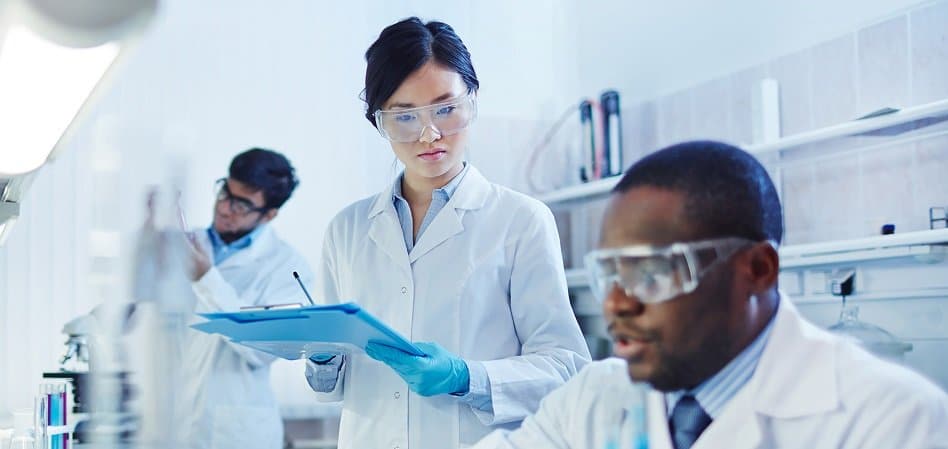
Business leaders in pharma and life sciences have more specific business intelligence needs than peers in other industries. In addition to common business drivers like marketing, profit and loss, and customer churn, pharmaceutical companies need information regarding patients and clinical trial results.
Long histories of clinical trials can be difficult to comb through to find insights on how to plan future ones, and past results can be helpful when trying to identify best practices. As such, software vendors offer solutions to pharmaceutical companies to help them find useful information from their past work and produce the best plans of action for their clinical trials.
Business Intelligence in Pharma and Life Sciences – Insights Up Front
Many of these vendors offer AI and machine learning to automate the process of sifting through data and creating visualizations to study that data. Predictive analytics is the predominant application for this type of solution because there is a high volume of numerical and structured data within the space.
Dataiku claims its solution, the Data Science Studio (DSS), helps optimize clinical trials by making predictions on the results of planned trials. They state the software can simulate those planned trials to give clients a more detailed look at how the prediction was made and which factors they can change to improve results.
Domino Data Lab’s software platform is advertised to help with clinical trial design and emphasizes reproducibility. This would help pharmaceutical companies prove their trial results in order to comply with healthcare regulations. Domino Data Lab also claims users can revisit trials to find insights on topics that were relevant in the past and have come up again more recently.
Complexica offers a more widely-applicable solution which it claims enables companies to find information on business operations, clinical operations, and marketing initiatives. Users can search with Complexica’s digital analyst to gauge marketing conditions for their products, which can help drive more valuable marketing investments.
Medidata claims their MEDS platform can compare the performance of a client company’s clinical trials with that of their peers. This allows business leaders to see differences in clinical operations between companies and make decisions on how those operations could change to improve results.
There are many companies that claim to offer AI-powered business intelligence software to life sciences and pharmaceutical companies. The solutions we found are intended to help these companies with at least one of these business problems:
- Planning Clinical Trials
- Conducting Clinical Trials
- Marketing
We’ll begin exploring applications one by one, beginning with use cases for clinical trials planning:
Planning Clinical Trials
Dataiku
Dataiku offers software called Dataiku Data Science Studio (DSS), which it claims can help pharmaceutical data and BI teams optimize their clinical trials using predictive analytics.
The software uses clinical data in different ways depending on the data analysis needs of the client. Dataiku DSS can create predictive models related to a patient’s response to the drug being studied, as well as run a simulation to show what the optimal number of patients might be for testing that drug. It can also purportedly detect chemical signals received by Internet of things (IoT) sensors as data and predict an adverse effect of a drug before it is given to a patient. These signals are called biomarkers.
Dataiku DSS’s machine learning model was trained on thousands of pharmaceutical data from various sources. These include clinical trials, electronic health records (EHRs), and data from IoT sensors. Each type of data would contain individual points pertaining to patients’ likelihoods to respond to the drug, adverse effects they might experience, and the number of patients enrolled in the trial. The data would then be run through the machine learning algorithm, which would have trained the algorithm to discern which data points correlate to clinical trials with the highest rates of positive patient response and the least adverse effects from the drug being studied.
The software would then be able to make predictions about the optimal size for future clinical trials, which patients will most likely respond to the drug, and if patients might experience adverse effects from the drug. This may require the user to upload new clinical trial protocol or recently discovered adverse effects into the software beforehand.
Dataiku claims users can integrate the software into existing databases for business intelligence data, such as a digital portfolio of clinical trials. These databases would be split up and categorized as datasets once integrated into the software.
Below is a demonstration video showing the first steps a user might take when working with Dataiku DSS. It is over 14 minutes long; however, we have found three main sections to consider when researching DSS:
- 0:00 – 4:18 gives step by step instructions on how to import and access datasets
- 4:19 – 9:45 shows how to prepare or “clean” the data for the purposes of the predictive model being made
- 9:46 – 12:50 shows how models can be created, depicted, and modified
Dataiku claims to have helped AramisAuto create business intelligence and data analytics projects in-house. AramisAuto deployed Dataiku DSS for their business intelligence engineers and data scientists and trained them to use the software for predictive modeling and teamwork.
Before deploying the software, AramisAuto was outsourcing its business intelligence initiatives to other companies for accurate insights. According to the case study, they became independent in developing data analytics and strengthened their data experts’ skills in using Dataiku’s software.
Dataiku also lists Primera Blue Cross and Essilor as some of their past clients. Business leaders should be aware that these are not pharmaceutical companies, however, but occupy different sectors of the medical space.
Florian Douetteau is CEO at Dataiku. He holds an MS in Mathematics and Computer Science from Ecole normale supérieure. Previously, Douetteau served as VP of Research and Development at Exalead.
Domino Data Lab
Domino Data Lab offers a namesake data science platform, which it claims can help pharmaceutical companies design more effective clinical trials using predictive analytics.
The platform allows for multiple data science and business intelligence employees to collaborate on projects through comments and discussions that are saved in each project’s files.
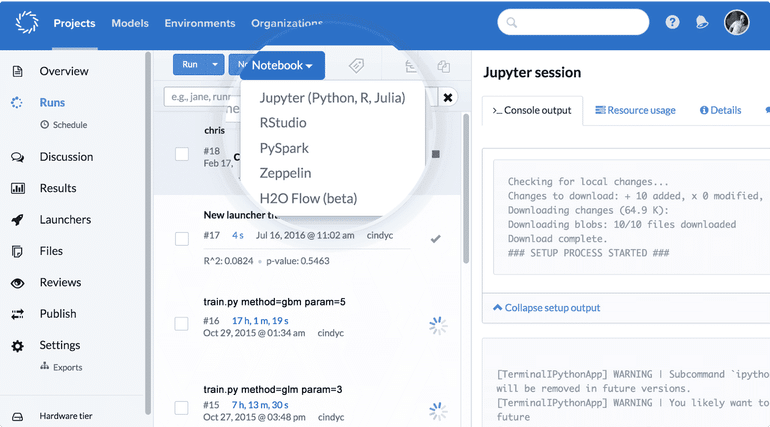
It also allows users to reuse past data analytics experiments and projects to improve on them and gain past business insights. Domino Data Lab states that all work done on their platform is saved with data and results intact to preserve reproducibility. This is crucial for pharmaceutical companies looking to easily comply with 21 CFR Part 11.
The machine learning model behind the software was likely trained on thousands of data points from past clinical trials. These data points would involve patient demographics, patient response to the drug being studied, and the risk associated with a patient or drug. The data would then be run through the software’s machine learning algorithm. Doing this would have trained the algorithm to find which data points correlate to the clinical trials with the best patient drug response and the least risk factors.
The software would then be able to make predictions about which patients will respond to the drug best and which patients or drugs pose risks to each other. This may require a data scientist to upload information regarding future clinical trials into the software beforehand.
Domino claims data scientists can integrate the software into existing databases for clinical data and previous data science experiments or projects. We could not find a demonstration video that shows how the Domino Data Science Platform works.
Domino Data Lab does not make available any case studies showing a pharmaceutical company’s success with the software.
Domino Data Lab also lists Bristol-Myers Squibb as one of their past clients.
Christopher Yang is CTO at Domino Data Lab. He holds a Master’s of Engineering (MEng) in Computer Science from MIT. Previously, Yang served as Software Developer at Bridgewater Associates.
Conducting Clinical Trials
Medidata
Medidata offers software called MEDS Perform, which it claims can help life science and pharmaceutical companies find insights regarding business operations and bring drugs to market faster using predictive analytics.
As the name implies, MEDS Perform compares clients with the success of their peer organizations. The software uses operational data from clinical trials for similar drugs or with similar practices to show the differences in success between the client and the other organizations studying drugs for the same or similar diseases.
In addition to the focus areas of cycle time, enrollment, and costs, MEDS Perform provides risk evaluations of individual patients so they can be monitored more closely if need be. The software also segments patients in the trial into groups with different likelihoods of responding well to the drug.
This is called genetic clustering. This way, operational management is able to find the general areas it needs to improve on, as well as specific cases where they can take action and improve the results of trials that are already underway.
Medidata states the machine learning model behind MEDS Perform was trained with “the largest store of clinical trial data.” It is unclear on what grounds they make that claim. The data points that would be taken from these clinical trials would be information about the clinical operations of the pharmaceutical companies that conducted them.
These include how long a trial takes to complete its cycle, enrollment, trial conduct, and costs. They would also include any information about the risks involved with certain patients, as well as the patients’ genetic data. All of this information would then be run through the machine learning algorithm behind MEDS Perform.
This would have trained it to discern which data points correlate to a high-risk patient, a cluster of patients whose genetics respond similarly to the drug, and efficient operations.
The MEDS Perform software would then be able to predict which operational procedures will yield the best results for clinical trials. This may require the user to upload the information on new operational protocol into the software beforehand.
It’s likely that users can integrate the software into their existing database that stores the client’s clinical trial and study data.
Unfortunately, our research yielded no results when we looked for a demonstration video showing how MEDS Perform works, and it seems as though Medidata does not make available any case studies showing success with its software. However, Medidata lists Worldwide Clinical Trials and Tesaro as some of its past clients.
David Lee is Chief Data Officer at Medidata. He holds a Master’s degree in Statistics from the University of Columbia in the City of New York. Previously, Lee served as VP and Head of Science at AIG.
Marketing
Complexica
Complexica offers software called Larry the Digital Analyst, which it claims can help pharmaceutical companies gain operational insights and model marketing situations using predictive analytics.
Larry the Digital Analyst is not a chatbot, even though Complexica states users “ask” it questions. The software receives all requests through the clicks of a button, as opposed to a typed or spoken command. The software is used across multiple data analysis solutions that Complexica offers, including their What-If Simulator and Optimizer, Promotional Campaign Manager, and Customer Opportunity Profiler.
Pharmaceutical corporation Pfizer’s Australian branch chose Complexica to improve its business decision-making process. Its chosen solution was the What-If Simulator and Optimizer, which uses Larry the Digital Analyst to model sales, marketing investments, and market conditions. Marketing conditions could include a seasonal rise in demand for a certain drug or which retailers are seeing the most sales of that drug.
The solution also uses the Digital Analyst to optimize sales territory mapping based on size of the territory, the roles of the sales representatives, and geographical location. It should be noted however that Pfizer was the only major pharmaceutical client that could be found on Complexica’s website by the time of publishing.
The company states the machine learning model behind Larry the Digital Analyst was trained using thousands of data points from sales, marketing investments, and marketing campaign projects. It is likely that it would also need to be trained on drug sales categorized by segments based on customer health needs.
An example of this would be geographical sales data across a drug for adults and its child equivalent. These data points would involve customer profiles, the impact of investments, and market conditions such as rises in demand due to seasonal or unexpected outbreaks.
The data would then be run through the software’s machine learning algorithm. This would have enabled the algorithm to determine which data points most often indicate customers with the most opportunity value, effective investment strategies, and favorable market conditions.
The software would then be able to make predictions regarding which possible customers offer the most sales opportunities, which marketing investments will pay off, and when to launch a marketing promotion. However, this may require the user to upload data about their future promotions, recent changes to their marketing strategy, or recent rises in demand into the software before conducting the analysis.
Below is a short 2-minute video demonstrating how Complexica’s “Larry” Digital Analyst provides predictions based on the user’s needs:
Complexica does not make available any case studies reporting success with Larry the Digital Analyst.
Complexica also lists Pfizer Australia, Sigma Healthcare, and Boehringer Ingelheim as some of their past clients.
Zbigniew Michaelwicz is Chief Scientist at Complexica. He holds a PhD in computer science from the Polish Academy of Sciences. Previously, Michaelwicz served as Chief Scientific Officer at SolveIT Software.
Header Image Credit: Healthy Debate