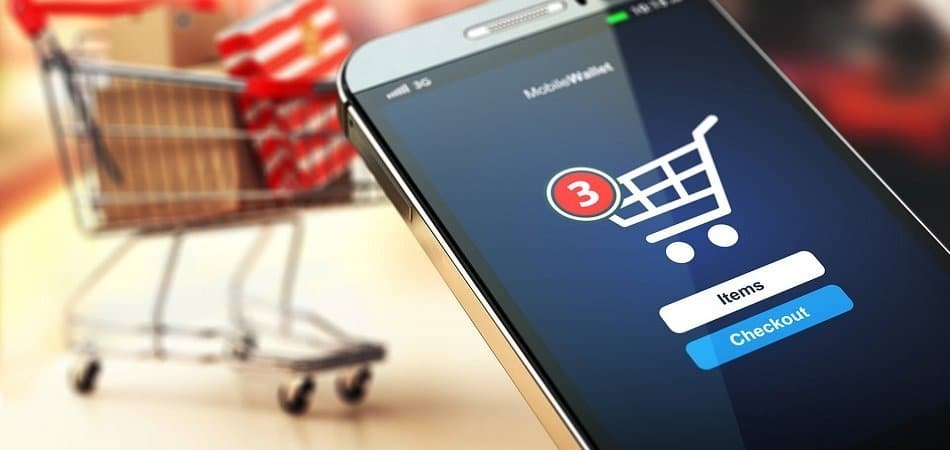
Episode Summary: Artificial intelligence plays a role in the future of retail in terms of a deeper understanding of customers going beyond intuition. This week, we speak with Pedro Alves, CEO of a company called Ople, based in San Francisco. Alves was previously the Head of Data Science at a number of companies in addition to being Director of Data Science at Sentient Technologies, one of the best known AI firms in the Bay Area. Sentient has raised upwards of $200 million.
We talk with Pedro about the future of retail, the future of understanding customers with artificial intelligence. We ask under what circumstances would a retailer need to go beyond intuition in order to inform their understanding and their ability to influence the actions of their customers or their users?
In addition to that, Alves talks with us about what has to happen to AI as a technology to become more accessible and within reach of existing enterprises. Knowing now all the points of friction for bringing AI into an existing business, he talks about the transition points that he thinks are going to have to happen over the course of the years ahead in order to make these technologies more accessible to companies.
Subscribe to our AI in Industry Podcast with your favorite podcast service:
Guest: Pedro Alves, founder and CEO – Ople.ai
Expertise: enterprise adoption of AI, machine learning, data science
Brief Recognition: Before founding Ople, ALves was Director of Data Science at Sentient Technologies, Chief Data Scientist at Banjo, and a senior data scientist at Tagged. In addition, Alves holds a PhD in Computational Biology from Yale.
Interview Highlights
(03:00) How do we find groups of maybe better buyers, or worse buyers, or people with similar behavior?
Pedro Alves: Yeah. When you’re thinking of traditional models that you’re building, you’re thinking of first of all supervised [learning]. With that, there’s this implication of you’re thinking the model is going to understand how to predict something, how to classify something. If you’re doing customers in retail, and you’re saying, “Will this person purchase item X, yes or no?” The model doesn’t really need to understand the customer; it just needs to predict. It just needs to be accurate at predicting what’s going to happen.
What we’re talking about here is something different. The idea is instead of training the model on making a prediction, what if we train the model on trying to understand the intuitive choices that an expert would make when looking at these customers? What I mean by that is take, for example, you sell shoes online. And you have people in your company that understand your customer base, they understand your products, they’re not technical at all, but they just really understand who buys, why they buy, and when they buy.
A lot of the times, you can ask them, “Hey, look. Tell me about these customers.” You can show them a list of 10 customers and say, “How would you pair these customers up? Or how would you find customers that are similar?” They would say, “Okay. Customer X and customer Z are close to each other.” Then if you ask them, “Why? What is that? Why is customer X closer to Z than any other two in this big list?” They might not know exactly why, they might not be able to say, “Okay. It’s because I look at their age, and multiplied by the,” you know, whatever, “How much money they spent last month-”
So what if we could just say, “Okay, we don’t know what it is, and you don’t know what it is, but you have that gut intuition, you are the expert.” What if we could train a model to basically try to understand and figure out what that intuition is? Once that model then understands that intuition, that model can start making those same choices, that model can start doing two things actually. It can start making those selections the same way the expert would say, “Oh yeah, X is really close to Z.” But, because the model’s job is to understand the intuition, it not only can say X is close to Z like the expert can, but it can actually show you why or how those customers are similar.
So, you get these two benefits of this type of model…The most basic form is just saying, “Show me similar customers.” I mean, right there there’s the value of creating proper A/B tests. Every time I talk to companies in retail, that’s a major problem for them is how do you create proper A/B tests that are valuable. Because everything they do, improving the website, testing machine learning models, promotions, everything depends on proper A/B testing. So if the A/B testing is not done properly, then they’re not going to make the right choices, which could swing their business up or down in a significant way.
(06:45) How does this go down?
PA: What it looks like is, you’ll have people that are not technical. People that are technical in not data science, machine learning, but technical in their field whether it’s selling insurance, or selling shoes or selling cars. They’re going to be having a conversation with the AI. That’s literally what’s happening. To the person, it’s a pretty easy-to-have conversation because it’s abstracted and pretty meta. To the machine, it’s much more complicated. But again, it abstracts all those layers. The type of back and forth that a person is going to have with the machine is going to be something that I wouldn’t even have to teach you anything, I could sit you down in front of our UI and say, “Here you go.”
What I think is changing is not, “Oh, you’re not going to need data scientists anymore.” I think what’s going to change is where the data scientists are going to spend their time is going to shift, and the overall picture of what a data scientist is, like their background, their knowledge base, is also going to shift. But data scientists for sure are going to endure the next decade. It’s just there’s going to be a shift in what it means to be a data scientist.
(10:00) I wanted to chat about some instances where maybe you’ve seen where companies have needed this kind of clustering and understanding in the real world.
PA: I would explain it as an embedding, but if that’s too technical of a term, it’s a mathematical representation of people, of customers, that can be interpreted in human language. Because in the way of training, we’re having that conversation with a person, there’s going to be a translation learned between the math behind the representation of the customers and the language that people use. So that when the user sees it, it can actually be expressed in something that a human would understand. To answer your question, I think A/B test was an obvious way of using this that a couple customers had. I don’t think it actually is going to end up being the biggest reason this is sold, and the biggest value that this can bring.
I think the biggest selling point for this is if you can have this understanding, this representation of your customers, you can basically either look at where the customers are, but also you can look at where they’re going. So you can say, “Okay. Map all my customers.” And then I can understand, “All right. In this map of customers. Where are my customers that spend a lot of money at Christmas time? Oh, here they are.” Then you can say, “Well. If I’m going to do a promotion at Christmas,” you might want to say, “They’re going to spend at Christmas no matter what, I’m going to spend my efforts in people that usually are not, and have a chance of spending on Christmas if I actually do something for them.” Or, whatever it is you’re doing.
If you also understand the movement though, imagine this is not like a static map, but customers move and shift, they change their buying behavior in a website, they change what kind of customer they are. If you can start noticing that pattern, that migratory pattern of customers, those herds moving along the map, you can literally ask the software and say, “Okay, this group of people, they moved from here to here, and that means they’re going to from not spending a lot to spending a lot. And this group of people they go from medium spend to just dropping off completely. Tell me, what’s different between these groups?”When a person changes direction, what happened? What trait changed? “How can I get these people that I think are going to move in this direction, which is stop using my website, into this direction, which is people that spend twice as much more?” Once you start having that interaction, you just can have total understand and control, not complete control, because people are still people and they’re going to do whatever they want, but [that] can help influence where they’re going.
(17:30) What aspects of AI in terms of customer understanding will be just part of retail [in 10 years]?
PA: I think there are two aspects there. One is the growth of proper usage of AI. Right now, the techniques are there, and they’re wonderful. If you do it right, great. We both know in the majority of cases, companies are struggling. They’re not getting their ROI whether it’s because they didn’t hire the right people or because they, you know, there’s like a million reasons, we’d be talking here for two hours.
So I think the first step in that 10-year journey is making AI something that’s easy, cheap, and ubiquitous. I think as it progresses from easy, then it becomes cheap because once it’s easy, a lot of people are going to be doing it.
Once it’s cheap, every company is going to get it. Finally, which is not there yet, once every company gets it, it’s ubiquitous. So I think the first step is that. It’s, for example, this use case that you talked about. Getting every company, no matter how big or small, no matter what ability they have to hire that top talent. Forget about all that. That no longer becomes an issue. Every company can get it and use it properly. Once that happens, then the next thing that’s going to happen is what I’m really excited about. It’s really what I don’t know. As a species we’re very, very bad at predicting things very far out.
[Educated people once said], “The world will never need more than eight computers.” And after the airplane was invented, people said, “This has no military applications.” These were serious predictions from serious people. That’s where we are with AI today. I really believe that. As much as we like to think we know where AI is going and we like to make these predictions, I think we’re that level of infancy. Because right now we’re blinded by the cost and the expense and the difficulty of it. So it puts blinders on our eyes, and it doesn’t let us really let our imagination fly.
What I’m excited about is there’s three categories of problems that companies have right now. Category one are the difficult problems. The ones that they’re struggling to solve, I mean, I’m talking about AI problems, the difficult problems that they’re trying to solve with the people they have or hiring people to solve. The second class are the impossible ones, the ones that some company says, “We wish we could do stuff with computer vision, but there’s no way we can hire a PhD in Computer Vision or deep learning.” So we put that into the impossible bucket, we’re not even going to try to do it.
Then there’s the third category, which is the unimaginable ones. Which are the problems that only when the difficult and impossible become easy are people going to start thinking, “Wait a minute, we could put AI here, here, here, here, here.” In the many places that we don’t even think about yet. That’s where 10 years from now retail, for example, is going to be. It’s going to be using AI in places that I can’t even tell you right now.
(21:00) Easy, cheap, ubiquitous: what are the biggest barriers to “easy?”
PA: I think “easy” has two big facets. One of them is time, the other is expertise. Because somebody was asking me the other day, what makes it hard? I would say washing a car doesn’t take expertise, you don’t have to go to school really to wash your car. But to wash a car really well, and polish it, and wax it, still might take like five hours. That’s not easy, that’s still kind of hard. So the time component is one, easy should be, you can wrap up a project in a couple weeks, not three or four months.
Subscribe to our AI in Industry Podcast with your favorite podcast service:
Header Image Credit: Marketing Land