Today, customers interact with banks and financial institutions across several different channels which has lead to an explosion in customer data being collected by these organizations. This data can be effectively leveraged using AI to gain insights on current and future customer behavior.
There have been many instances of financial institutions instating innovation centers focused on artificial intelligence and machine learning to take advantage of their data ‘plumes.’ This history hints that banks and financial institutions might need to acquire the technological skills to create better products and customized experiences which can potentially increase revenues and decrease costs.
Predictive analytics is one such AI application that could help banks to optimize their processes while simultaneously reducing cost and resources deployed. In this article, we will highlight four applications for predictive analytics in finance through the use of case studies from companies in the space. We segment these applications as:
- Fraud Detection and False Positive Reduction: Using predictive analytics to pick up on the minute differences in transactions to determine their legitimacy
- Managing Credit Card Default Risk: Default rates may occur when a credit card holder does not pay back their debts.
- Modeling Customer Lifetime Value: A prediction of the net profit attributed to the entire future relationship with a customer and a bank.
For more information on how AI applications such as predictive analytics can help financial institutions and banks continue to innovate, download the Executive Brief for our AI in Banking Vendor Scorecard and Capability Map report.
We begin our exploration of predictive analytics applications for financial institutions with Dataiku’s fraud detection solution.
Fraud Detection and False Positive Reduction
Dataiku
Dataiku, founded in 2013, claims to have developed machine learning techniques that used to analyze raw data (such as historical transactions for a particular product or customer transcripts from sales interactions in retail) in many formats aimed at building predictive data models. The company claims their software can help businesses forecast and find relationships in the raw data which in turn leads to higher efficiency and lower operational costs.
For example, due to the stringent regulations in the banking sector, major banks, such as Wells Fargo, produce large amounts of raw data in the form of customer conversations, transaction data, marketing campaigns, social media content and website management. With this process:
- Marketing managers at the bank or an internal fraud detection team might gain access to predictive analytics insights from the Dataiku software by means of a dashboard which prompts with employees notifications or notes of anomalies in transaction data.
- Along with detection, the company claims its software can collect, clean and analyze raw data on customers to gain actionable insights such as:
- Relationships between social media content and campaign sales might be identified to understand customer trends and predict untapped marketing segments.
- Patterns in international transfer transactional data and customer interaction data that might help identify banking fraud and allow the bank to build further prevention policies.
When a user logs into the data system, they can upload or integrate data to be organized by the platform. According to the company, the data shows up in spreadsheet format and is organized. The software will associate traits to the data. For example, the company says it can note whether specific data is associated with a male or female customer, or a customer in a certain age range. For further organization purposes, and to identify where there may be missing data, each column, such as one showing age or gender, has a small proportion scale at the top to give a user an idea of how many missing values were found in that column.
From there, a user can click on the head of each column for data visualization options, which could allow them to see this data in charts or graphs. They can also generate graphs cross referencing different columns. If the user suspects there is outlier data, the program also has options that prompt a user with instructions on how to correct it and further train the program.
Below is a 4-minute demonstration video from Dataiku showing how businesses can view, edit, monitor and gain insights from raw data on the predictive analytics platform:
In a 2017 case-study, Dataiku claims to have worked with BGL BNP Paribas (based in Luxembourg) in developing an upgrade for the bank’s existing fraud detection system:
According to Dataiku, BGL BNP Paribas’ former machine learning model for fraud detection was limited by lack of access to data projects and data science resources (curated data and data science engineers who can organize the bank’s data to collect data proactively across teams)
BNP used the Dataiku DSS to ensure that all the data collected by the bank, transactions made by customers, geographical locations of customers, international fund transfers and other actions were easily accessible throughout the company, according to the company.
According to the case study the project took eight weeks to complete and involved data analytics users (such as BNPs data security or fraud detection teams) from the fraud department and data scientists from BGL BNP Paribas’ data lab working alongside data scientist from Dataiku.
Dataiku claims that at the end of eight weeks, BGL BNP Paribas was able to launch their new fraud prediction project with a reasonable level of accuracy in fraud prediction. No further details on measurable results for this collaboration were available at the time of writing.
As an outcome of this project, Dataiku says BGL BNP Paribas might have gained the ability to test (within two to three weeks) new AI use-cases by leveraging their data. Dataiku claims that BNP has begun three additional data science projects following the first fraud prediction prototype.
The 170+ employee company’s VP of Data Science Louis-Phillipe, has a PhD in Operations Research from the Grenoble Institute of Technology in France. The company claims to have worked in predictive analytics projects with customers such as AXA, L’Oreal, Bechtel, Webbmason, Urban Insights.
Teradata
Teradata was founded in 1979 in San Diego and currently has over 14,000 employees. In 2014, Teradata acquired Think Big Analytics, founded by Google’s Technical Director for Applied Artificial Intelligence, Ron Bodkin. Teradata has since begun to offer what they claim is an advanced AI-based analytics platform. This software can be used in several industries including media, financial services and healthcare, according to the company.
From its tutorial videos, Teradata seems to be more suited for data scientists, but can be personalized to collect and organize a variety of data. For banking:
- Teradata claims that they can build and develop enterprise level solutions where the raw data like customer information is collected, cleaned, analyzed and presented using machine learning algorithms.
- The fraud detection team at the bank can use the software’ dashboard to view alerts for anomalous transactions.
- The alerts are then investigated further by human analysts in the bank’s fraud detection team to determine if there was an instance of fraud in that particular alert event.
With the program, an analyst or bank employee can upload or integrate datasets and assign them labels such as “bill pay” or “credit card application.”
From there, according to Teradata’s Youtube playlist, a bank employee with less data science experience can then use the program to see “Paths” related to a data set. When they log on to the site, they can click the paths field and get a drop down menu with various data set labels or banking topics. The program, according to Teradata, analyzes statistics, and shows an individual’s activity through a visual image of a “path.”
This path includes labels of where a bank customer or group of bank customers’ various banking actions took place. For example, if a banker was interested in seeing what led users to signing up for a credit card, they could search for that related path on the Teredata site. The platform could generate a path saying that someone went to a credit card form, then contacted customer support, and then signed up or did not sign up for a credit card.
Teredata claims that the program can also use these paths to give a user predictive insights on other topics such as showing them paths that may signify fraud.
The 5-minute video below gives a demonstration of how Teradata’s guided analytics programs can be used to analyze online banking data:
In a case study from Teradata, the company claims that the Nordic Danske Bank used their analytics platform to better identify and predict cases of fraud while reducing false positives.
The study notes that Danske needed to find a better way to detect fraud since their traditional rules-based engine had a low 40-percent fraud detection rate and almost 1,200 false positives everyday. According to the company, over 95 percent of cases investigated were not found to be fraud.
The bank’s existing systems had similar user interaction process as mentioned for the Teradata project but with much lower rates of successful fraud identification.
According to the study, Danske implemented an “upgraded” fraud prediction and detection analytics platform. Teradata says they assisted the bank with upgrading its older machine learning models to a deep learning prediction model capable of identifying fraud in multiple channels including mobile transactions.
The case study notes that this first involved data scientists at Teradata working with employees of Danske for gathering and cleaning any existing data like customer transactions and location and establishing a ‘data pipeline’ for both existing and emerging datasets which would ensure access to the ‘right kind’ of data for the AI platform.
Teradata claims that Danske deployed their deep learning software that could generate and compare many different models for fraud detection based on data like customer geographic locations or recent ATM transactions. The model which performed the best in terms of identifying anomalies in customer and transactional data was chosen as a potential roadmap for future model iterations.
The system was not completely autonomous, Teradata noted. While it could identify anomalies in the transaction data, these detections would then have to be designated as a case of fraud by a human analyst, according to the study. For example, the platform may identify anomalies as a customer’s debit card purchases start occuring around the world, but a notified human analyst would have to investigate if this was a case of fraud, or if the customer made an online purchase that sent the payment to China followed by a purchase while vacationing in London.
After a five month setup and integration period Teradata claims that their deep learning model was able to perform significantly better than Danske’s existing rules-based engine and machine learning model in terms of reducing false positives in the anomalies detected. The figure below shows company results of the product:
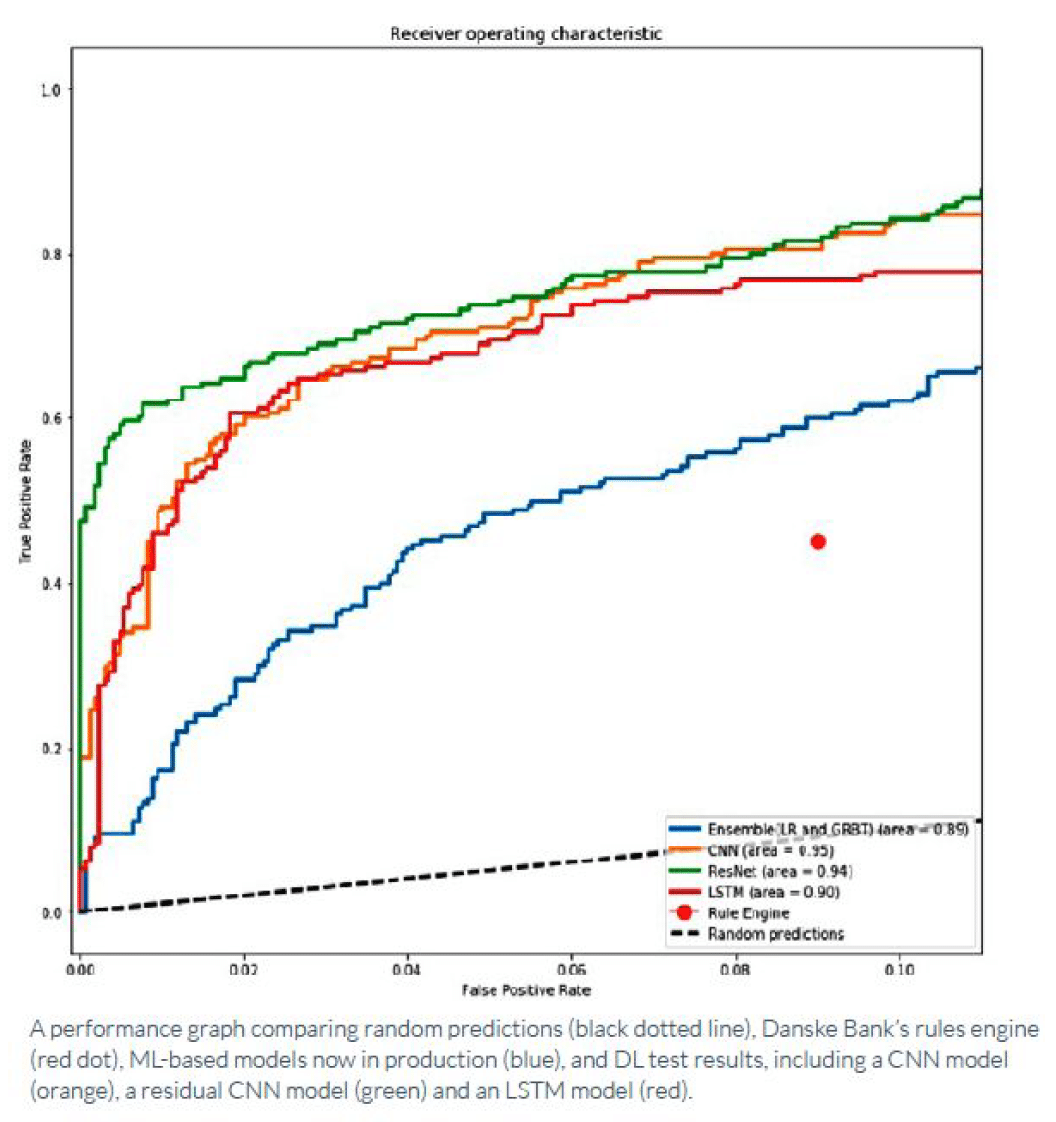
Reducing false positives may be an important way some companies can enhance their user experience. When customers do not have to worry about their legitimate transactions getting recognized as fraudulent, their engagement with the company’s brand may become more amicable than before We spoke with Sebastien de Brouwer, Chief Policy Officer of the European Banking Federation, about where business leaders should be focused in terms of AI on our podcast, AI in Banking. When asked about which capabilities will matter in terms of being critical in the future, de Brouwer said,
“We strongly believe that AI will have indeed a transformative effect on the banking industry … The most important aspect is certainly that it will change and hopefully enhance the customer experience. So that’s already a very important element I think for the banks who will succeed, and that is of course interactivity with the clients, because this should allow [banks to use interactivity data to create better offerings.] … One activity where many banks are looking at is investment advisory or recommendations. I think that is certainly an area where no big players are looking very seriously at AI [as a solution.] … This may also expand the client segments that would have access to those kinds of services.”
Along with Google alum Ron Bodkin’s experience, the team’s Principal Data Scientist, Jack McCush previously earned a Master of Arts in Statistics and a Dual Masters of Arts in Economics and Statistics from the University of Missouri-Columbia.
Teradata also claims to have worked on projects with companies like Maersk Line, Verizon, Siemens and Proctor and Gamble.
Managing Credit Card Default Risk
DataRobot
DataRobot is a Boston-based startup founded in 2012. The 400+ employee company claims to offer predictive analytics services in the FinTech space through its Automated Machine Learning platform.
When a bank employee or lender logs in, they see a data dashboard showing columns and cells with key aspects that they would like to monitor. They dashboard is also capable of showing insights and trends in various graph formats. A user can also search and look at specific data associated with someone applying for a loan and his or her loan application to determine if they should get approved.
According to DataRobot, its services aim to predict risk in lending (credit default rates) or identify anomalies in payment transactions for fraud detection. For example:
- The Bank of America (BofA), one of DataRobot’s clients, might lend money to customers in the form of loans or credit cards and growing their business means increasing the value and number of such loans. The bank may need a scalable strategy to predict the likelihood (risk) of default among large numbers of applicants.
- The bank’s loan managers might use the DataRobot platform to gain insights on risk of loan defaults for new customers by means of a dashboard.
- The AI platforms are trained using historical loan repayment records and other data like social media data to coax out patterns that might lead to a customer defaulting on credit card payments. DataRobot claims that their platform can also clean and parse the raw data although users can also use third party data cleaning tools like Trifecta (see video below)
- Loan managers can then use the dashboard to review the applications that have a high risk of default thereby speeding up the loan approval process.
- BofA might use DataRobot’s predictive analytics platform to predict the risk of default for new borrowers by analyzing historical data about existing borrowers default rates. By integrating these predictive models into their loan-approval the bank could potentially expand their loan portfolios while simultaneously managing the risk involved, according to DataRobot.
Below is a 1-minute video which gives a demo of how businesses can leverage their internal data using DataRobot’s Automated Machine Learning & Predictive Modeling Software:
From our preliminary research we found several use-cases from DataRobot of predictive analytics in FinTech applications.
When working with Crest Financial, a “No Credit Needed” lease to own company offering microloans up to $5,000 with immediate approval, DataRobot said they used predictive analytics to predict credit default rates in more detail.
- To previously attempt to predict default rates, Crest’s two-person data scientist team used information provided by the customer and additional data, like rent and utility payment histories gathered from a credit or background checks, as inputs for their built-in-house machine learning models.
- Since the Crest team was building and testing the machine learning predictive models manually, this process often took months, facing several deployment delays, according to the case study.
- Crest tested a demonstration of the DataRobot platform to understand how much more efficient it might be compared to their data science team’s efforts. After a successful test where DataRobot claims that the risk models that their software created performed more accurately in the first hour after being deployed than a month’s work of progress made by Crest’s data science team.
- DataRobot claims that after the integration of their platform, Crest was successfully able to Identify the customers in high-risk and highly-competitive markets, detect anomalies in customer transactions that might be fraudulent and predict the likelihood of default for loan applicants.
DataRobot’s current Co-Founder and CTO Tom de Godoy has previously earned BS in Physics and an MS in mathematics from UMass Lowell and has also served as the Senior Director for Research and Modelling at Travelers Insurance although we couldn’t be sure if any of the DataRobot leadership team had specific experience in AI projects previously.
Some of DataRobots clients include healthcare software company Evariant, and DonorBureau, a startup in the nonprofit space.
Modeling Customer Lifetime Value
RapidMiner
Boston-based RapidMiner, founded in 2007, claims to offer a software that can help data science teams to develop predictive models in fields including industry banking, healthcare and automotive.
The company claims to be using AI for predictive analytics in areas like pricing optimization, predicting customer lifetime value and fraud detection. Their use-case on predicting customer lifetime value states that banks might use their platform to:
- Predict the lifetime value of a customer based on their historical transaction data.
- Identify customers with high long-term values and prompt marketing options based on the type of customer.
- Identify the ‘profiles’ for ideal long-term customers which can then be used to predict if a new customer might fall under this category.
- Help direct the bank’s cost and effort towards customers that that might continue working with the bank in the future, and reduce time on customers with low lifetime value.
A bank might integrate the RapidMiner analytics platform alongside their existing enterprise sales systems (like CRMs). The customer service representatives in the bank can then use the RapidMiner dashboard to see the lifetime value for all their customers and prioritize the customers with longer lifetime value.
Below is a 3-minute video demonstration from RapidMiner showing how the RapidMiner Studio’s user interface and product extensions can be used in building predictive models.
Although we found evidence of multiple case studies from RapidMiner including a collaboration with PayPal for sentiment analysis applications, we could find no robust case study from RapidMiner in the banking and financial sector.
The company has raised over $36 million in funding so far, however we could find no clear evidence of previous AI project or academic experience in RapidMiner’s leadership team
RapidMiner claims to have worked with companies like Austria’s mobile phone service provider, Mo-bilkom Austria and PayPal.
Conclusion
Key themes:
From our research we were able to classify the most common predictive analytics applications for AI in the finance sector as follows:
- Fraud detection and prediction for financial institutions and banks.
- Predicting if a customer might default on a loan or a credit payment.
- Predicting customer behavior to maximize a company’s resource allocation towards customer that might deliver the maximum ROI over their life times
- Using customer and market data to optimize pricing of financial products and services
What Business Leaders in Finance May Need to Know Before Getting Into Predictive Analytics Projects
- Predictive analytics would require ensuring that company-wide data policies are aligned towards making the data easily accessible, as well as establishing a pipeline to continue a streamlined data collection process as seen with the Dataiku use case.
- The integration of predictive analytics platforms would also require financial domain experts to work in collaboration with data scientists in order to arrive at more accurate models.
- As with the DataRobot use-cases customized AI platform integrations could last for three to five months typically and models may still need to be fine-tuned for accuracy well beyond that timeframe.
- In most cases like that of Teradata, human analysts will still be a key part of the process for the next two to five years in most applications of predictive analytics in finance, although it’s use might become fairly ubiquitous in that period.
Header image credit: SME Finance Forum