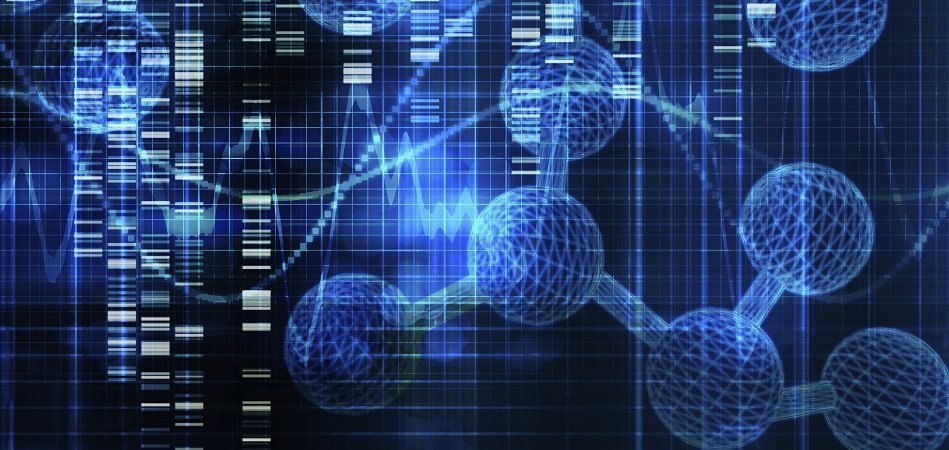
It may feel as though AI applications like machine vision and natural language processing hold the most potential value to pharmaceutical companies because of their capabilities to intake and transform unstructured medical data. This is especially true with machine vision, as medical imaging data can be used across multiple departments when analyzed by AI software.
Despite this, there is still a relatively equal amount of use cases for predictive analytics software that requires more prepared and structured pharmaceutical data. Leaders designing clinical trials may benefit from a predictive analytics tool that can compare their plans with the operations and procedures of past trials. A user may also be able to search for past trials based on the type of drug being tested and find which practices led the leading drugs to success.
The same is true for the discovery of new molecules and compounds that can be used to produce new drugs. Marketing teams may also benefit from predictive analytics solutions in that they can determine where demand for certain drugs may rise across different geographical locations. They may also be able to use the software to compare marketing campaigns for new drugs, strategies, and sales techniques with the current practices.
We spoke to Daniela Braga, founder, and CEO of DefinedCrowd, a data enrichment and crowdsourcing company, about how business leaders can determine their data needs for new AI projects. For pharmaceutical companies, this would be especially important for predictive analytics solutions as finding the correct data set amongst large stores of legacy data can be difficult.
With regards to cleaning and preparing data for use in machine learning models and data science experiments, Braga said:
So you need to collect data, you need to annotate data. There is a lot involved in entity tagging. It is one of the most difficult tasks especially, in domains. You need sometimes domain-specialized people to annotate those entities. And there is a whole way around measuring the quality of the people because we combine people with machines to make our data processing more efficient and accurate.
We caution business leaders looking to implement predictive analytics software to be aware that cleaning and structuring their data for processing by a machine learning model may be necessary.
This report covers possible use cases for applying predictive analytics to the following business problems facing pharmaceutical companies:
- Clinical Trial Design and Optimization
- Drug Discovery
- Marketing
Interested readers may want to read our full reports on AI in the European pharma industry and AI in the Asian pharma industry.
Our report begins with the uses of predictive analytics for the design and optimization of clinical trials, and how business leaders can gain a better understanding of past trials for similar drugs to the one they are looking to test.
Clinical Trial Design and Optimization
The most prominent use case for predictive analytics in pharmaceuticals is in the design and optimization of clinical trials. AI software solutions could analyze patient profiles and their medical histories to determine which patients will respond best to the drug being tested. This can help pharmaceutical companies save time when trying to find the best patients to inquire about enrolling in the trial.
Some predictive analytics software can be used for genetic clustering, or the segmentation of a customer base into groups that reflect each individual’s chance at responding well to the drug.
Companies can also make use of their patients’ medical histories here because there are solutions that can let companies detect adverse effects of the drug being tested before they happen. This could come from an allergy or a history of using a drug that is known to interact badly with the drug being tested.
Pharmaceutical companies can also use predictive analytics for finding and establishing best practices for clinical trials based on their past trials or a database of trial data from other companies. These documents would include information about clinical trial operations, procedures, and the relative success of the trial.
An AI software can then sift through all of this data to find examples of similar trials with varying levels of success. This would allow a business leader to compare their plans for a new trial with the operations of one that may not have seen success for the same type of drug. Conversely, the business leader could also find a new approach to clinical trials they want to adopt. Some predictive analytics solutions will automatically highlight similarities between past and current trials for the user or have some infrastructure for the user to create a predictive model that might illustrate them more directly.
Important to most clinical trials is the eventuality of fulfilling market demand for that type of drug. Because of this, pharmaceutical companies may want to use analytics to gauge market demand and how likely doctors are to prescribe their product. This can be done using electronic medical record (EMR) data, physician data, and sales data.
Below is a demonstration video from Dataiku regarding their DSS software platform. The video shows the user’s process for utilizing sets of data to predict doctors’ prescriptions of different drugs. The video is 13 minutes long; however, we have found the most important sections and have listed them here:
- 0:00 is where the user begins with finding the necessary datasets for the prediction they want to make.
- At 2:30 the user explains the goal of this analytics experiment and combines datasets to see if there are any contradictions or incongruencies.
- At 6:30 shows how the user joins datasets and “cleans” any incongruencies between the datasets. For this exercise, the user needs to make sure all of the physician ID numbers show up accurately or find out why certain ones may not be there.
- 8:25 is when the user generates all relevant data into a single table, where they can find certain rows that can be combined into a single one for less granular categorization.
- At 9:58 the user takes all the cleaned and organized data and utilizes it to generate a predictive model.
Unfortunately, our research yielded no results when we searched for case studies showing a pharmaceutical company’s success with predictive analytics software for this purpose. It would appear that this use case is relatively nascent compared to others, but vendors such as Dataiku, Domino Data Lab, and Medidata are offering this type of software to clients in and adjacent to the pharmaceutical industry. These clients include Primera Blue Cross and Worldwide Clinical Trials.
Drug Discovery
Another pharmaceutical area that predictive analytics can serve is drug discovery, or the methods by which companies can isolate specific molecules and test their effectiveness for treating disease and illnesses.
We spoke with Murali Aravamudan, founder and CEO of Qrativ Biotech, about the possibilities of AI and ML within pharmaceuticals. In the interview, Aravamudan highlighted “the ability to essentially take lots of drugs and assets that have gone through some amount of pre-clinical testing, but make use of the other assets of data that we have and come across some new pieces of information” as an important difference between AI drug discovery methods and drug discovery methods from the recent past. It is clear from this statement that he sees AI and data analytics as an important step towards driving efficiency in drug discovery.
Some AI vendors are offering software that can utilize large amounts of pharmaceutical data to determine the physical and chemical attributes of a drug molecule that are important to gauging long term success. These could be the way they interact with other molecules and how the atoms of that molecule must fit together. Predictive analytics solutions can search through data points about any molecules with similarities to the one being tested and compare their success or failure to help business leaders understand the molecule’s commercial viability.
Once a molecule has been isolated and identified, a company could use predictive analytics to determine how likely it is for a drug to do well when tested. This would come before the drug ever goes to clinical trial so that business leaders can work on finding the safest drug to test. This would be based on past clinical trial data, most specifically of drugs made up of similar molecules and compounds or drugs made to treat the same illness.
In order to use predictive analytics or possibly other AI applications to analyze drugs at the molecular level, other technologies may have a role to play in facilitating procurement of data with which to train machine learning algorithms. This is true for AI vendor Xtalpi, which combines predictive analytics with a high process computing engine based in the cloud. They also claim that they use quantum chemistry and physics principles to aid in computing and analytics.
Xtalpi’s software can purportedly help companies find the best physical form to produce the drug in, whether it be in the form of a pill, gel capsule, or another manufacturing method. They state this can be helpful in later drug development stages including mass production and packaging of the drug. The solution may also offer information about the drug and the forms it could be produced in to help drive success in clinical trials and defend against patent challenges.
Xtalpi calls their combined predictive analytics and quantum chemistry solution, “crystal structure prediction” because they state it can determine the likelihood certain types of crystal structures will form when combining drug molecules. They offer four case studies on their services page to show the results of their software’s analysis of an individual drug.
It is important to note that the case studies do not mention pharmaceutical clients by name. Instead, the case studies highlight a large amount of information their software is able to glean from a sample of molecules for future drugs.
Within each case study, they detail the drug’s number of H bond receptors or atoms in the molecule or compound that can form chemical bonds with hydrogen. The studies also show the likelihood of the type of crystal structure the molecule will form based on the energy of the chemical reaction and the combined density of the atoms.
Below is a short, 6-minute video where Leila Pirhaji, CEO and founder of ReviveMed, explains the possibilities for drug discovery with artificial intelligence. ReviveMed is a 2016 startup in AI drug discovery using metabolomics, or data recorded from metabolic processes. She details how her company’s AI platform makes use of bodily attributes previously unusable by earlier AI technologies.
Pharmaceutical Marketing
Predictive analytics also has a place in the marketing sector and also has specific use cases for pharmaceutical marketing. The possibilities for these use cases are listed below:
- Analyzing sales rates and market conditions
- Determining sales statistics within territories and segmentation of those territories
- Optimizing marketing campaigns
- Finding new sales opportunities from existing customers
Companies can use predictive analytics solutions to analyze sales rates across different geographical locations. Insights include which retailers are seeing the highest sales of a product, which demographics are using the product the most, and a rise in demand of a certain product.
Predictive Analytics solutions can also segment sales rates across geographical territories assigned to smaller marketing teams within the department. These can help business leaders see which sales associates are driving the most sales or which territories may be more difficult to sell in than others. Depending on the factors that are hurting sales, such as newer sales associates still in training or a significantly low demand for certain products, business leaders can change the territory assignments to attempt to counteract those issues.
In addition to sales and market conditions, pharmaceutical companies can also model their marketing campaigns to see which types of campaigns see the most return on investment. If a company’s predictive analytics solution modeled both of these things, they may be able to find better marketing strategies as well as retailers that attract the biggest demographics currently using the product.
Another possibility is predicting customer opportunity value, or a customer’s likelihood to offer more opportunities to sell a product to. With customer profiles, retailer and sales data, and marketing investment data, a company could see which how many retailers that sell their products a customer encounters in their normal life. They could then tweak their marketing investments to include more retailers or advertisements in those areas.
It is apparent that the possibilities for predictive analytics in pharmaceuticals are widespread across different departments and applications. With both clinical and administrative solutions currently sold to the pharmaceutical industry, business leaders can be selective about which processes to automate. This would allow them to stay in control of their business operations while integrating new technology.
Header Image Credit: In-Pharma Technologist