Discovering a new drug is a long, expensive and often haphazard process. Thousands of compounds are subject to a progressive series of tests, and only one might turn out to be a viable drug. Any tool which can speed up just one of these steps in this long multi-step process would have big implications down the entire chain. This is why some of the largest pharmaceutical companies are turning to AI to help the process.
This article explores how five of pharma’s biggest companies are applying (or attempting to apply) AI and machine learning to improve drug discovery. We aim to answer the pressing questions that pharmaceutical business leaders are asking today, including:
- How are industry giants like Pfizer and Merck using AI to develop drugs today?
- How are large drug development firms partnering with startups to fuel innovation?
- How do industry-leading pharma companies expect AI to change the process of drug development in the future?
To fully understand why drug companies are turning to AI requires a basic knowledge of how drugs are discovered and approved, so we’ll start with a simple overview of the drug discovery and approval process (if you’re an industry veteran, feel free to skip this section and scroll directly the AI use cases of the “big 5” drug makers).
Basics of Drug Discovery and Approval
In simple terms, each potential drug is filtered through a system of a dozen progressively more expensive and involved tests/trials to determine if the potential drug is both safe and effective.
The below video from Pharmaceutical Research and Manufacturers of America (PhRMA) provides a concise overview of the process in the video below:
The drug company will learn about a new biological discovery that provides insight into how the human body or harmful bacteria functions. The company will then examine numerous compounds to find ones that may act on a specific discovery. In the lab they will do basic tests for toxicity, to determine if the compound can be absorbed properly, to study how it might be metabolized etc.
Only compounds that pass these early tests will potentially move on to clinical trials so they can get government approval. There are three phases of clinical trials, with each step requiring a larger number of volunteers and with more stringent criteria to pass. The process takes several years and only a fraction of drugs make it through.
According to the Tufts Center for the Study of Drug Development, the average cost to develop and gain approval of a new drug is $2.558 billion. Their figure is based on an estimated average direct cost of $1.395 billion and $1.163 billion of time costs (expected returns that investors forego while a drug is in development).
PhRMA claims that the process, from initial discovery to the marketplace, take at least ten years, of which around six to seven of those years are spent undergoing clinical trials. According to the industry groups, the success rate for drugs is remarkably low. Less than 12 percent of drugs that enter clinical trials will eventually be approved. PhRMA visualizes the lengthy process with the graphic below:
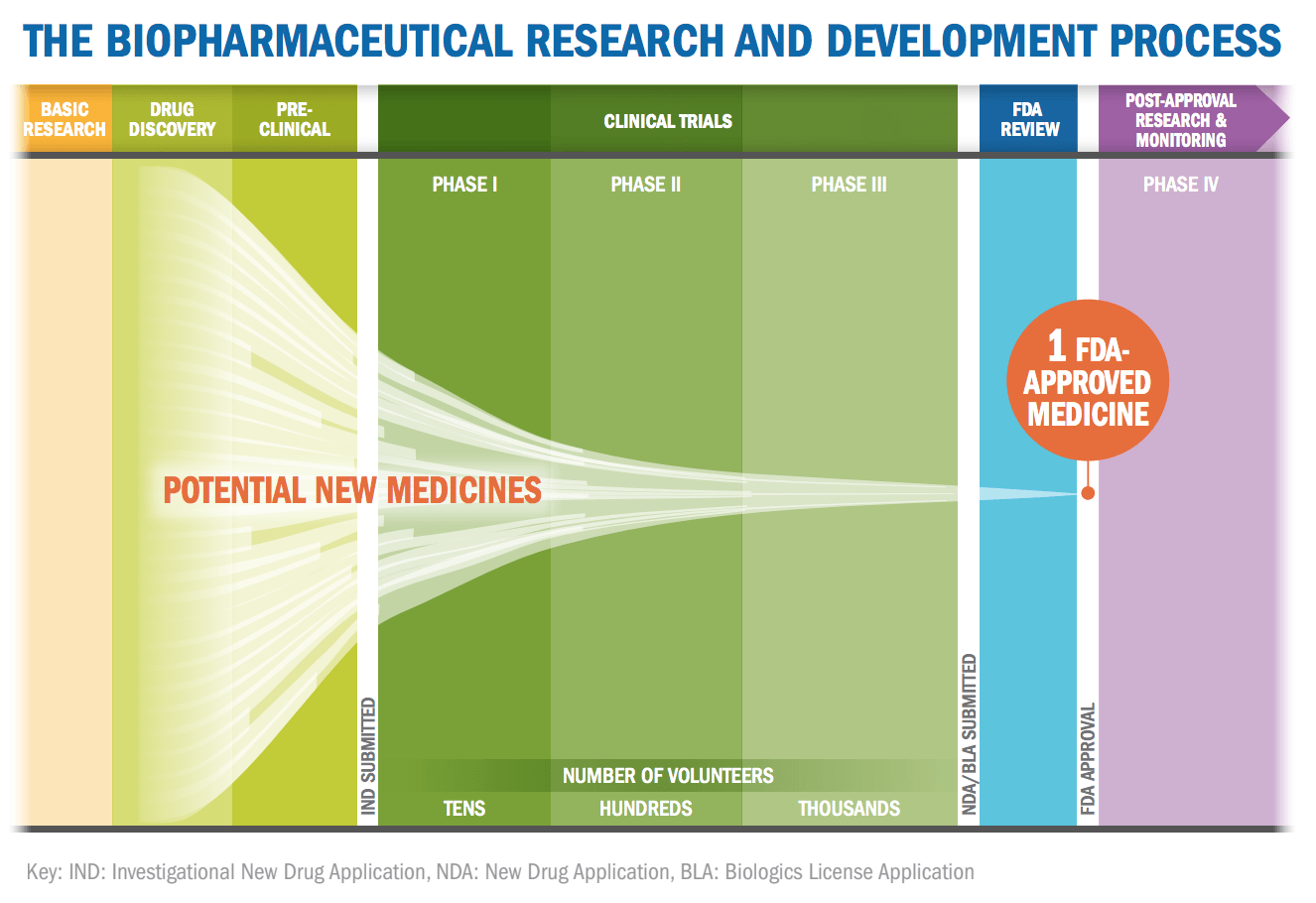
FDA approval is not a 100 percent guarantee that a company will see a return on their investment. There is always the possibility the FDA might pull the drug after discovering problems among patients taking it or the remote possibility another company might soon after release a better drug that steals it market.
If AI or machine learning can improve that success percentage up just a few points to, say, 14 percent or 16 percent, it would be worth billions to the industry. A program that could, for example, better predict the likelihood of toxicity in the earliest stages before the company even tries to take a drug to clinical trials would directly save the company millions and importantly save it time. Companies have limited bandwidth when it comes to running trials. Any way to slightly improve any of the early screenings compounds benefits down the entire development timeline.
Some of the largest pharmaceutical companies are attempt to make use AI in the following capacity:
- Find new compounds that could be potential drugs
- Predict what how well potential potential drugs will do in testing
- Discovery drugs that could work together as a combination for treatment
- Find new uses for previously tested compounds
- Create personalized medicine based on genetic markers
(For a broader look at the specific AI investments of the 5 largest pharma giants, check out our full article titled “AI in Pharma and Biomedicine“.)
The Pharmaceutical Companies
Many of the top pharmaceutical companies have only relatively recently made serious efforts to apply AI to drug discovery, but there have been several high profile deals, partnerships, and collaborations in the past few years. In the sections below we’ll explore the present initiatives and applications that seemed important, or indicative of a greater shift in the sector:
Pfizer Collaborating with IBM
Pharmaceutical giant Pfizer in late 2016 announced a collaboration that will utilize IBM Watson for Drug Discovery. Pfizer is using IBM’s AI technology on its immuno-oncology research, a strategy of using a body’s immune system to help fight cancer. Based on our research, this appears to be one of the first significant uses of Watson for drug discovery. The move was a big public announcement for both Pfizer and IBM.
IBM points out the average human researcher reads between 200 and 300 science articles a year while Watson has “ingested 25 million Medline abstracts, more than 1 million full-text medical journal articles, 4 million patents and is regularly updated.”
The value proposition of having machines do the reading is a strong one considering the huge volume of medical literature published each year – but it seems unclear whether Watson has “cracked” the problem entirely and made its value proposition actually work for hospitals and drug developers.
One of the hopes is that Watson’s deep pool of information can enable it to make non-obvious connections that could lead to combination medicines for cancer. Finding combination medicine is even more challenging than looking for a single drug treatment.
Pfizer President of Innovative Health Albert Bourla told Wired the best way to get the body to fight a tumor is “some combination of agents to spur the immune system into action. But possible combinations are countless, so the greatest challenge is to find ways to narrow the field and predict what combinations might be more effective. Thanks to Watson, which has been trained with historical data, we’re trying to predict the winning combination.”
(At about 22 minutes into our interview with IBM’s Swami Chandrasekaran we go into more some additional examples of applying “precision medicine” approaches with AI – listen to our interview with Swami here.)
Roche (Genentech) Collaborating with GNS Healthcare
Genentech, a member of the Roche Group, this summer announced their own collaboration with Cambridge, MA-based GNS Healthcare. GNS Healthcare’s mission statement is to use the latest innovations in machine learning to turn biomedical data into solutions and treatments.
This collaboration’s first focus will also be on oncology, just like the IBM/Pfizer deal. Genentech plans to use the GNS REFS™ (Reverse Engineering and Forward Simulation) causal machine learning and simulation platform to find and validate potential new drug candidates. They will also look for genetic patient response markers that could lead to targeted therapies.
One problem drug makers have run into is that a potential drug will sometimes only seem to work for a small group in the clinical trial. Or on the other hand it will be deemed unsafe because a small percentage of patients developed serious side effects.
Cheap genetic testing has made it possible to determine if there is a genetic reason for this. It is possible for a drug to work but only for people with a specific gene. Tailoring treatment to individuals genes is at the core of so-called personalized medicine and is a big focus of GNS Healthcare.
personalized medicine is seen as having significant potential for the future, and machine learning could play a role in determining what genes and genetic markers are interacting with a possible treatment. Earlier this year the FDA approved Keytruda (pembrolizumab), the first treatment only for patients whose cancers have a specific genetic feature.
Only a small percentage of patients’ cancers have these specific genetic features, which would make it ineffective to give this treatment to most people but effective for this small subset. Drugs that are only usable for a small number of people with a particular gene are becoming more common.
The program is just beginning at Roche and reasonable results or evidence of ROI (good or bad) won’t be expected in the near term.
Sanofi Collaborating with Exscientia
One of the largest by drug discovery collaborations and strategic leasing agreements announced this year based on possible revenue was between pharmaceutical maker Sanofi and artificial intelligence driven drug discovery company Exscientia. In the video below Exscientia CEO Andrew Hopkins explains how their system works.
Exscientia will use its AI powered system to identify and validate combinations of drug targets that could work synergistically. It will generate bispecific-small-molecule compound designs for Sanofi. Effectively, Exscientia is responsible for inventing new potential drugs while Sanofi will be responsible for making them, testing them, and bringing them to clinical trial.
Andrew Hopkins, the CEO of Exscientia, claims that compared to traditional methods Exscientia system can deliver drug candidates roughly 25 percent faster and 25 percent cheaper. The algorithms can design new molecules and is intended to be able to better predict which molecules would be the most effective and safest. If Exscientia managed to hit all the milestones in their agreement they could potentially receive EUR250 million from Paris-based Sanofi.
GlaxoSmithKline Collaborating with Exscientia and Insilico Medicine
Exscientia also signed a similar deal with pharmaceutical company GlaxoSmithKline in July. The drug maker is employing Exscientia to discover novel and selective small molecules for up to 10 disease-related targets. Achieving all their milestones in this deal would be worth £33 million (~$43.7 million USD).
Just one month later GlaxoSmithKline announced another deal with another artificial intelligence drug discovery company, Insilico Medicine. Insilico completed a series of pilot challenges for the drug maker and now GlaxoSmithKline will evaluate Insilico’s technology in the identification of novel biological targets and pathways.
These are just two of the many deals GlaxoSmithKline has reached with different companies to use new computer modelling systems to improve the discovery of drugs, biomarkers, and new vaccines.
(You can also read about GlaxoSmithKline’s AI applications in this featured natural language processing business use case.)
Johnson & Johnson and BenevolentAI
Unlike many of the other recent deals between established drug makers and start-up companies using AI in drug discovery, last year BenevolentAI reached a deal to license potential drugs from Johnson & Johnson. BenevolentAI licensed the right to develop, manufacture and commercialize a select number of novel clinical stage drug candidates from Johnson & Johnson. The total number of drugs – and the specific names of the drugs – appears to be kept secret.
Instead of just trying to discover a new compound from scratch, BenevolentAI uses their AI system to try to find new potential uses for existing drug candidates. While BenevolentAI doesn’t have any succinct videos on their own YouTube channel to summarize their value proposition, the video below (from CB Insights) feature Benevolent’s James Chandler explaining their company and mission in a nutshell:
There are numerous drug candidates that went through significant early testing for safety but were abandoned when they were found to be ineffective for the disease they were designed to treat. By using AI to find other disease these drug candidates might instead work for, BenevolentAI can theoretically dramatically shorten the process, since all the preliminary safety testing was already done. This would allow them to go directly to phase II clinical trials.
It appears that BenevolentAI’s recent phase 2b trial for a drug to treat sleepiness in Parkinson’s patients is one of the first uses of this agreement. This seems to be the first test trial in the J&J / BenevolentAI partnership, and results aren’t expect until August 2018.
Conclusion
The use of artificial intelligence in drug discovery is a relatively new application of the technology. It is going to be very difficult for any outside observer to point to definitive proof that AI is clearly improving drug discovery. This is true for a number of reasons:
- Long development time – It takes around a decade for a drug candidate to go from discovery to market and even a single stage of clinical trials can easy take a year.
- High failure rate – Even with AI assistance we can expect most companies drug candidates to fail. It would not be unexpected or a proof of a bad algorithm if an AI drug companies first several attempts failed. Success for AI in drug discovery would be just a slightly lower rate of failure and that requires a large dataset to draw conclusions from.
- Trade secrets – Most companies are very protective of their trade secrets and unlikely to the exact degree to which different proprietary tools were used in their R&D.
At this point one of the best indication that AI drug discovery platforms have the potential to have a real impact on the pharmaceutical industry is how the biggest pharmaceutical companies are reacting to it. While they have not yet engaged in a full on embrace of these platforms via large scale acquisitions, over just the past year we have seen many of the big drug makers seriously examine the potential of the technology via numerous tests, official collaborations of different sizes, and licensing agreements.
The big drug makers have begun exploring how AI might be used at all levels of the drug discovery process from the creation of new compounds, to finding potential combinations, determining how to develop personalized medicine, and discovering possibly new uses for previously tested drug candidates.
Theoretically, it is easy to understand why investors and drug makers believe AI could eventually play a big role. It can be argued that the pharmaceutical industry has already discovered much of the low hanging fruit — simple, easy-to-find drugs that are safe and effective for everyone. Many of the future treatments will likely take the form of drug combinations or treatments that only work for people with specific genes. Finding these treatments can require cross checking massive data sources for possible interactions, the type of tasks that can be difficult for people but relatively easy for computers.
Header image credit: Prescription Assistance 123