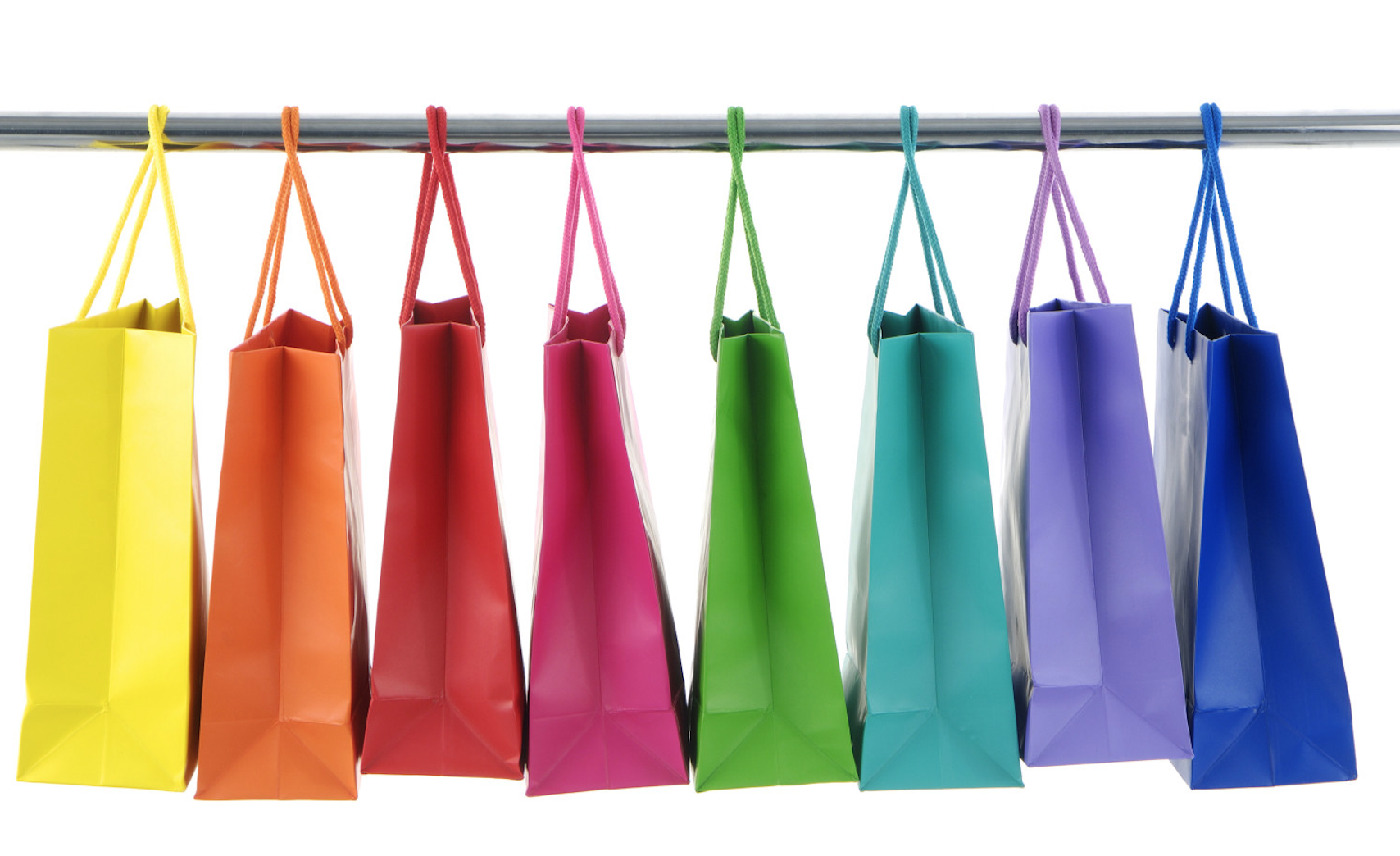
When managers and leaders think about machine learning in retail, they often imagine helpful in-store robots, automated processes like checkout or stocking shelves, or conversational agents that suggest products and answer questions for customers. While these applications should be considered as disruptive factors for retail’s future, it’s unlikely that these factors will be the first wave of AI’s impact on the sector.
Our own research on AI in retail suggests that the initial implications of artificial intelligence and machine learning will involve much less physical automation and direct replacement of human workers (shelf stockers, checkout clerks, etc), and much more augmentation of the current retail experience with data and decision-making.
The Online-to-Offline Trend for Machine Learning in Retail
This first phase of AI in the retail sector can be thought of a process of “bringing the online, offline.” The online shopping world generally has the advantage in optimizing its analytics, marketing, product placement, and product stocking. This is not only because eCommerce companies tend to be more tech-savvy – almost by definition, a pure-play eCommerce company is younger than most brick-and-mortar retailers (the internet hasn’t been around for very long in the history of shopping!).
Rather, online business have an advantage because their “stores” (read: websites and apps) are instrumented to detect every click, every scroll, and every single user behavior. This information can be fed into data processes that help predict (in real time) the success of online advertising efforts, and the
In this article we’ll break down the online-to-offline trend into the following four sections:
- Application Area 1: Stocking and inventory
- Application Area 2: Behavioral tracking – marketing and product placement
- Application Area 3: Behavioral tracking – theft
- The shift towards efficiency and what retails / suppliers need to do about it
While this certainly won’t be an expose of all of the possible applications of AI for retailers, we believe that these three categories will account for a bulk of the tangible value that AI will create in this sector in the next 2-4 years.
1 – Machine Learning for Retail Stocking and Inventory
Optimizing inventory is by no means a problem exclusive to retail – but it is an inescapable consideration for businesses predicated on holding physical items to be sold in-person to customers (not that eCommerce doesn’t have it’s hassles, but optimizing per location for a retail chain is often a logistics concern that eCommerce players don’t have to deal with).
What may be possible in the near-term:
- Retailers will be able to use purchase data (real time and historical, online and offline) to predict inventory needs in real time (based on the season, the day of the week, activity at other area stores, eCommerce activity from customers in that geo area, etc…)
- This information may prompt a daily dashboard of suggested orders to a purchasing manager, or some AI systems may be approved to make small orders automatically without requiring human approval
- Using machine vision for cameras (either installed throughout the building, or carried by employees), inventory systems of the future will be able to generate accurate, real-time estimates of all products in a given store.
- Such a system could notify store managers of unusual patterns of inventory data, such as suspected theft, or an unusual uptick in sales of a particular product that may warrant a faster re-order than might have been previously planned
(Stocking and inventory are broad topics that expend beyond retail, for more insight on this domain specifically, see our recent article on “cognitive procurement”, written by procurement expert Ed Zagorin.)
2 – Behavioral Tracking – Marketing and Product Placement
If inventory optimization is about cutting losses, marketing and product placement is about driving revenue. As in-store environments become full of sensors and adept at tracking valuable information, stores will be able to encourage sales and cart value in many new ways.
Our own research into the applications of machine learning in marketing seems to bode well for retail applications. In our interviews with 51 different AI / marketing executives, we found that the AI marketing vendors we spoke with were overwhelmingly optimistic about retail and eCommerce as sectors ripe for applying AI to marketing. Below is a graphic that demonstrates the sectors that these AI marketing vendors were targeting (i.e. selling to) from our recent consensus:
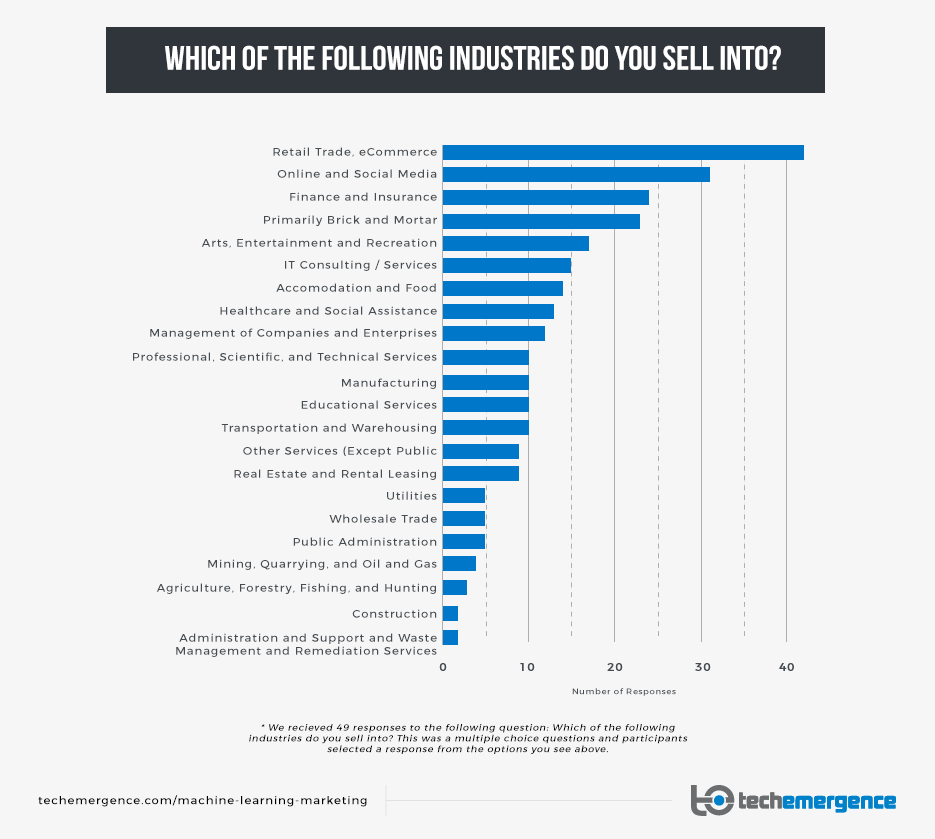
What may be possible in the near-term:
- Cameras detect the walking patterns and the direction of the gaze of its customers. This information could be used to analyze the interest in various products, to restructure store layouts, or to test new products in locations that not only get the most foot traffic, but also receive a high percentage of visual attention from shoppers.
- Cameras in stores could pick up on demographic differences between retail locations (or differences in days of week, or season), and use this information to adjust product placements (for example, if elderly people shop much more on weekdays, then products that sell well to those groups may be placed or promoted more prominently on weekdays).
- AI systems may become capable of not only detecting patterns in data (financial, marketing, visual, etc), but also suggest marketing actions or promotions to the store managers. For example, if a certain product is selling much better than competitive brands, a system may suggest placing it more prominently, or not re-ordering the alternatives. Or – if a product isn’t selling sell, a system may have a suggestion for a discount and placement that will get the inventory offhand and clear space for better selling products.
In most retail stores today, cameras are used for little more than security. These simple cameras require a human being to watch a screen in real-time in order to take action. Most camera systems in the retail environment of the future will be used for computer vision – delivering value to businesses without humans needing to watch the footage themselves.
Detecting suspicious behavior, gathering broad information about demographics and behavior patterns in-store… all of this will be possible via an AI systems – with reasonably limited human interaction. Granted, any robust AI application requires setup / integration, as well as monitoring and tooling (done by someone with strong AI experience – and such employees are not cheap) – but this beats the alternative to having thousands of interns with clipboards writing down the foot paths and eye gazes of all of a store’s customers in real time (which is clearly impossible).
There are a number of startups already focused on marketing analytics from video data. Aurora, for example, is a product from San Jose-based RetailNext. The device aims to add richness to customer traffic data by using machine vision to garner data about demographics and customer engagement in real time:
We imagine that large retail chains will be the first to implement these technologies, as they have the biggest technology budgets, and are potentially most poised to see an ROI with machine vision if they can transfer important marketing lessons across multiple stores to improve traffic and sales.
3 – Behavioral Tracking – Theft
Theft will become increasingly difficult as physical retail locations are instrumented for extracting patterns and meaning. If the isles of a store are monitored by cameras that detect if someone looks at, picks up, or interacts with an item – then detecting someone putting that same product in their shirt or in their backpack is not a significant step up.
What may be possible in the near-term:
- Detection of any item that is hidden or concealed by the individual in the store
- Detection of suspicious behavior (based on training data from previous stores, garnering a sense of what kind of behavior patterns are common among thieves)
- Detection of “sweethearting” – when checkout clerks skip over items or avoid scanning them (possibly to do a favor to a friend or familiar customer)
- Detection of individuals deemed statistically likely to steal (based on training data of previous confirmed thieves inside the same store)
The latter two applications are likely to spawn criticism (and maybe rightfully so), but they’re likely to be employed by any retail who is technically capable thereof.
Security personnel or managers may receive messages from an AI system – prompting that employee to watch the video clip to ensure the behavior themselves before confronting the individual in the store. At a certain level of consistency and performance, store employees may only need to refer to the footage as a backup, and may be confident in approaching an individual in-store simply from a prompt from the AI system.
It is also likely that systems of this kind may employ personal identifiers such as facial recognition, gait analysis, and other factors that can inform law enforcement if the individual escapes the store after stealing (or attempting to steal).
Cleverly-named and Cambridge, MA-based StopLift is example of a theft-prevention vendor that offers a machine vision solution already. In the video below, StopLift demonstrates that various scenarios of theft at checkout – and how their system detects these incidents:
Because the ROI for theft prevention is so strong, we suspect that these technologies will have faster adoption in the near-term than more advantaged marketing applications of machine vision. If a store owner or manager knows their average monthly loss to theft (say, $8,000), then they can potentially get a sense of a theft prevention system’s ROI in a matter of months (by simply reporting to ensure that the system costs less than the theft it prevents).
Humans tend to be motivated more by preventing loss than making gains, we suspect that this “defensive” mindset will also promote theft-prevention adoption before the general adoption of machine vision in marketing or product placement.
The Great Shift Towards Efficiency – and How Retailers and Suppliers can Prepare for It
Smart retail executives are always interested in understanding the realistic “possibility space” of a technology’s applications – and that’s what we’ve tried to do in this article. What makes this information more valuable is an understanding of what it means for them. If we take a moment to conclude and step back from the use-cases and companies we’ve mentioned – what is the overarching take-away message that retail executives should consider when it comes to AI?
We think that the most compelling distillation of the key lesson is this:
Offline retailers should prepare to collect and understand more data to improve efficiencies in inventory, placement and in marketing.
The biggest retailers in the world will likely be the first-movers on these technologies for a number of reasons. They are most likely to have the technology budget, the data science-savvy staff, and the raw ability to collect data at scale from many locations (and possibly from a large eCommerce sales operation as well). These retailers will be “instrumenting” stores to be more like eCommerce sites – making decisions off
What does this mean for how retail is run? In general, it means that retail is likely to make leaps in its efficiency. That means that factors other than data will have less and less impact on the decision-making of retailers, and this has implications for vendors and suppliers who sell into the retail space. Review the following factors:
- Trusted, established brand names: When a retailer knows it sells more of a less well-known brand, and gets the same or lower refunds, it may well drop a well-known brand in favor of its economic incentives
- Close, long-term sales relationships: A smooth salesperson who sends Christmas cards to the purchasing manager every year may not be able to win business with a great “business relationship” if the tangible ROI isn’t there for the retailer
- Intuition or “gut feeling”: Purchasing managers (or marketers, or store managers in charge of store layout, etc…) who base their plans primarily by their “gut” may be at a disadvantage if competitors are gradually cutting losses and piling in more returns quarter-over-quarter with better, more nuanced financial decision-making
If I were a vendor (i.e. a business that sells, say, window dressings or display tables to retailers) or a supplier (i.e. a brand that sells its goods to retailers so that the retailers can sell to customers at a profit)… and my business was heavily predicated on any of the factors just listed, I would be taking actions to change my strategy to align with a more data-centric worldview.
Concluding Thoughts – and Next Steps for Retailers / Suppliers
While there’s no need to panic and consider a swift overhaul in how retail is done (these changes won’t happen overnight, and there are many applications still to be fleshed out), leaders in the retail sector should prepare for a future where decisions are made more and more on hard data.
In-store robots, augmented reality, chatbots… there are a lot of potential future applications that will alter the retail experience – but we don’t see many technologies likely to physically alter retail in the next 5 years (Note: we’ve covered some of the current use-cases of chatbots today, and the most prominent among them seem to be more geared toward customer service and eCommerce, rather than in-store retail).
Rather, we see a general shift towards “instrumenting” retail stores to make real-time decisions based on data, relying less on “how it’s always been done” and more on constantly adjusting the retail environment to cut losses and produce gains.
By our estimation, the most fruitful activities for today’s retail leaders would be:
- Keep a close look at Amazon and the biggest retailers in the world – particularly those in your industry (i.e. fashion, home goods, sports, etc). The use-cases that gain traction (and deliver value) for the biggest players are most likely to be the applications that define innovation for smaller companies.
- Ask yourself: Are our stores becoming more and more like websites? There is lurking danger for retailers that don’t improve their ability to track, test, and handle data (from marketing, inventory, etc…) quickly. For most retailers, “stepping up” the IT infrastructure and the data science chops of an organization would probably be better to do now than later.
Header image credit: Rodo Hotel