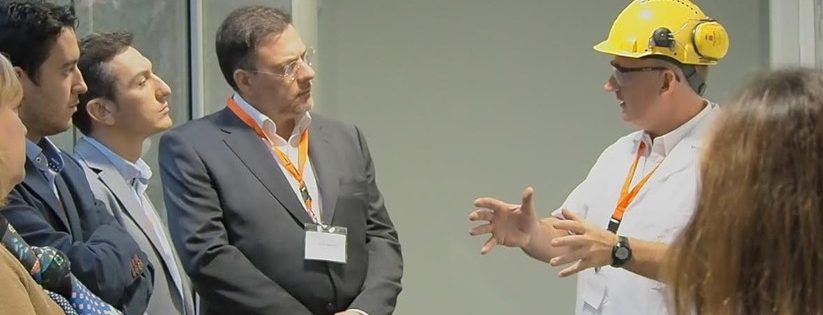
Episode Summary: For business leaders who are thinking about integrating AI into their company or who are just in the very beginning of that journey, this may be a useful episode of the podcast.
Many times, people think that finding the right talent is the biggest challenge when it comes to integrating AI into the enterprise. Much of our own research and conversations with machine learning vendors and the consultants trying to sell AI into the enterprise actually think there’s another, bigger problem: combining the expertise of subject matter experts and that of data scientists to leverage information for future initiatives in business.
This week, we interview Grant Wernick, CEO of Insight Engines in San Francisco. We speak with Grant about the initial challenges of organizing data and setting up a data infrastructure a business can use to leverage AI. We also talk about using data in leveraging normal workflows so that non-technical personnel can use it to drive better product innovation to help the company.
Subscribe to our AI in Industry Podcast with your favorite podcast service:
Guest: Grant Wernick, CEO and co-founder – Insight Engines
Expertise: natural language, intelligence augmentation, and machine learning technologies
Brief Recognition: Grant is an experienced product focused leader with over 15 years of experience building natural language, intelligence augmentation, and machine learning technologies. He is the co-founder and former CEO of Weotta
Interview Highlights
(04:04) Broadly speaking, if you just think about paradigms, what are the critical challenges that are keeping the non-technical folks from in any way gaining and receiving benefit from a company’s data?
GW: That’s an awesome question, Dan. And to answer that really well, I want to step back just one second. We live in a world today where we utilize probably 1% of the data coming off of all of this machine data out there, and we have many different places with throw data…it’s basically put into these data lakes—they’re basically swamps—and it’s very hard to get things out of these places unless you have a lot of technical knowhow.
And so today, we’ve been in this world of “put it somewhere, just put it somewhere, and we’ll figure it out later” kind of thing. “Just the fact that we have it stored somewhere, we’re going to be able the do something with it.” And then people start realizing, “ok, now we have it, what can we do with it?” There’s this huge talent gap.
In every organization I spend time with, there’s one or two people who are really the deep folks that can dig into that data, and everybody else on the team wants access to this stuff. They have ideas. These people are oftentimes domain experts. I live and breathe in security and IT, and you have people who are awesome at security, but they don’t always have the technical chops for the various different products an organization may bring on.
And so a lot of the day ends up being staring at dashboards, and not actually getting to exercise their creativity and dig into this data. We’re really at an interesting point where we are lucky to be the kind of company building things in this world because we’re at the point where people are starting to realize, “Hey, I got it. I want it. I want to do something with it.” We’re at the point where more of the office is curious. We’re at the point where the news has made it very interesting, and when insights do come out they’re always very fascinating. And so we’re at a point where people have a lot of curiosity to want to dig deeper, that computer is actually a point where it’s helpful.
What is just starting to happen is being able to organize this data and make sense of this data. So we live in a world of data. We live in world of log, store, search. We live in a world of “go and dig through my data lake,” but now you are starting to see a world where…”let’s make this accessible to more people.”
And this is where AI comes in, and it’s really a subset of AI. It’s intelligence augmentation. People have a lot of interesting ideas. Let’s help them up level themselves. Let’s help them have tools that enable them to be able to ask these questions of data, and so you don’t have to have secret super wizard skills anymore. But we’re just starting to scratch the surface.
(08:02) You talked about chucking [the data] somewhere. Is there a different way that you think people will need to start thinking about determining what to store, and how and where they’re storing it?
GW: So, Dan, people have to reverse their thinking on it. Instead of trying to huck their data somewhere, they need to start thinking in terms of, “hey what do we actually really want to get out of this? Oh, these are things we actually want to understand. We don’t want to understand something, for instance, we’re really curious about, people from a specific country accessing our systems. And so, that’s what we really want to know. Ok, what data sources do we need for that? How should it be organized?” And this is something a non-technical person can actually dig into and just really look at things from a use case standpoint.
It’s actually funny. I’ve been in development a long time. I always say 80% of your time should be spent figuring out the problems faced, and 20% should be actually taking up the code so you really understand what you’re actually doing. So this is a place that even with the current stuff, you have one or two people who are wizards on your team, it’s creating a lot more communication between them, and it’s that human-to-human element that we can do today.
Now, I’m going to pause for a second, because there’s a lot more to think about here. There’s a lot you can dig into on that. We are starting to see tools that allowed non-technical people to become a little bit of these wizards. You are starting to see interfaces that people can get access to, and you’re starting to see more and more. If enough thought is put into these stores upfront, you start to see more and more interactive charts and sites of visualizations. We’ve been in these amazing situations because of the kind of stuff we do. We have actually helped up-level people who have a lot of domain expertise, in this case their security guards, and they will become part of the cybersecurity team in a matter of a couple weeks. And that’s only possible when you start bringing it the right ideas from the get-go. So you start structuring the data well, then you start thinking about what you want to get out of it, and then now that it’s that way, you can bring in products that enable people that are not technical to start asking questions and dig into data.
(10:54) It seems like those conversations between subject matter experts and the data scientists upfront is the beginning of this pipeline of value sharing that eventually we get to. Is that safe to say, or would you put a caveat on that?
GW: Oh, it’s totally safe to say. And it goes into like I hear a lot of complaints with people: Oh, my gosh, I spent a fortune on infrastructure because we put all this data here. Oh my gosh, my license for X product is way too expensive and now I need to bring in more data to get the insights I want. It’s like go into that closet, let’s clean the house, let’s figure out what you really need in there. Let’s get that stuff in good shape, and you’re like, wait, I have plenty of space. I have plenty of CPU utilization and capability here. It’s like…we actually can do what I want, but because people have this unfiltered kind of aspect, and it’s kind of like mandates on the top: Just get it all. Yeah, it’s like more of that thoughtful process upfront will help people a lot, and it’ll also help you think more clearly, too, about the things you want to get done with the data.
(12:59) What are the broad paradigms that are shifting? How gradually is data interacting with and having a valuable relationship with non-technical people? What are the factors that are shifting in a half-decade ahead that you look out to that are permitting these case studies to even be possible?
GW: Yeah, pretty funny. We were just starting to see tasks that would take, in our case, in the security world investigations that can take two weeks so now taking a couple hours because the current way of doing thing is, “Okay, I need to investigate something. Okay, I need to get to the right person to go craft the right queries, well, that’s not exactly what I mean, and I need to dig in. Oh, wait, we don’t have the right data. We need you to go grab the right data. We need to go ingest that data. And a lot of things are done on the back end and not thinking about it at the front end when you’re actually trying to get things together. And so the marriage hasn’t happened beforehand. We’re just starting to see a world where people are being more thoughtful about this. We’re seeing, “Hey, we can get things done in a fraction of the time because machines can do a lot of this laborious processes data onboarding,” like data cleansing, riding the esoteric queries that are necessary to access data out of a data store. They’re able to start giving you visualizations that matter to a non-technical person, that a non-technical person can understand. That’s something I’m extremely passionate about.
But it really comes down to the marriage of focus. When you want specific insights, don’t try to boil the ocean at every facet of your business. So, if you are a healthcare company, for instance, there’s all sorts of interesting things you can do. You could do things from: What’s our security around patient records? What are doctors doing and how many visits have they done in the last couple weeks? and Are they sending people to ER? and that kind of stuff. You could do things around drug use and drug prescriptions. These are all very different facets. And if you start thinking about all the different facets, the data sources become immense. And so my big piece of advice there is rank these things, figure out what is most important, and start drilling them down, and do one at a time, and use one as a sample set. If you do that, you end up in a situation where you get a really good bright star of success before you start drilling into all the facets of your business where you want insights. That seems to be a shift that people need to start focusing on more since we come from this data lake or data swamp world. It’s just been like, “Oh, I can do everything!” and nothing gets done. You just focus.
(16:11) So it sounds like when big decisions are made, the continued merger of subject matter expertise and data sciences is just something that has to more and more become the norm. Is that trend actually starting to happen? I still see a lot of silos, but i hope they’re gradually being chiseled away at.
GW: Yes, they are gradually being chipped away at, but it’s still a pretty siloed world. It’s very rare that the domain expert and the technical expert are the same people. If we want to get the kind of insights that we dreamed about and see in the movies and futuristic stories talking about, there has to be this blending of, “hey, you don’t need to be a technician anymore. You need to be a domain expert.” This is where AI really comes in, and what we should embrace very fully is that if they use it in a great way, really structured way, then we can actually employ the right kind of products on top of it. Then the people who are the domain experts can have direct access. That’s a world I get super excited about.
(17:39) We actually don’t have enough precedents to know the way, but are we on our way to bridging the gap between the data science and subject matter expertise people?
GW: We absolutely are, and the challenge is that people need to embrace new technologies and try things out. As far as technology goes…we’re at a point where it’s ok to start figuring things out. It’s okay to start looking at this brave new world.
(18:30) You had mentioned the example of the security guard. What did that look like and how does that represent the dynamic we’re talking about?
GW: There was this CSO, and he’s like, “Awesome, your technology helps me. I marry my data and technical knowhow and people have domain expertise. Awesome, let’s just grab some people with only domain expertise and see if this is possible.” He really challenged me, and over a three-week period we helped him get [his company’s] data in better shape. [That was] step 1. Step 2: we set a nice framework so their technical people could work side-by-side with their non-technical folks. Then we put their people on a keyboard and gave them a tiny bit of training. Within a matter of three weeks, they come up with new use cases and dynamic things they can do with it and help start to move their organization beyond a world of static dashboards and static frameworks, which got me so excited because that’s why I do what I do.
You’re talking about cyber security: zeros and ones. You’re talking about a very technical team, and you’re talking about a group of security guards that stared at security footage. Some of these guys are ex-cops. They have a really investigative mind. You’re bridging that gap, in this case, utilizing our product to do so where people are able to get there and say, “Hey, I need to see a network traffic to China today,” and in literally seconds say, “Hey, there you go.” These are the things that would take an advanced person a good amount of time to do. And so they actually start using that creative mindset to start digging into stuff.
(20:43) Would you say that the challenge is to make sure you can make things simple for folks to move them around and add value if there’s stuff to drag and drop and enter and search for, and make sense into it?
GW: Absolutely, from an interface perspective. From a data perspective, if you haven’t taken the time to have good data hygiene, it’s garbage in, garbage out. So you can put these domain experts on top of something, and they are not going to get the answers they want. They’re going to get very frustrated. And so you really need to take the time to have good data hygiene before any of the new technologies make any sense. Anything you’ve seen out there, regardless of what it says, requires you to take the time and have good data hygiene.
Subscribe to our AI in Industry Podcast with your favorite podcast service:
Header Image Credit: Direccion por Misiones