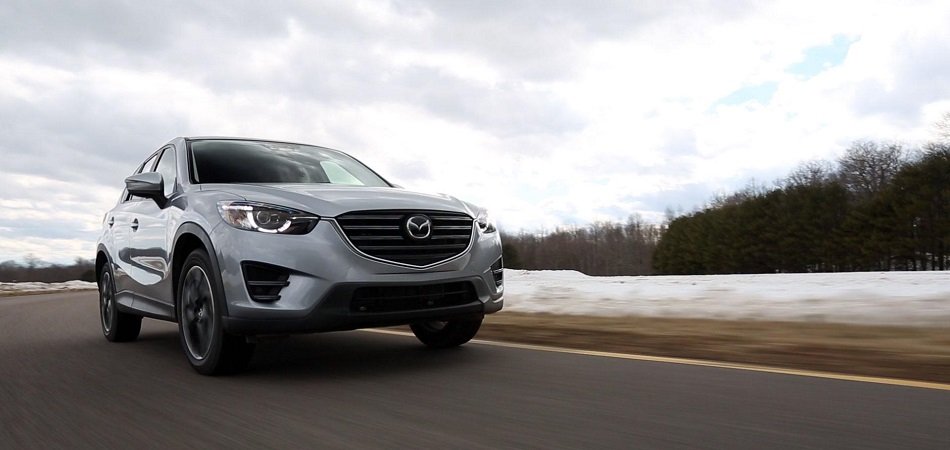
Statista estimated that the market for partially autonomous cars will be at $36 billion by 2025, and the market for fully autonomous cars will be at $6 billion. We researched the automotive space to discover how and where AI might be driving business value for companies in the auto industry. The companies in this report all claim to offer one of two sectors of the industry:
- Auto Insurance
- Autonomous Driving
What Business Leaders in the Automotive Industry Should Know
AI applications for the automotive industry seem to fall into one of two sectors: insurance and self-driving car services. Progressive worked with Microsoft Azure to build a chatbot that we can presume uses natural language processing. There are numerous other insurers that claim to offer machine learning chatbots, but we believe Progressive’s Flo chatbot has the highest likelihood of legitimately using AI.
Progressive may also be poised to launch further AI initiatives in the future, a result of collecting large volumes of customer data from their Snapshot telematics program, which allows drivers to install a sensor in their car that sends information on how they are driving to Progressive. They could in the future use that data to train a predictive analytics software that may allow them to offer personalized insurance policies to its customers.
Tractable is the only startup discussed in this report, but we believe them worthy of there place here. The company offers a machine learning software for the insurance claims process. They claim auto insurers can integrate their software into their existing workflows, likely allowing customers to upload pictures of their damaged vehicles to the software. The software would then estimate the customer’s payout based on the damage.
They employ roughly 80 employees, raised $34 million, and were founded in London in 2014. Their founding date is the first signal to us that Tractable is likely genuine in claiming that it offers machine learning software. Many older companies that were founded prior to the late 2000s might claim to offer AI solutions for various industries, but it’s very difficult to transition from a company that doesn’t do AI to one that does; attracting data science talent is a real challenge for older firms, and data scientists are necessary for building machine learning models. Even a company like Progressive likely struggles with hiring and retaining data science talent that would prefer to work at companies whose value propositions are predicated on AI, such as Google, Amazon, or Facebook.
Tractable, on the other hand, was founded by researchers with advanced degrees in not just computer science, but machine learning. Although many of their artificial intelligence “analysts” and “specialists,” as the company calls them, seem to be more insurance experts than people that live and breathe data science, the company’s lead researcher, Ken Chatfield, was the company’s first employee, and he holds a PhD in Computer Vision and Machine Learning from Oxford. The company’s co-founder, mentioned later in the article, also holds an MPhil in Computer Science with a machine learning distinction from the University of Cambridge.
It’s likely that these executives lead the AI efforts at the company and might be working with their AI “specialists,” who seem to be subject matter experts for the purposes of data labeling, to build machine learning software for their two niches: auto and disaster insurance. In addition, they are backed by NYC-based Insight Venture Partners. This bodes well for the company and for auto insurers looking to work with them.
When it comes to self-driving cars, one doesn’t need to stray too far from the usual players in the AI world. Google and NVIDIA seem to be at the forefront of machine vision technology for self-driving cars. There is little question as to the legitimacy of the AI behind these companies’ software; a car isn’t going to drive on its own without some kind of machine learning behind its system, and companies like Google and NVIDIA are global leaders in artificial intelligence.
That isn’t to say business leaders should trust everything these companies say about what their AI can do, but it’s safe to say that it’s doing something, even if not what they claim.
Google-owned Waymo plans on unveiling a fleet of self-driving cars for a ride-hailing service in the coming year. NVIDIA claims to offer an AI-based assisted driving software in addition to software for fully autonomous vehicles. The machine vision behind the software can reportedly detect if a driver is distracted and alert them when necessary. For example, if the driver is falling asleep at the wheel, NVIDIA’S software can reportedly sound an alarm in the car to wake them up.
Although this report does not cover machine learning for manufacturing cars and other vehicles, interested readers may want to read our general report on machine learning in manufacturing. Many of the technologies in that report are transferable to car part assembly lines.
Auto Insurance
Progressive
Progressive offers a chatbot called Flo, which the company claims can help their customers file claims, view and change payment dates, and get quotes for their auto insurance. The chatbot uses natural language processing and a cloud-based API that pulls data from social media responses and training data. We previously covered Progressive’s chatbot in our report, Chatbots for Insurance.
We can infer the machine learning model behind the software was trained on hundreds of thousands of customer support tickets and their responses from customer service agents involving filing claims, billing, and deductible rates. This text data would have been labeled as claims-related, billing-related, deductible-related, or related to other categories Progressive chose. The labeled text data would then be run through the software’s machine learning algorithm. This would have trained the algorithm to discern the chains of text that a human might interpret as a claims-, billing-, or deductible-related question as displayed in a text message.
A customer could then message the chatbot, and the algorithm behind the software would then be able to categorize the ticket as claims, billing, or deductible-related. The chatbot likely then comes up with a confidence interval on how likely it correctly categorized the ticket. It would also be programmed to take two actions depending on that confidence interval. Above a certain percentage, the chatbot would send an appropriate response to the user. Under a certain percentage, the chatbot would route the ticket to a human agent for review.
Progressive does not have a demonstration video showing how Flo works. Also, Progressive uses Flo internally, and so there are no available case studies for the software.
Progressive claims Microsoft Azure helped them build their chatbot, which emulates their popular TV mascot in addition to understanding customer questions and responding quickly. Flo uses simple language, but adds in wit where appropriate, which may engage customers and tie into Progressive’s marketing. Progressive built the Flo Chatbot using Microsoft Azure Bot Service and LUIS. The company found that the software facilitates updating the bot and its responses without needing to write complex code.
The APIs also provide the ability for the chatbot to expand its database with each customer interaction, so the quality of responses improves over time. According to Microsoft, Progressive updated Flo’s models over 75 times in the first four months of activity to help the chatbot continue to improve itself and customer interactions.
Tractable
Tractable offers a software which it claims can help automotive insurance businesses automate the claims process using machine vision.
Tractable claims insurance agents can upload images related to the claim, such as photos of a damaged car, along with an estimate of how much money the client should receive based on the car’s damage. The software then compares the uploaded image to every image of a damaged car that has been uploaded previously, along with the amount of money paid out to the owners of each car in the images.
We can infer the machine learning model behind the software was trained on thousands of images showing cars with varying degrees of damage from various angles and in various lighting conditions. These images would have been labeled according to how much damage the pictured car sustained and which parts of the car were damaged. These labeled images would then be run through the software’s machine learning algorithm. This would have trained the algorithm to discern the sequences and patterns of 1’s and 0’s that, to the human eye, form the image of a damaged car as displayed in a photograph.
The user could then upload an image of a damaged car that is not labeled into Tractable’s software. The algorithm behind the software would then be able to determine how much a car is damaged and on which parts, and how much the owner of the car should be paid. The system then shows a human employee a text description of the damage and the amount of money to pay the owner.
Below is a short video of Adrien Cohen, co-founder and CCO of Tractable, demonstrating how Tractable’s software works. The demonstration can be found between 2:18 and 9:45.
Tractable does not list any major companies as clients, but they have raised $34.9M and are backed by Ignition Partners, Insight Venture Partners, and Zetta Venture Partners.
Razvan Ranca is CTO at Tractable. He holds an MPhil in Computer Science with a machine learning distinction from the University of Cambridge.
Autonomous Driving
Waymo
Waymo, a self-driving car company owned by Google, claims its self-driving car technology will soon be available to the public. Waymo claims its software automates driving using a combination of computer vision components such as LiDAR, radar, and hi-res cameras.
We can infer the machine learning model behind the software was trained on hundreds of thousands of miles of test driving in busy city streets. This would introduce the model to live footage of everything a driver would need to pay attention to, such as other cars, traffic lights, street signs, and pedestrians. Each of these entities would be labeled under those categories. This labeled footage would then be run through the software’s machine learning algorithm. This would have trained the algorithm to discern the sequences and patterns of 1’s and 0’s that, to the human eye, form the image of a car, traffic light, street sign, or pedestrian as displayed in the live footage.
Google could then send a car using Waymo on a test drive, which would give it brand new live footage that is not labeled. The algorithm behind the software would then be able to detect objects around it and determine whether they are other cars, traffic lights, street signs, or pedestrians. The system then drives the car with the ability to account for traffic signals and people on the road.
Below is a short 3-minute video demonstrating how Waymo works:
Waymo has partnered with WalMart, AutoNation, Avis, DDR Corp, and Element Hotel to provide autonomous rides to customers for a limited time in the Phoenix Area.
Dimitri Dolgov is CTO and VP of Engineering at Waymo. He holds a PhD in Artificial Intelligence from the University of Michigan. Previously, Dolgov served as a Senior Research Scientist at Toyota Research Institute.
NVIDIA
NVIDIA offers a software called NVIDIA Drive, which it claims can help car manufacturers create automated driving systems using machine vision. The NVIDIA Drive software platform consists of Drive AV for path planning and object perception and Drive IX for creating an AI driving assistant.
To create such a system would require training the machine learning model on large amounts of driving data, so it is likely that the software has been tested using multiple types of cars at NVIDIA.
We can infer the machine learning model behind the software was trained on thousands of hours of driving footage showing objects on the road such as traffic lights and pedestrians, in the case of Drive AV. This footage would have had each object within it labeled. This labeled footage would then be run through the software’s machine learning algorithm. This would have trained the algorithm to discern the sequences and patterns of 1’s and 0’s that, to the human eye, form the image of a traffic light or pedestrian as displayed in live footage while driving.
However, Drive IX would have been trained on footage showing the actions of drivers during a trip. For example, actions unrelated to driving, such as texting, looking through a bag, or turning one’s head to talk to someone would be labeled as distracted driving. This labeled footage would then be run through the software’s machine learning algorithm. This would have trained the algorithm to discern the sequences and patterns of 1’s and 0’s that, to the human eye, form the image of a distracted driver as displayed in the training footage.
The developer could then drive with either one of these software, exposing Drive AV and Drive IX to live driving footage that is not labeled. The algorithm behind Drive AV would then be able to detect objects and people around the car while driving. The system then drives the car with consideration of the car’s surroundings. The algorithm for Drive IX would be able to discern between when the driver is paying attention to the road and when they are distracted. The system would then provide an audio alert of distracted driving to encourage the driver to pay attention.
Below is a short 1-minute video demonstrating how Drive IX alerts for distracted and drowsy driving:
NVIDIA’s software is both new and only legal for public use in select areas, and so there are no case studies involving client companies available.
NVIDIA also lists Tesla as a client for Nvidia Drive. The companies have partnered to integrate NVIDIA Drive into all Tesla vehicles to provide fully automated driving to Tesla owners.
Header Image Credit: Consumer Reports