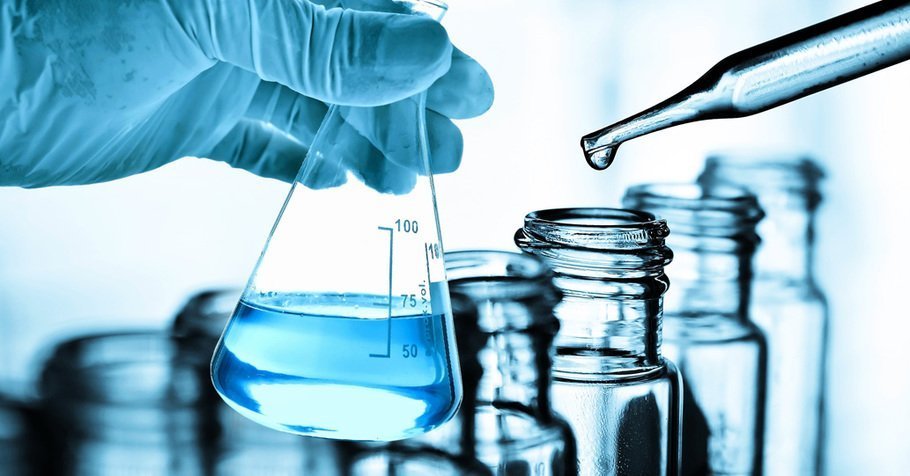
The clinical trial is a foundational pillar of the pharmaceutical drug discovery process. Essentially, clinical trials are research studies which seek to determine if a medical treatment or device is safe and effective for humans. While the pharmaceutical drug industry has experienced some fluctuations it remains a profitable market. Global prescription drug expenditures are estimated to reach nearly $1.5 trillion by 2021 according to Quintiles IMS Holding.
However, major challenges facing researchers structuring clinical trials are high costs and have low rates of success. According to the U.S. Food and Drug Administration, roughly 1 in 10 of drugs tested in human subjects receive FDA approval and millions of dollars are invested in the research process.
In this report, we set out to determine the answers to the following important questions:
- What types of AI applications are emerging to improve clinical trial efficiency and optimization?
- How is the healthcare market implementing these AI applications?
The majority of AI use-cases and emerging technologies for clinical trials appear to center around three primary applications:
- Patient Recruitment: Companies are developing software platforms to better match patients to clinical trials based on specified criteria.
- Clinical Trial Design: Companies are developing machine learning algorithms to help researchers manage clinical trial workflows.
- Clinical Trial Optimization: Companies are developing machine learning models to predict which patients are at risk of dropping out of clinical trials to prevent threats to trial validity.
Patient Recruitment
Antidote
Founded in 2010 with headquarters in London, England and offices in New York, NY and Carmel, Indiana, Antidote claims that it uses machine learning to connect patients to medical research studies through its clinical trial matching platform.
Using algorithms trained on high volumes of patient eligibility criteria for clinical trials, thousands of open trials are searched by the Antidote Match search engine based on user responses to a questionnaire.
Upon accessing Antidote’s website, patients searching for clinical trials are prompted to provide responses to a series of questions about their health such as “for what condition are you looking to find a clinical trial?” and about their location to be matched to opportunities nearby.
In the 2 minute video below, CEO and Founder Pablo Graiver and Antidote staff discuss the mission of the company and its potential value to the drug discovery process.
According to a case study, a clinical trial relating to Alzheimer’s disease required 10,000 participants in 2 months. Antidote claims that through its platform and partnerships, it “delivered 8,000 of the required 10,000 referrals in less than 2 months [and its referrals] were seven times more likely to complete the required follow-up than referrals from other sources.”
Through Antidote’s Connect Network, the company has expanded its platform access to health organizations by allowing the Match search engine to be embedded into websites. As a result, the company claims that its platform reaches over millions of patients through more than 180 online communities.
Companies partnering with Antidote include the National Kidney Foundation, Lupus Research Alliance, and Healthline.
Staff members with machine learning experience include Tom Reeve, a London-based Data Engineer trained at the University of Essex. To date, Antidote has reportedly raised $28.9 million in funding and is backed by the Merck Global Health Innovation Fund.
Deep 6 AI
Founded in 2015 and based in Pasadena, California, Deep 6 AI claims that it uses natural language processing (NLP) to better match patients to clinical trials.
Deep 6’s algorithms are trained on patient medical data and thousands of clinical data points, such as symptoms, diagnoses, and test results, and they are extracted from patient health records. The company claims that it can execute the extraction process “while masking protected health information.”
Next, the extracted clinical data points are aggregated to develop a clinical profile. The clinical profiles are used to find and compare populations, cohorts, and individual patients meeting user search criteria.
In the 2-minute video below, CEO and co-founder Wout Brusselaers provides an overview of the company’s goals to use its platform to help accelerate access to drug development.
In a case study, the company describes how it assisted Cedars-Sinai Heart Institute in improving its ability to identify eligible patients for a research study. Prior to implementation, the research team required an average of six months to identify two eligible patients for a clinical trial requiring a total of eight patients.
Using the Deep 6 AI platform, Cedar Sinai was reportedly able to identify 16 patients meeting the eligibility criteria for the trial in an under an hour.
B. Kerim Tshimanga is a Data Scientist for Deep 6 AI with machine learning and deep learning expertise. He received his degree in Physics from the University of California, Santa Barbara.
Clinical Trial Design
Trials.AI
Founded in 2015 and based in San Diego, California, Trials.AI claims it leverages NLP to help researchers more efficiently manage clinical trial workflows.
The algorithms driving Trials.AI’s cloud-based Study Optimizer platform are reportedly trained on “billions of data points from past clinical trials, medical journals, and real-world sources” to identify risk factors and provide recommendations for clinical trial optimization.
For example, the process begins with a user uploading his or her research protocol documents in Microsoft Word or PDF to the platform. Risk factors are identified by analyzing the protocol text, and any potential barriers to a successful trial. such as recruitment and retention, are reported on a dashboard.
Based on the insights derived from the analysis, the platform provides recommendations for mitigating risk to optimize the research protocol. Multiple team members can collaborate on the platform to help navigate stakeholders through their trial. This enables all team members to be informed.
At 2:20 in the 6-minute video below, Kim Walpole, co-founder and CEO of Trail.AI, discusses the features and potential benefits of her company’s platform.
Walpole claims that Trials.AI helped the Moores Cancer Center at UC San Diego Health shorten its study timelines by nearly 33% and reduce data errors by 20%.
The revenue model entails a one-time onboarding fee and a subsequent monthly data storage fee per trial. At the time of Walpole’s presentation, sponsored trials onboard at $15,000 with a $833 monthly fee. Enterprises onboard at $100,000 with a $250 monthly fee.
We were unable to find an estimate for the total amount of funding that Trials.Ai has raised However, investors include DreamIt Ventures, Nex Cubed, and EvoNexus. According to LinkedIn, the company’s Principal AI Architect is Fuad Rahman, who earned his PhD in Computer Vision and Pattern Recognition from the University of Kent in the United Kingdom.
BullFrog AI
Founded in 2017 with headquarters in Annapolis, Maryland, BullFrog AI claims that it uses machine learning to predict more accurate matches of patients to therapies in development.
The startup claims that its platform bfLEAP was developed over the past seven years at The Johns Hopkins University Applied Physics Laboratory. Trained on high volumes of clinical data, BullFrog AI’s algorithm drives the platform which conducts data mining to identify relationships and patterns within large, complex data sets.
For example, the software application starts the process by receiving a set of clinical data, the data is cleaned, analysis is performed, data correlations are made and predictive models can be generated.
In February 2018, the startup announced its strategic partnership with the Lieber Institute for Brain Development in an effort to improve antipsychotic drug selection for patients. In a quote, Kristin Bigos, MD, a lead investigator with the Lieber Institute, provides more context around the challenges to targeted drug selection:
Until now there has been no effective alternative to the trial and error approach for matching patients to the best antipsychotic. Being able to understand and predict which drugs will work best for which patients would be of tremendous benefit to both patients and the physicians that serve them.
In a case study, the startup claims that from a set of 240 million data points, the system identified 30 targets of interest, and ultimately seven of those were confirmed as accurate targets. Using standard methods, the same process would required 70,000 targets instead of just 30 to achieve the same results.
We were unable to determine the amount of funds the company has raised to date through our research, nor does the company list any employees on its Linkedin Profile. It seems Bullfrog is just getting off the ground.
Clinical Trial Optimization
Brite Health
Founded in 2015 and based in Palo Alto, California, Brite Health claims that it leverages machine learning to better manage patient engagement in clinical trials.
The company’s patient app and site dashboard are driven by its algorithm, which is reportedly trained on millions of clinical data points. The recommendation engine is able to identify key markers that tend to correlate with patient disengagement from research studies.
For example, through the mobile patient app (shown below), the system sends notifications to the user related to their scheduled tasks and site visits throughout the clinical trial. Engagement with the app is also encouraged through curated content and personalized communication. A conversational chatbot customized with approved study materials is available to patients at any time.
Research teams are able to monitor patient engagement and adherence using the platform’s dashboard (shown below). Insights and notifications from the recommendation engine allow earlier intervention with patients to prevent nonadherence and dropouts from the study.
We were unable to find case studies or the total amount of funds raised through our research. However, current backers include Plug and Play Ventures and Unshackled Ventures.
Brite has participated in a Health and Wellness Innovation Program led by Pfizer, and Bayer’s Dealmaker program.
There does not seem to be any C-level executives on the team with an AI background. We caution readers to be wary of companies that claim to do AI without any C-level AI experts on their team.
Takeaways for Business Leaders in Healthcare
While it is important to note that the majority of companies profiled are within their first few years of operation, applications targeted towards patient recruitment appear to have the strongest traction.
For example, pharmaceutical firms such as Merck have invested in Antidote’s platform which may offer important value to accelerate the R&D timelines by identifying the right candidates at a much faster rate. Considering the length of a successful clinical trial can run as long as 10 to 15 years from start to finish, time-saving strategies are of critical importance.
In contrast, patient engagement and optimization are some of the newest applications of AI. These applications require more case studies to gain a deeper sense of their potential competitiveness in the pharma industry.
This data will be particularly important in the case of patient engagement. As more healthcare providers use health IT to manage patient health getting patients to consistently use multiple apps may present challenges.
For large, international studies with thousands of patients, multiple language options will be needed to meet the needs of participants around the globe.
Whereas patients seeking clinical trials are often self-motivated to participate and tools to help them discover relevant opportunities could be an easier sell.
As the volume and complexity of clinical data continue to increase, AI applications offer potential value to the clinical trial process and the pharmaceutical drug industry.
Header Image Credit: International Myeloma Foundation