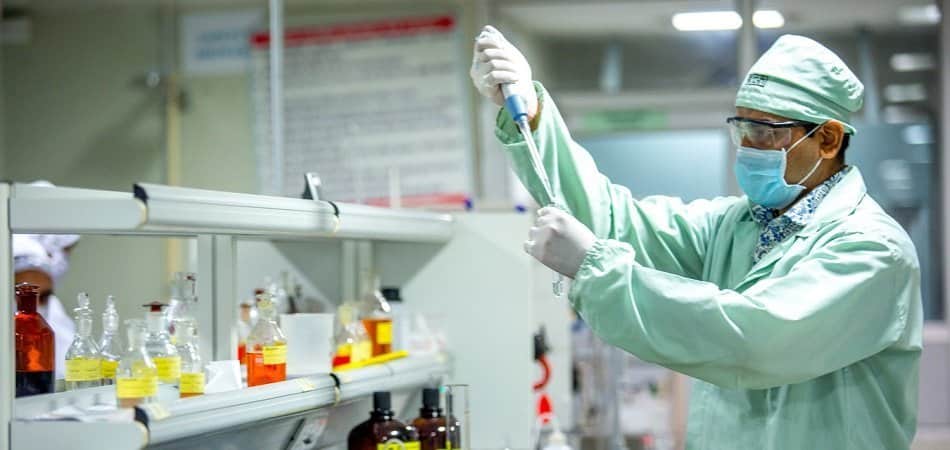
McKinsey estimated that embarking on digital transformation to restructure value chains and drive R&D innovation across the pharmaceutical industry could be worth $50–150 billion of earnings before interest, taxes, depreciation, and amortization. In particular, machine learning is likely to continue finding a place in the pharmaceutical industry. Pharmaceutical companies have found applications for machine learning ranging from drug discovery to clinical trial retention.
The State of AI in the Asian Pharmaceutical Industry
AI seems to be making its way into the pharmaceutical space in Asia over the last two or three years, particularly in China and Japan. For the most part, the companies offering or using AI for drug discovery are just starting to acquire funding and talent. XtalPi seems to have the highest density of talent with a decent likelihood of being able to work with machine learning.
The company employs numerous researchers with PhDs in fields such as biomedical engineering and other hard sciences. Virginia Burger is the Director of Scientific Affairs at the company, and she holds a PhD in Computational Biology from Carnegie Melon, which boasts perhaps the most renowned machine learning program in the world. As a result, Xtalpi’s small molecule discovery solution is likely robust, and the company is very likely legitimate in their claims to leveraging machine learning.
Cytlimic and Fujitsu offer software for predicting how well compounds will bind with each other and proteins. Cytlimic is a startup launched by NEC in 2016, and Fujitsu is a large enterprise with several tens of thousands of employees. These companies likely have the resources to acquire machine learning talent, and indeed, Cytlimic employs a president with a PhD. Deep Intelligent Pharma’s president also holds a PhD, his in Computer Science.
Although the initial talent working on the AI initiatives at these companies seem promising, the companies in this report don’t make available any case studies or provide any demonstration videos explaining their software. This likely indicates the nascency of these initiatives and the traction that AI pharma solutions have yet to gain in the Asian pharma market. Perhaps in one or two years, these initiatives will show more evidence of success.
That said, AI software in the Asian pharmaceutical space seems to cater to three applications, two of which involve drug discovery:
- Small Molecule Discovery
- Binding Affinity Prediction
- Medical Transcription
In this article, we’ll dive into four Asian companies using, offering, or developing AI software for the above four applications, similar to what we did in our report on machine learning in the European pharma space.
Small Molecule Discovery
XtalPi
XtalPi is a Chinese company that offers the Intelligent Digital Drug Discovery and Development (ID4) platform, which the company claims can help pharmaceutical companies predict the physiochemical and pharmaceutical properties of small-molecule candidates for drug development using machine learning.
XtalPi claims that it uses crystal structure prediction (CSP), also known as polymorph prediction technology, which calculates the crystal structures of molecules and their stability. CSP is important in drug development because many drugs are approved for only a single crystal form or polymorph. Medical treatments are often administered orally as a crystalline solid, and their solubility and shelf life depend on their crystal form.
XtalPi outlines their software’s process for determining the stabilities of compounds in 4 steps:
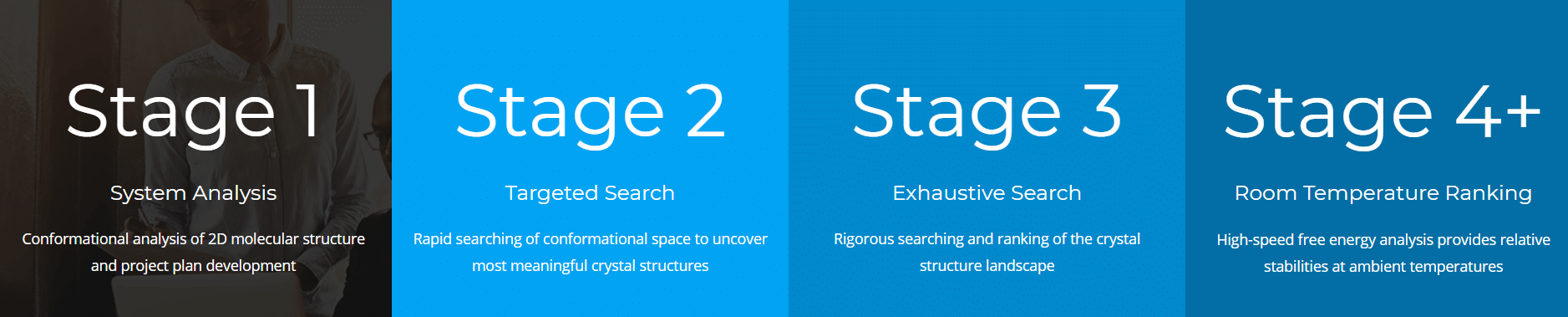
It’s unclear how the user interacts with the software and how machine learning connects the dots, but the company claims that the machine learning model behind the software was trained on chemical compound data and the corresponding amounts of energy they produce, as well as their behavior when they bind. We can infer the data was then run through the software’s machine learning algorithm.
This would have trained the algorithm to discern which data points correlate to low-energy compounds that would show stability upon binding. The software would then be able to accurately rank crystal polymorphs to determine the best solid-form candidates in the early stages of drug development.
We also could not find a demonstration video showing how the process works. The company does not make case studies nor a list of clients available, but it has raised $67.5 million in funding from the Susquehanna International Group, Tencent, and Sequoia Capital China, among a list of others.
Lipeng Lai is a co-founder at XtalPi. He holds a PhD in physics from the University of Chicago. Previously, Lipeng served as Research Associate at the Singapore-MIT Alliance for Research & Technology and a postdoctoral associate at the Massachusetts Institute of Technology.
Binding Affinity Prediction
Cytlimic
Cytlimic is a Japanese startup that was established by NEC in 2016. The company develops immunotherapy formulations for the treatment of cancer, including a cancer peptide vaccine, using machine learning. The company claims that the vaccines they develop aim to activate the human body’s immune systems to attack cancer cells. The formulations are also being promoted to pharmaceutical companies.
Cytlimic claims that the peptide selection process uses NEC’s “immune function prediction technology,” which virtually simulates the binding of peptides with antigens to trigger an immune response in the human body. Peptides predicted to have a high binding affinity are ranked and are considered candidate substances.
We can infer the machine learning model behind NEC’s software was trained on large numbers of amino-acid sequences of target antigen proteins. The data would then be run through the software’s machine learning algorithm.
This would have trained the algorithm to discern the data that correlates to the probability that specific peptides will bond with antigens. The software would be able to predict the candidate peptides to include in its cancer vaccines and other drug formulations. This may or may not require the user to upload information about their cancer treatment.
The company is brand new and seems to be in the early stages of both funding and talent acquisition. Shun Doi is the company’s president. He holds a PhD and is listed as having expertise in parallel computing, supercomputing, linear algebra, and parallel algorithm. Previously, Doi served as an executive expert at NEC.
Fujitsu
Fujitsu Laboratories is a Japanese company that offers molecular simulation software for drug discovery, which it claims can help pharmaceutical companies and drug research centers estimate the binding affinity of protein molecules. The company explains that the software predicts how well the protein molecules will bind to disease-causing proteins, which in turn could become candidate drugs for clinical trials.
Fujitsu claims that the software’s Optimum Packing of Molecular Fragments (OPMF) module is used at the compound design stage to design a compound that effectively interacts with an identified disease-causing protein (target protein) and enables drug companies to generate new compounds. This is done on a computer. The software’s algorithms then take the computational equivalents of the compounds and target proteins and combine them.
Meanwhile, the software’s MAPLE CAFEE module predicts the degree to which the compound inhibits the activity of the target protein. The technology also considers the effect of neighboring atoms on the compound.
Our research yielded no results when we tried to find case studies for the software, but Fujitsu announced a collaboration with the University of Tokyo’s Research Center for Advanced Science and Technology (RCAST) from 2011 to 2014, during which the company and RCAST researchers designed small molecule drugs for the treatment of cancer and other diseases. The current collaboration focuses on designing small molecule compounds using RCAST’s research on disease-causing proteins, as well as Fujitsu’s OPMF and MAPLE CAFEE modules.
Shunji Matsumoto is the Executive Researcher and General Manager at the In-Silico Drug Discovery Research Division, Bio-IT Business Development Unit at Fujitsu Labs. In his role, he is responsible for drug design and data analysis using machine learning technologies. He holds a BS in Applied Physics from the University of Tokyo. Matsumoto also serves as a researcher at the same university.
Medical Transcription
Deep Intelligent Pharma
Deep Intelligent Pharma (DIP) is a Chinese company that offers AI-based medical transcription software, which it claims can help pharmaceutical companies market their products and create content for documents for regulatory purposes using natural language processing.
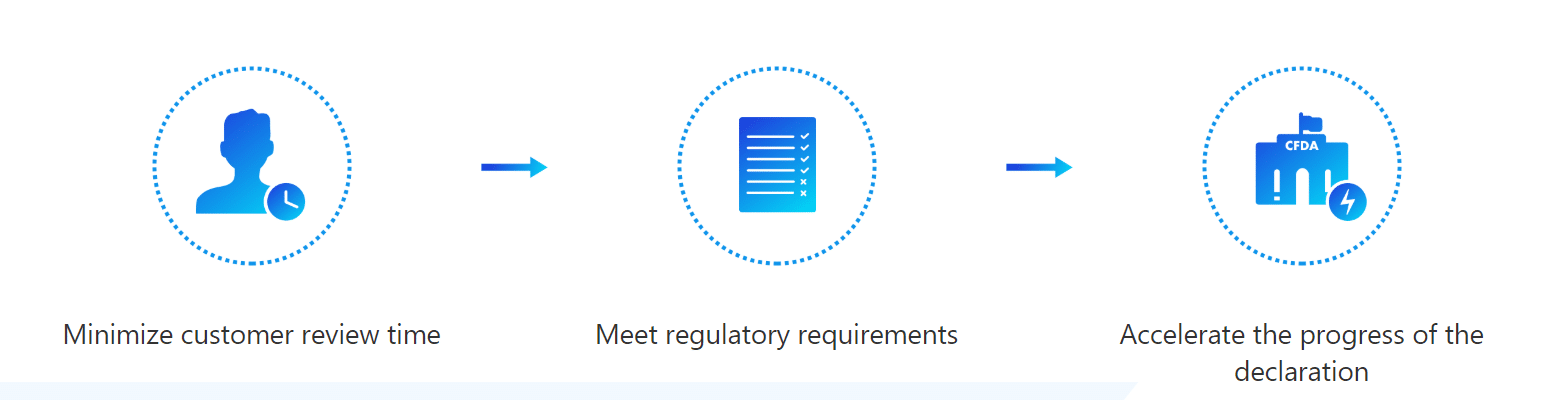
DIP claims that it developed a machine translation engine but did not explain how the AI-driven process works. The company only stated that the tool could help businesses translate marketing materials in a variety of languages. The company also did not specify what languages the software supports.
DIP also offers AI-enabled writing tools, including automated transcription, tabulated data analysis, document review, and quality control, among others. The company explains that the writing tools are targeted at creating content and documents to be submitted to regulatory bodies such as the Food and Drug Administration. The process was not explained, but the company claims it employs writers and AI professionals. How much of the writing is done by the AI and how much of it is done by human professionals is unclear.
DIP does not make available a demonstration video, case studies nor a list of clients. The company has raised $26.1 million in funding from Sequoia Capital China and ZhenFund.
Tong Xiao is the CTO, AI Scientist, and founding partner at Deep Intelligent Pharma. He holds a PhD in Computer Science from the Northeastern University of China. Previously, Tong served as visiting postdoctoral researcher at Cambridge University.
Header Image Credit: Asian Pharmaceuticals