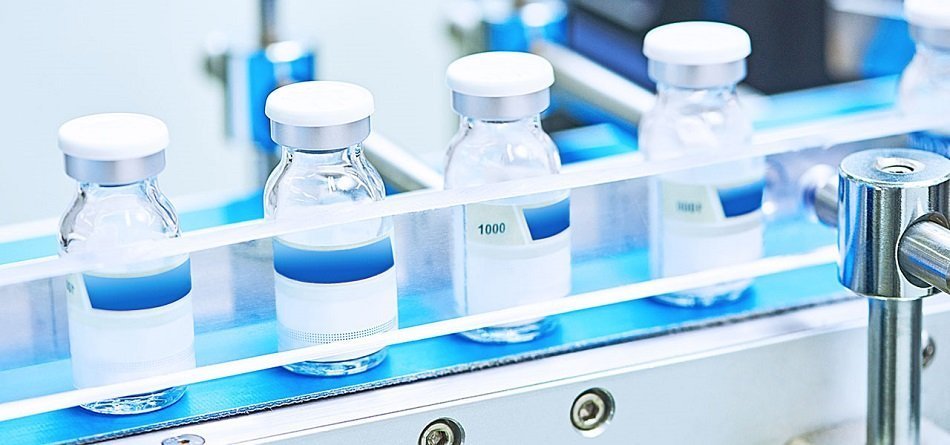
Accenture reports that in 2017, the 16 top biopharmaceutical companies in the world had an aggregate global revenue of $428 billion, which was nearly half the global pharmaceutical market by net sales. The report also revealed a shift to specialty drugs for hard-to-treat diseases.
AI has numerous applications in healthcare broadly, and with the help of AI in the pharmaceutical industry, global businesses and startups are collaborating to develop new treatments. Previously, we looked at AI healthcare innovations in Europe, and in this report, we specifically look at the European AI vendors that are offering machine learning solutions to pharmaceutical companies. This report covers vendors offering software across three applications:
- Salt and Polymorph Screening
- Discovering Single and Bispecific Small Molecules
- Matching Patients with Treatments
We’ll begin with initial insights for European pharma leaders, and continue with an assessment of AI vendor companies and capabilites below:
What Business Leaders in the European Pharmaceutical Industry Need to Know
The companies listed in this report all employ robust data science talent or people who, given their academic credentials, would be capable of working with volumes of data to build machine learning models. This bodes well for AI in the European pharmaceutical industry. Many industries are filled with purported AI vendors that claim to do AI but in fact couldn’t possibly offer machine learning solutions. This is because they don’t employ data science and/or AI talent, which is one of the rules of thumb we use when vetting a company on their claims.
For instance, Owkin offers a drug modeling software. It employs several data scientists with Master’s in specific AI-related fields, such as computer vision and data science. Many of these data scientists are fresh out of their Master’s programs, which increases the likelihood that they learned about machine learning while in school.
Healx also offers a drug modeling software, and their Head of AI holds a PhD in Computational Biology from 2008. This bodes well for the team that works under him and the company at large because his degree indicates a relatively rare combination of both subject-matter expertise and artificial intelligence talent. Both of these aspects are essential for building machine learning models to solve business problems, and it’s not often that they exist together in one person, as we discuss in our executive guide, Applying AI in Business – The Critical Role of Subject-Matter Experts.
Exscientia offers an AI-based drug discovery application. Although it doesn’t employ many data scientists according to Linkedin, it employs one with a PhD in Pharmacology and a Master’s in Data Science, which he earned in 2015. In addition, the company’s CTO holds a PhD in Bioinformatics from 2006. As a result, we believe that the company has a decent likelihood of actually offering machine learning software.
Finally, Tesella is a data science consultancy that employs a large volume of data scientists with PhDs in computer science and hard sciences. Their services have been used to build machine learning models for pharmaceutical companies looking to do salt and polymorph screening faster. Given the density of PhD-level talent on their team, we believe the consultancy has a high likelihood of being able to help companies build machine learning models like they say they can.
Salt and Polymorph Screening
Tessella
Tessella is a data science consultancy that offers AI and data science services. They claim to be able to help life science companies develop pharmaceutical treatments faster by helping them build machine learning models.
We can infer that one of the machine learning models they claimed to have built was trained was trained on data related to small atoms or molecules, chemical formulas, and their behavior when they bind. The data would then be run through the machine learning algorithm to discover which low-energy compounds would show stability when binding. This would have trained the algorithm to discern which data points correlate to their level of stability.
The software would be able to predict which molecules would best bind to each other to form stable compounds that can be applied to new drugs, and their efficacy in treating diseases.
Tessella claims to have helped GlaxoSmithKline (GSK) improve its salt and polymorph screening process of drug compounds. This is an important step in drug development to determine the compound’s solubility in water, crystalline form, and binding stability when it is created. Salt and polymorph screening is necessary for selecting the best physical form for the drug substance when it is administered to patients. It also determines the shelf life of the drug.
Tessella helped the company create a machine learning model to automate processes such as liquid addition/mixing, solid dispensing, heating/cooling, shaking, and sample transfer. The user could enter details about the screening into the system to create the protocol for each of the 50 to 400 experiments, including the type of solvent or counter-ions. The system would then calculate the amounts to be dispensed, depending on the material purities and solubilities identified by the user.
During the screening, data including Raman spectra, X-Ray Powder Diffraction (XRPD) patterns, and optical microscopy images, was collected by the system. GSK processed the data to identify the polymorphic forms of the compounds. The system presented the experiments as an array, which enabled the user to see trends and compare the findings. The data and final result were then published by the chemist.
The case study reports that the automated screening process developed by Tessella created consistency to ensure that the test methods and results could be replicated and minimized contamination among the samples. The new workflow also reduced costs, streamlined the work, eliminated the manual notetaking, and centralized the data.
Tessella also lists AstraZeneca, Unilever, Altran, Statoil, Clinigen, British Petroleum, Spatial Ecology & Epidemiology Group at the University of Oxford, and Fusion for Energy as some of their past clients. The company was acquired by the Altran Group in 2015 for an undisclosed sum.
Nick Clarke is the Head of Analytics at Tessella, where he has worked for more than 19 years, rising from software engineer to project manager, program manager, Predictive Analytics Program Manager, to his current role. He holds a PhD in Theoretical Chemistry from the University of Liverpool. Previously, Clarke served as research fellow at the University of Birmingham and University of Milan.
Discovering Single and Bispecific Small Molecules
Exscientia
Exscientia offers a drug design software which it claims can help pharmaceutical companies discover small molecules and compounds that could treat single and bispecific target diseases using machine learning.
We can infer the machine learning model behind the software was trained on data related to small molecules, compounds, and their properties or binding behavior, chemical formulations, and the target diseases. The data would then be run through the software’s machine learning algorithm. This would have trained the algorithm to discern which data points correlate to the molecules that would show stability when combined, and their efficacy to treat specific target diseases.
The software would then be able to predict which molecules would stay bonded when they are applied to the target disease. This may or may not require the user to upload phenotypic data such as clinical information regarding patients’ disease symptoms, as well as demographic data such as age, ethnicity, and sex into the software beforehand.
We could not find a demonstration video showing how Exscientia’s software works.
The company also does not feature official case studies on its website, but in July 2017 announced that it entered into a contract with GlaxoSmithKline (GSK) to research and discover small molecules for up to 10 target diseases. The report did not specify the length of the contract.
Part of the agreement was to reduce the number of compounds required for synthesis in the discovery of the candidate compounds. The news item reports that Exscientia intended to use its machine learning algorithms and big data resources to design novel molecules. No other details were provided in the report.
Exscientia explains on its website that drug discovery for bispecific targets uses a similar process. The difference is that is that potency of the compound must show efficacy in two different targets at the same time.
Exscientia also lists Evotec, Sanofi, Sumitomo Dainippon Pharma, and Sunovion as some of its clients. The company has raised about $17 million in funding from Evotec and Frontier IP Group, and earns about $2M in revenue.
Adrian Schreyer is the CTO at Exscientia, where he has worked for 5 years. He holds a PhD in Structural Bioinformatics and Drug Discovery from the University of Cambridge. Previously, Schreyer served as postdoctoral research assistant at the University of Dundee and as a postdoctoral research associate the University of Cambridge.
Matching Patients with Treatments
Healx
Healx is a UK-based company that offers a software called HealNet, which it claims can help pharmaceutical companies match rare disease patients with drug treatments using machine learning.
Healx claims that the application takes data from clinical trials and finds correlations between patients’ rare diseases and drug compounds that could potentially be used in treatments. The HealNet database contains information about more than 7,000 rare diseases and treatments. The database, consisting of information sourced from publicly available and exclusive sources, includes scientific literature, patents, clinical trials, disease symptoms, drug targets, multi-omics data, and chemical structures.
Using the data, the company claims to develop potential therapies that combine pharmaceuticals and nutraceuticals as the machine learning predicts compounds that could work well together. The company website did not explain in detail how this works, but we can infer that this is done when the algorithms search the database to find data with similar attributes as the clinical trial being performed or the rare disease being studied.
The algorithms might then correlate the patient’s physiological condition to a combination of little-known treatments and rank the combinations according to their probability of success. The algorithm also predicts if known drug treatments for other diseases can be used for rare diseases, a process called drug repurposing.
Healx claims to be helping FRAXA Research Foundation identify and validate approved drugs that could be used to treat the inherited disease called Fragile X syndrome. Despite extensive research, no effective treatments have been found. This project is helping the foundation expedite the availability of treatment for patients.
Eight repurposing candidates were identified to be treated for six months. The compounds identified by Healx had not been previously tested against Fragile X. This project also identified new potential treatments.
According to the study, several candidates showed progress. One is advancing to the phase 2a trial. According to the company, Healx’s work has expedited the drug discovery process, resulting in a timeline of 18 months from project start to Phase 2a clinical trial. Healx and FRAXA Research Foundation are also collaborating on two further projects to identify drug combinations and biomarkers for fragile X syndrome.
Healx does not list other clients but has raised $11.9 million in funding from Amadeus Capital Partners, Balderton Capital, Jonathan Milner, and Pitch@pPalace.
Ian Roberts is the CTO at Healx. He holds a PhD in biology and biological sciences from the University of Cambridge. Previously, Roberts served as founder at Assert Informatics, Director of Informatics at Population Genetics, and as Senior Bioinformatician at 14M Genomics.
Owkin
Owkin is a France-based company that offers a dataset called Socrates, which it claims can help research institutions, biomedicine, and pharmaceutical companies create predictive models and optimize drug development using machine learning and deep learning.
Owkin claims that medical and pharmaceutical researchers, doctors, biologists, and other non-machine learning experts can use the application to conduct their AI-driven projects and develop predictive algorithmic models.
The application’s algorithms have been pre-trained and work with a mix of structured and unstructured data such as:
- text reports from consultations, surgery, and radiotherapy
- medical images radiotherapy and histology
- biological measures such as biochemistry and genomics
The company did not describe how the user interacts with the application but says that the algorithmic models could discover biomarkers, which are substances in an organism that could indicate a disease, infection or environmental exposure, by searching the database for data with similar attributes as the patient’s data. This enables the researchers to find biomarkers. Finding these biomarkers could:
- help diagnose a patient
- predict the severity of an illness or the risk of a relapse
- predict a patient’s potential response to a new drug and improve treatments if needed.
Owkin does not have a demonstration video available showing how its software works, nor does it feature any case study in its website but lists Roche, Amgen, Institut Curie, INSERM, and Mount Sinai. as some of its partners. The company has raised $18.1 million in funding from GV, Otium Venture, Cathay Innovation, Plug and Play, and NJF Capital.
Gilles Wainrib is co-founder and Chief Scientific Officer at Owkin. He holds a PhD in interdisciplines that include applied math, physics, biology, and economics from Ecole Polytechnique. Previously, Wainrib served as Assistant Professor at the Ecole Normale Superieure and Universite Paris.
Header Image Credit: HygroMatik GmbH