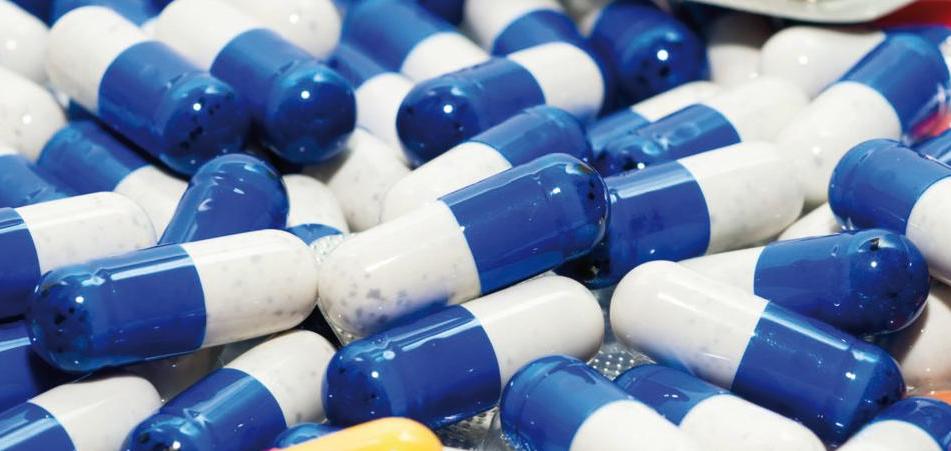
Generic pharmaceuticals require less research and development than their brand name counterparts. As a result, AI applications for research and development don’t seem to be the most prominent solutions for generic drug companies. That said, despite the lack of precedence, there may be many areas in which AI could help generic drug companies.
In this article, we discuss what might be possible with AI in the generic drug industry, such as:
- Finding Drug Biosimilars: Predictive analytics and natural language processing for searching through databases of brand-name drug compounds to find similar compounds scientists can use to make generic drugs
- Researching Drug Compound Crystal Structures: Predictive analytics for determining how the shapes of compounds will react to certain methods of manufacturing and other drug development processes
- Salt and Polymorph Screening: Machine learning for determining the solubility of a compound to make sure it maintains its effectiveness over time
We begin our exploration of AI for generic drug companies with how predictive analytics might help generics companies find biosimilars:
Finding Drug Biosimilars
Preprocessing Pharmaceutical Data for AI
Generic drug companies might use AI for finding alternatives to brand-name drugs, otherwise known as biosimilars. These companies likely have a large database of existing drug and chemical data, but that data may need to be processed and labeled before it can be run through a machine learning algorithm. Generic drugs need to have similar contents to their brand name counterparts. As such, data on these drugs might be labeled according to their solubility, their reactions to manufacturing, the shapes of their crystal structures, and other data points that an algorithm might use to correlate the drug to brand name counterparts.
Image data from advanced microscopes need to be labeled electronically based on what the company intends the AI algorithm to search for within those images. For example, microscopic images of a drug compound forming a crystalline structure would need to be labeled according to where the molecules bind and the shape of the structure.
Clinical trial data is typically written in lab notes by scientists conducting experiments. These notes include details about patient experience with a drug, how it affected their illness, and any side effects they may have suffered. Some patient data may already be labeled with IDC-10 codes. This can be helpful for generics companies in sorting out which clinical trial patients are good representatives of a given drug’s intended behavior. This allows the company to work toward a tangible result for testing their generic version.
Natural Language Processing for Isolating Biosimilars
In theory, a pharmaceutical scientist could search a brand name drug compound within the AI software, and the software would return biosimilars. They could then use these biosimilars to manufacture a generic drug.
Natural language processing-based drug discovery applications for example, are typically used for sifting through large amounts of clinical trial notes or electronic medical records. The application may then provide the user with all of the data the company has regarding a given chemical compound. This could help generic drug companies narrow down their known compounds to find the most affective biosimilar of a particular brand-name drug.
Generic drug companies may be able to use these applications to discover biosimilars. The company would then only need to test those compounds the software found to be closest to a the brand-name drug for which the company is making a generic.
Vender Landscape: BioSymetrics
BioSymetrics offers services for data organization, labeling, and cleaning to biomedical and healthcare companies. This data might include images, geolocational stats, streaming data, and the traits of previously discovered compounds. The company’s machine learning platform, Augusta, can purportedly recognize medical imaging data from MRIs and numerical data from EKG scans. It can also purportedly extract information from EMRs. Below is a full list of the data that Augusta can work with, according to the company’s website:
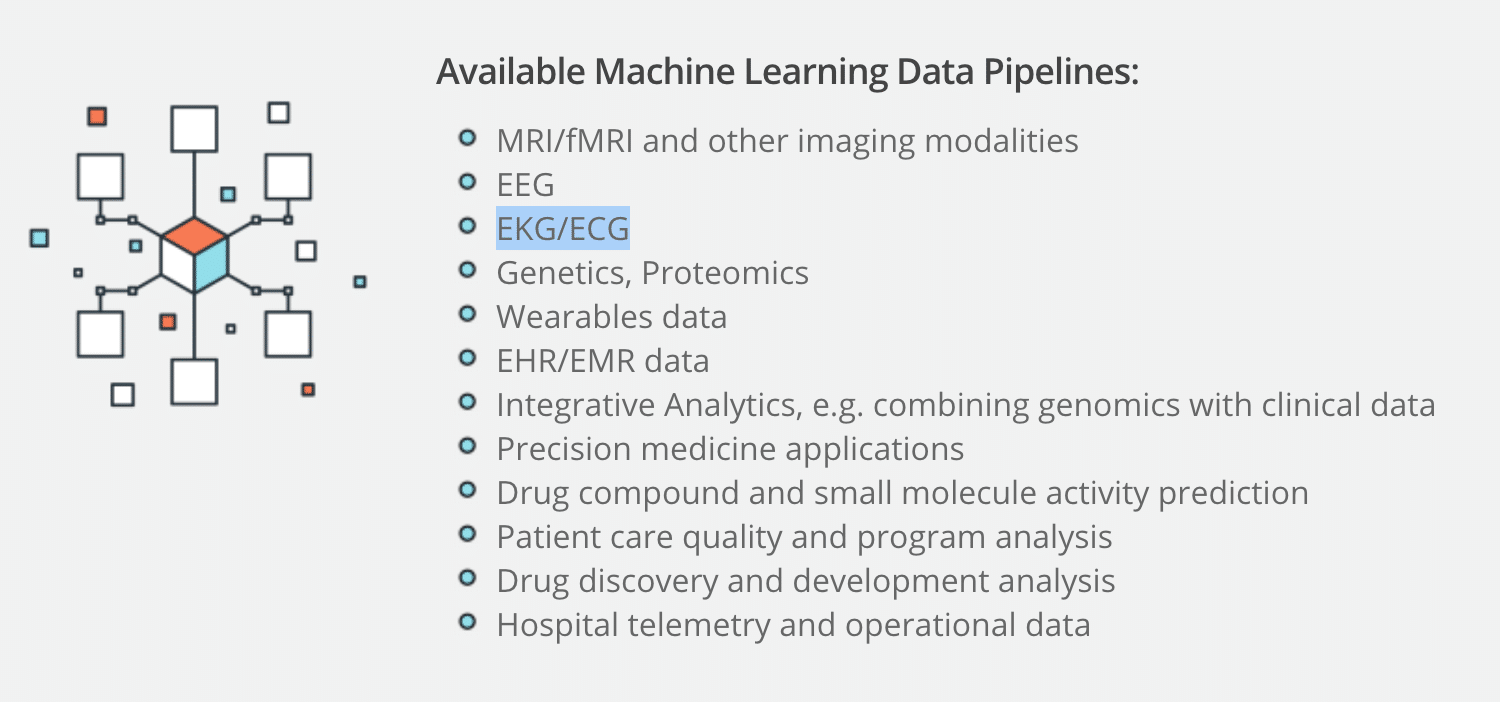
Augusta uses predictive analytics to determine if drug compounds will bind, among other use-cases, which could help generic drug companies determine if the biosimilars they discover can be combined to create a generic drug.
BioSymetrics claims its platform has uses in drug discovery, clinical trial optimization, and precision medicine. That said, they do not list case studies purporting a pharmaceutical company’s success with their software. They do, however, employ a Chief Scientific Officer with a PhD in Molecular Genetics. The company employs several data scientists with PhDs in similar fields. This bodes well for the company’s clients; BioSymetrics is much more likely to actually be doing AI than many AI vendors offering machine learning to pharmaceutical companies.
AI Integration Considerations
AI applications for finding drug alternatives likely need to be integrated more deeply into a client company’s workflows and systems than they first expect. We spoke to Zhigang Chen, Director of Healthcare Big Data Lab at Tencent, about how to handle the challenges of adopting AI into the healthcare industry. Chen said:
There’s a big gap between what the technology and the technology companies can offer, what the industry needs, or what the real world problems need. Take healthcare as an example. AI has offered a lot of wild expectations. But, what happened was when people try to apply the models, apply the AI system, in the real world, it didn’t work out quite well. That’s one.
And the second is, usually when we, as people who work in the technologies domain talk to you, the folks in the healthcare industry, we find there’s a mismatch. We thought that we were trying to solve their problems, but they said, “No, no, no. This is not the problem we wanna solve. We have these other problems.” There is a mismatch, in terms of priorities.
Chen highlights the difference in priorities between AI companies and the companies they serve. A generic drug company that employs in-house data scientists and subject-matter experts that know how to speak what we call the “language of data science” may find they have a smoother AI integration period.
Researching Drug Compound Crystal Structures
Some pharmaceutical companies use AI software to research the crystal structure of drug compounds when they are solid. A predictive analytics algorithm would need to be trained on data connecting the names of drug molecules to the crystalline shape of their solid form. The crystal structure of a drug compound can have an affect on how the drug should be manufactured. This is because the crystal structure may not react well with the rest of the ingredients that go into a pill or liquid medicine.
Generics companies may be able to take advantage of this type of application by using it to determine the crystal structures of their biosimilars. This may help them produce the drug to have the structural integrity it needs to not break down and stay effective.
However, finding useful training data for a machine learning model that analyzes the drug at the molecular level may pose a challenge to generic drug companies. This is because they may not have access to partnerships with healthcare companies or others that may have data they can use.
Salt and Polymorph Screening
When selecting a suitable biosimilar for their new product, generics companies may want to find information regarding the drug’s solubility. This information comes from data regarding how the drug’s crystalline structure breaks down when immersed in a solvent such as water or when ingested.
Applications for finding this type of information are most often predictive analytics applications. This is because they are able to form predictions about the solubility of a biosimilar based on large databases on past drugs, molecules, and research related to past clinical trials. This has the potential to increase the efficiency of discovering of viable biosimilars by gathering insight that is usually very time consuming to synthesize.
Some AI vendors claim their solutions can analyze large amounts of information on biosimilars to reveal information about their chemical traits, such as compound solubility and the shapes it can take when manufactured differently. A predictive analytics solution could analyze client research data on thousands of compounds for correlative data points about a compound’s solubility. This includes any previously discovered chemical reactions or shapes the compound can take in various states of matter.
Xtalpi claims to offer a software that can analyze molecules and search for crystal structures within its database Below is an image showing how their software purportedly does this:
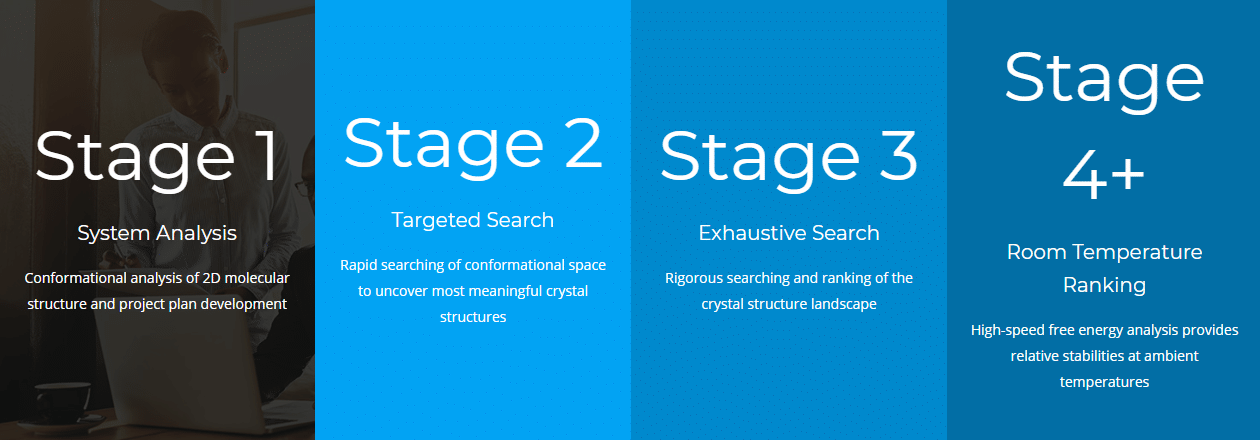
This could be helpful in determining many important factors to generic drugs, including how the active compound in the original drug reacts to being processed and manufactured differently. Additionally, these types of applications could reveal the solubility of the drug and if the compound stays effective when dissolved.
We could not find any results showing a generic drug company’s success with a vendor’s software. Additionally, we were unable to find any case studies from any AI vendor selling these types of software to generics companies.
Perhaps the most obvious reason for this is that the drugs these companies are handling are usually well researched, and biosimilars do not tend to be too volatile in terms of the manufacturing process. However it could also be because generic pharmaceutical companies are prioritizing innovation in other areas, such as white collar automation.
It is important to note that our research also did not yield much evidence of the top generic pharmaceutical companies leveraging AI. This widespread lack of adoption could be indicative of the fact that the brand name pharmaceutical industry has more practical uses for these innovations along with the means to integrate and develop them further.
We interviewed Abinash Tripathy, Founder and CSO of Helpshift, about the challenges of enterprise adoption of AI solutions. When we asked him about how innovations from the top global companies will find their way to more companies, Tripathy said,
Now what startups will do, like us, they will basically take all of these algorithms that get pumped out and figure out how to apply them to specific business cases, so it’s the application of AI. So I call it applied AI. So most of the startups that you will see emerge will be just applied AI companies. They won’t be core AI companies.
With regards to the pharmaceutical industry, we can infer that generic pharmaceuticals would largely make use of applied AI technology when working with AI startups. Additionally, they likely will not see AI solutions developed in house until more of the name brand companies have their own proprietary AI platforms and business infrastructure.
Header Image Credit: Monicakrish