford and USC. Information on company funds raised is not currently available.
Concluding Thoughts
Among current and emerging AI applications in dermatology, the dominant trend appears to be focusing on leveraging the power of image analysis to assist in the diagnosis process (readers with a deeper interest in diagnostic applications may want to read our article about machine learning applications for medical diagnostics).
The majority of companies are taking the route of using smartphone cameras, however, in the example of MoleScope, an image device has been integrated in an effort to mirror the higher resolution capabilities of a dermascope.
This is an important distinction as research suggests that dermoscopes have been shown to improve diagnostic accuracy and to reduce incidences of false positives in identifying melanomas.
This is particularly meaningful for encouraging use among licensed dermatologists and clinicians who are non-specialists. In the near future, we should anticipate increasing implementation of dermascope-like devices which are designed to be used with smartphone apps.
However, determining factors in the success of this business approach will be ease-of-use and profitability. With the current lack of data on funding, it is challenging to predict how quickly this approach may proliferate in the market.
New startups are emerging using a recommendation engine approach to matching user skin type with skincare products.
With these companies only within 1 to 2 years of operation, their ability to scale up their client reach and capacity will be critical. This is demonstrated in the ambitions efforts as in the case of PROVEN Beauty which aims to both manufacture the products and tailor them to each user.
Dermatologists and medical institutions will continue to be important advisors and collaborators in helping companies shaping their approach and delivering value to improve clinical workflow and efforts to achieve earlier detection of skin diseases.
Header image credit: Adobe Stock