Stockbrokerage might be viewed by investors as a traditionally human-based service allowing them to buy and sell equities. When looking at the shift in how stock brokerage is different today compared to the early 2000s, the largest change seems to be in software-based automation. Put simply, a lot of what was being done by humans (such as executing trades, giving advice to investors, discretionary trading) can now be done through software.
We aim to explore the current state of artificial intelligence applications in stock brokerage by using use-cases from companies operating in the space. We have grouped together the researched AI applications in stock broking within the following segments:
- AI for Trade Executions
- Automated Trading
- Advisory Services
Below we highlight use-cases for AI in trade execution and cases where businesses are actively using AI for automated trading. While we note that robo advisors could be another cluster of AI applications, we previously covered them in a previous piece called Robo-Advisors and Artificial Intelligence – Comparing 5 Current Apps.
AI for Trade Executions
Sigmoidal
Sigmoidal was founded in New York in 2016 and has around 18 employees today. The company offers consulting services for businesses looking to leverage AI in the finance, construction, military and technology spaces.
The company claims to be using AI for algorithmic trading in stock brokerage. They claim their software utilizes advanced statistical models machine learning to make high-speed decisions and transactions in the financial markets. Their software might help businesses with:
- Trade execution algorithms – When a trader executes a buy request, stock exchanges need to match these buy orders or bids, with sell orders in order to execute securities trades. Sigmoidal’s claims their software uses statistical techniques to break up trades into smaller orders with the aim of minimizing the impact on the stock prices after the trade is executed.
- Identifying opportunities for arbitrage — a process where investment managers can potentially take advantage of differing prices for the same asset (stocks, commodities or securities) in different markets. Sigmoidal claims their software can automatically search for such arbitrage opportunities and list them out to the investor in their dashboard.
According to Sigmoidal, an investment manager or a stock broking firm might use their platform for algorithmic trading applications. For example in the case of trade executions, traders and investment managers at a stock rocking firm might gain access to insights on the most optimized way to execute their trades by means of a dashboard.
The employees at a stock brokerage firm would specify the orders that they might want to buy or sell on the firm’s existing trading systems. The Sigmoidal platform (integrated with the trading systems) would prompt the user with the most efficient way to execute that trade with the aim of minimizing the effect on stock prices. For example the software might suggest using an alternative trading system (ATS) over the stock exchange to execute a particular trade with the aim of improving speed of execution and minimizing effect on stock price.
The stock brokers can then approve the trade execution method that was recommended on the Sigmoidal dashboard. The company claims this feature integration can help stock brokerage firms assist their traders, or clients, in executing trades much faster than they would doing so manually.
Although Sigmoidal mentions a case study where they claim to have used AI to optimize asset allocation for investment banking, we could find no robust case-studies or relevant demo videos for Sigmoidal’s projects in trade execution for stock broking.
The company claims to have worked on projects with NASA, DARPA, NVIDIA, Microsoft,
PwC, Goldman Sachs, and Intel, yet there wasn’t much information about said collaboration to state if these were one time partnerships or continuing clients.
Sigmoidal’s current Head of Artificial Intelligence Marek Bardoński has earned a bachelor’s degree in Computer Science from the University of Warsaw, Poland and has previously served as the Senior Deep Learning Research Engineer at NVIDIA.
Advisory Services
Kavout
Kavout is an AI investment platform that the company claims uses big data and machine learning to help investor and equity firms gain better insights and make smarter decisions. Using distributed computing and machine learning models, the company claims that its platform can analyze large amounts of unstructured data in real-time and identify complex patterns in the financial markets. For example, Kavout claims that their AI model Kai leverages deep learning for stock ranking.
Kavout claims their software uses machine learning techniques on data such as SEC filings, price patterns and sentiment analysis from news, blogs, analyst reports and social media feeds to coax out patterns that might help with more accurate predictions of future stock prices.
Employees in stock broking firms can log on to the Kavout platform and access a dashboard which offers insights on the predicted performance of selected stocks. The company offers this service through a web portal where brokers can log in to view stock performance notifications customized for them based on their trading history.
The trader or investment firm can then choose the stocks with relatively higher Kai Scores which Kavout claims will lead to better returns.
- According to Kavout, the Kai platform first analyzes financial data such as SEC filings, technical indicators, price patterns and sentiment analysis based on news, blogs, analyst reports and social media feeds relevant to a particular broker’s market interests.
- The platform then identifies the complex correlations between stock attributes that might affect the future performance of a particular stock (at a speed and scale which would be impossible for human investors).
- The platform might then show an investor predictive insights and an actionable numerical rank called the Kai Score. Higher Kai scores for a stock indicates a higher probability of them outperforming past trends in the next one to three months.
Below is a video from Kavout where CEO Alex Lu gives a pitch and shows concept images to explain how their products, including Kai oould help investors and stock brokers.
The photo from Kavout below further shows the predicted Kai scores for a number of different portfolios of stocks of S&P500.
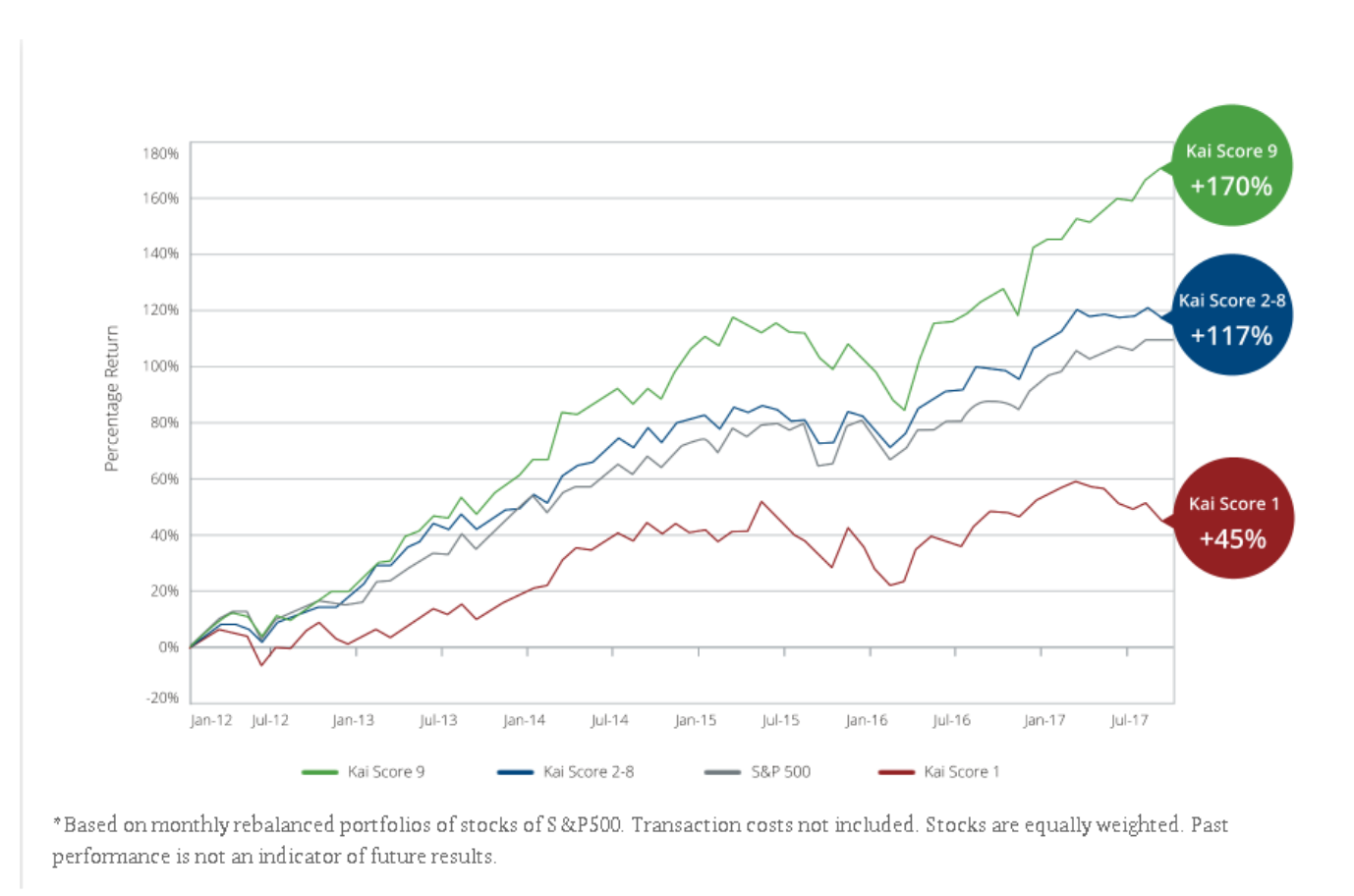
Investment managers might use the Kai platform to gain insights on which stocks to pick for a particular portfolio based on the Kai scores. According to Kavout users can also add the Kai Score to their own quantitative models to improve the ROI that might be gained using algorithmic trading.
CEO Alex Lu has previously earned a PhD in Computer Science from the University of Maryland. Lu has also served as Senior Software Engineer at Google, Principal Program Manager at Microsoft and Engineering Director at Baidu.
We could not find information on marquis clients or case studies where the Kavout platform was used successfully in stockbroking applications.
Discretionary Trading
Trade Ideas
Trade Ideas, founded in 2002 in Encinitas, Calif., offers a virtual AI-assistant called Holly which can prompt traders and stock brokers with trading strategies for individual stocks. The 28-employee company claims that Holly can execute discretionary trading for investors without needing much input from the traders.
Stock brokers can download the Trade Ideas windows desktop application and choose one of many channels such as ‘social media’ or ‘industry sectors’ to discover trades being mentioned most on social media platforms like facebook and twitter or look at the top performing stocks on the S&P 500 Index in sectors such as automotive or healthcare.
Traders can also gain access to insights from the Trade Ideas AI assistant Holly through a dashboard-like interface inside the same desktop application. Trade Ideas claims that Holly simultaneously tests several dozen different investment algorithms and coaxes out the best trading strategies by testing over a million trading scenarios overnight.
The software can then alert users with notifications for the trades with highest probability of returns the next day. The users can also give Holly the permission to execute trades automatically without any user intervention needed.
The image below from Trade Ideas shows a screen-grab from the Trade Ideas application along with the windows indicating the most discussed and trending stocks.
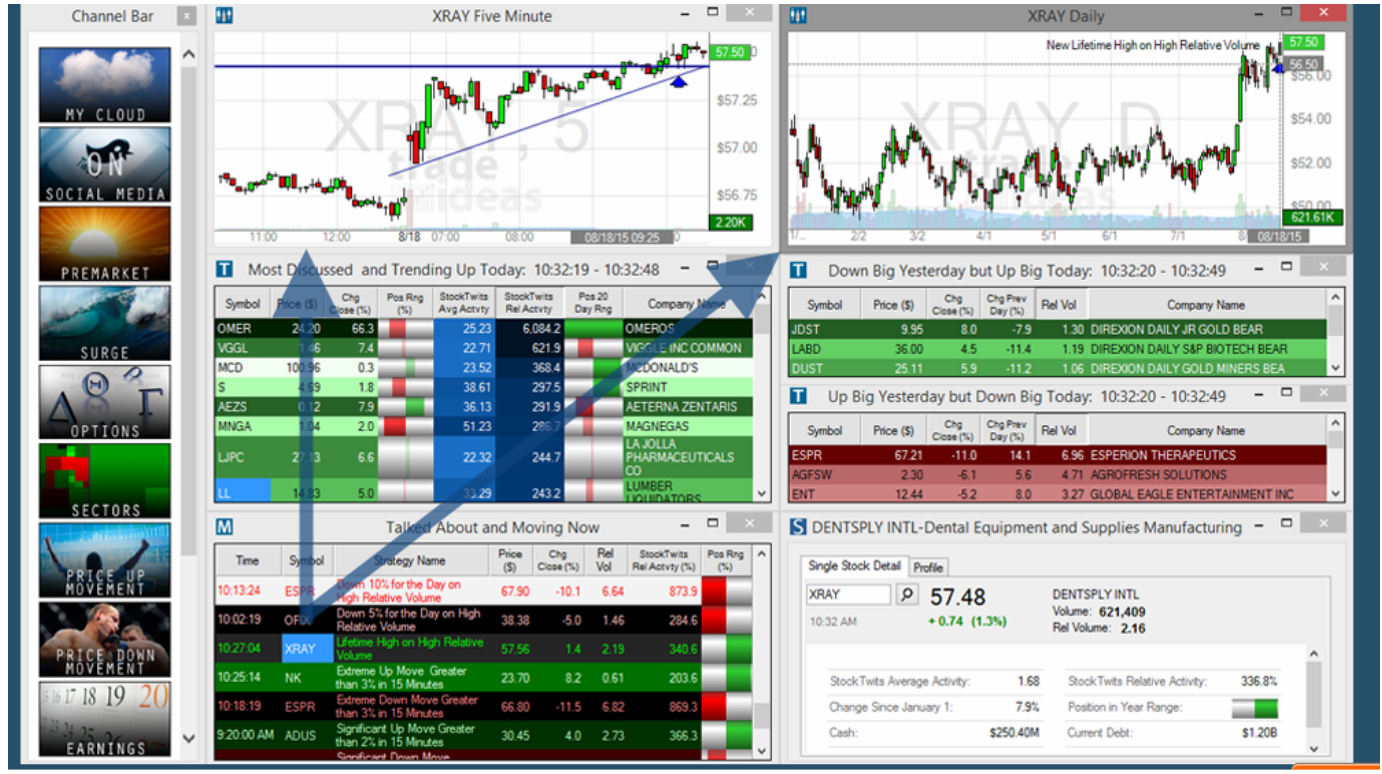
Trade Ideas claims that Holly evaluates all the different models each night and produces five to eight strategy suggestions to the client. In alignment with the client’s requirements, Trade Ideas claims these strategies are meant to help them identify successful opportunities in the market. These insights are then presented to the client in an AI Strategies Window on the software’s dashboard. The software also only chooses the strategies with a predicted success rate above 60 percent and a 2:1 profit margin.
Hedge funds, asset managers, registered investment advisors, financial institutions, online brokers and direct access traders use the software as an idea generation and risk management tool, according to the company
Below is a 5-minute video where CEO of Trade Ideas Daniel Mirkin explains how users can see insights derived from Holly to analyze potentially successful trading strategies:
We could not find any robust case studies for Trade Ideas software tools being used by stock brokerage and investment firms. Trade Ideas however claims that Holly was able to show a net return of 16 percent for Q1 2018 in Trade Ideas’ simulated portfolio. The company also claims that out of 747 total trades made by Holly in Q1 2018, the software made 54.3 percent of winning trades, which made a profit at an overall profit factor 1.54.
Below is a graph from Trade Ideas showing the simulated performance of Holly as compared to benchmark indices such as SPY or S&P 500.
Conclusions
From our research, we’ve determined a few strategies that stock brokers, traders, banks and other financial institutions may want to do to prepare for AI:
- AI trading platforms of the future might be capable of scanning most stocks relevant to a portfolio to identify patterns aiding in forecasting the performance. Trade matching and trade execution seem to be the low hanging fruit applications for AI in stock broking as seen with Sigmoidal.
- The next generation of Robo Advisors will mean that trader might change their financial strategy every three to five years and be able to track many different asset categories simultaneously in real time. Software tools like Kavout’s Kai score which predict the performance of stocks and portfolios might be more commonplace in the next three to five years with the advent of robo-advisors from most large investment management firms propelling this trend.
- As in the case with Trade Ideas, natural language processing and predictive analytics might be more commonplace in the next three to five years for applications like sentiment analysis in real time which are capable of processing news pertaining to a particular stock value. Companies mentioned above seem to be entering this opportunity area with their heavy use of sentiment analysis for stock broking
Header image credit: The Street