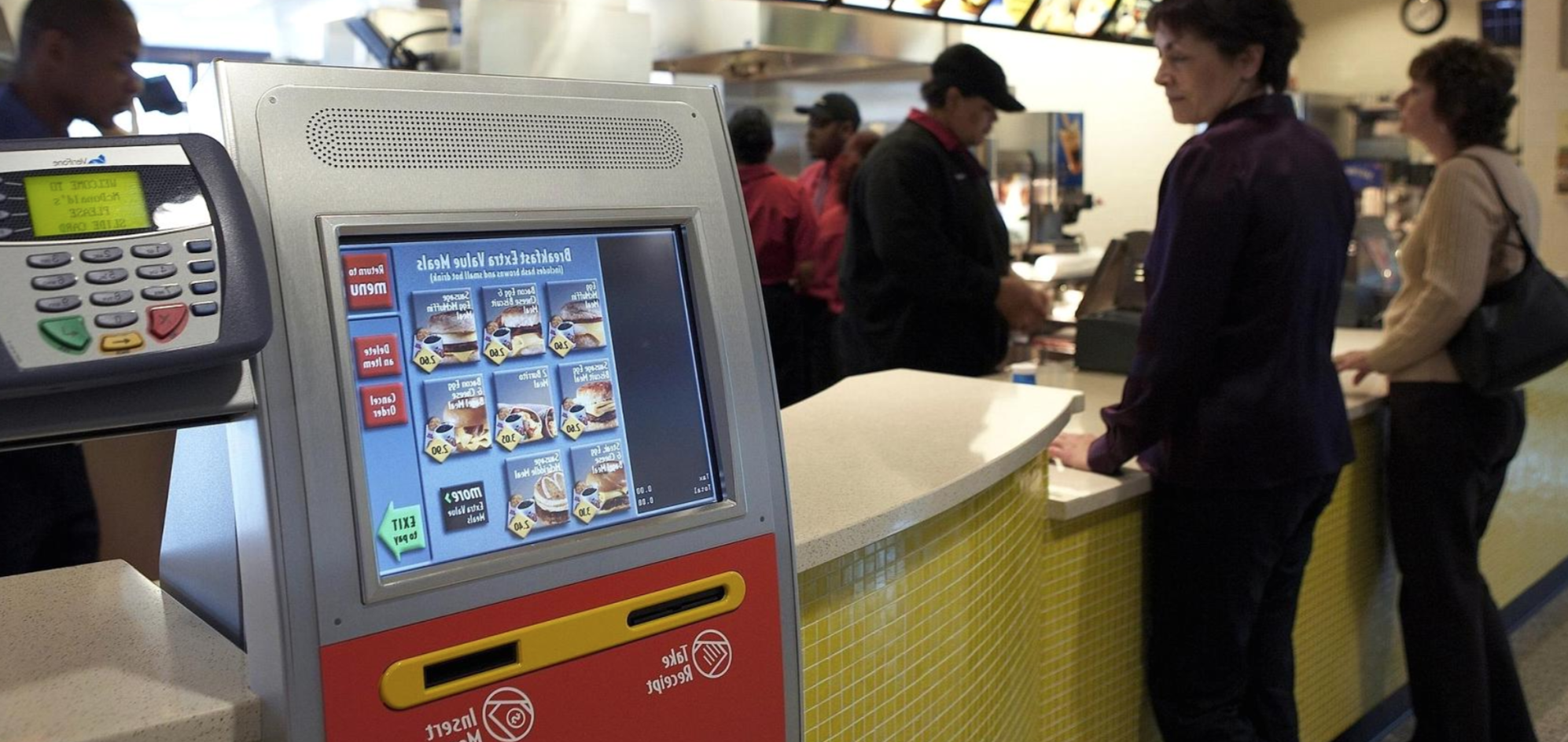
This year has been marked by an increase in artificial intelligence interest from new business sectors. Just one years ago, we hardly had any visitors or subscribers from the food services sector – and this year we have a strong interest from food processing and food services leaders, which has come as a surprise.
I was flown out this year to speak this year at the Ohio Restaurant Association’s annual conference, an event with over 4,000 attendees, mostly business leaders in the Midwest food services industry. This article is based on my preparation before that talk, and some ideas and details that I’ve added after speaking to a dozen-or-so business leaders in this sector.
Most of the audience was small business owners, but many of the perspectives in this article apply just as well to larger national food services firms.
All in all, AI has not penetrated the food services industry in general (compared to more active industries like healthcare or finance), and even less so the industry’s small- and medium-sized businesses (SMBs) – companies with revenues amounting to less than a billion dollars. Admittedly only a few trends are probably worth taking seriously in long-term strategic planning.
To help SMBs discern the right action around AI in food services, this article examines:
- The current state of machine learning in SMBs in food services
- The forward-looking trends of AI in food services
- AI technologies that may impact food services in the long term.
See the presentation deck directly below, and read the full article further down:
The State of AI Among SMBs in Food Services
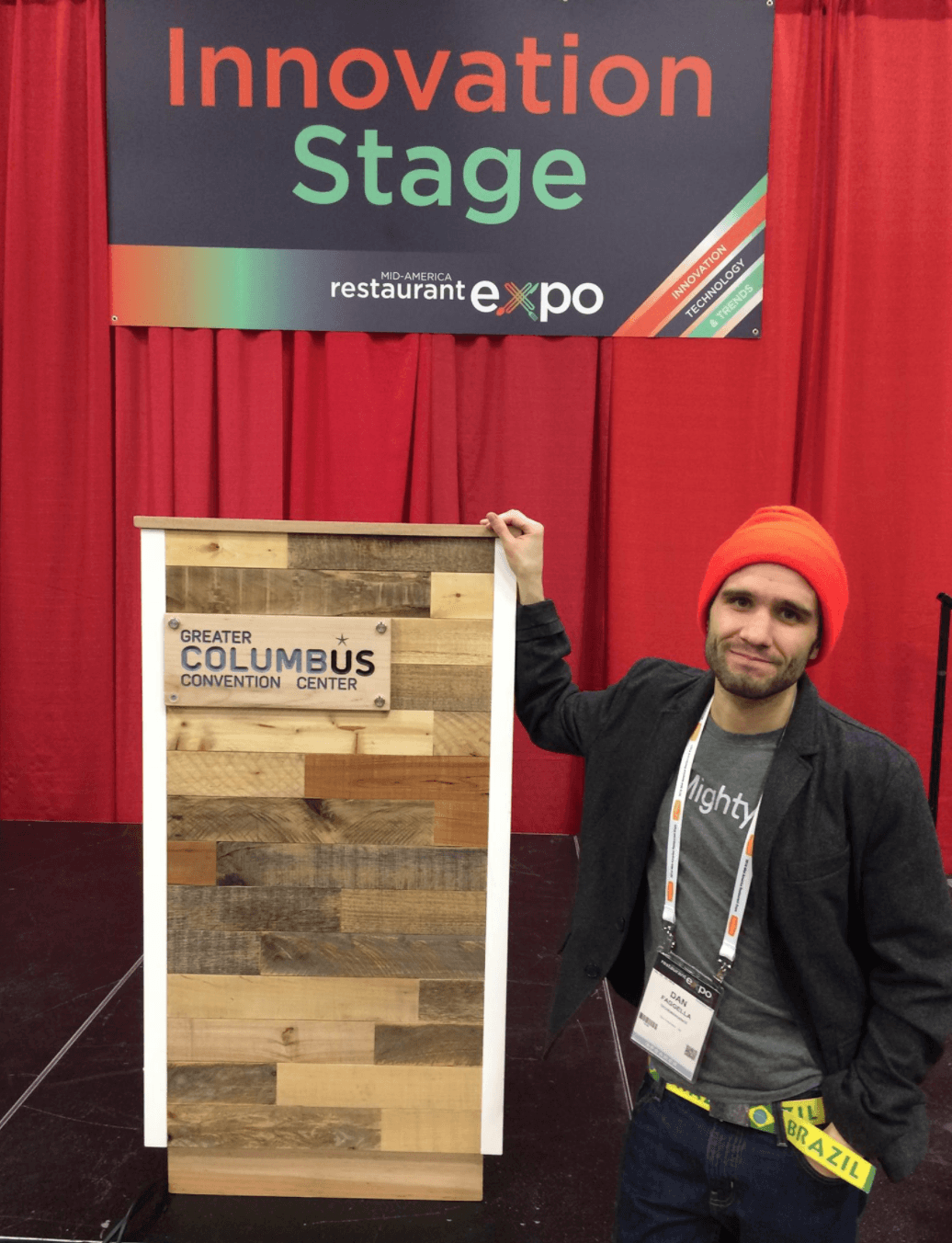
Based on our research, we have found that for every 100 “AI companies”, only 1/3 of these companies have the academic or intellectual horsepower to leverage machine learning in any serious way while many are merely marketing hype. Of this number, a third has gone past pilot testing their product or service but have yet to show any real positive impact on their bottom line.
Any existing vendor applications are mostly exclusively made for large chains that have the data flow and the business model to merit the use of AI and machine learning. Whatever announcements companies make about investments and innovation for AI in food services is mostly unsupported by clear returns at this point.
At this early phase of AI’s life cycle, most AI companies are burning through venture money, trying to thresh out use cases and to their viability in the market. This seems to be true not only in food services but also in healthcare, finance, retail and other industries.
AI is clearly not for mom and pop operations at this point, and even if a business were fortunate enough to have money to invest in machine learning technologies, it probably wouldn’t have the data science talent and the R&D experience to really get value from artificial intelligence. Hence, even firms with the budget might be better off investing in security, customer service, marketing, or other areas.
For certain, there is a great deal of noise around AI but food services SMBs need to be able to discern the grain from the chaff. As it happens, the majority of AI applications should be ignored, with only a few worth considering in long-term strategic planning, not immediate implementation. We will cover some of these impactful long-term technologies later in this article.
Machine Learning Challenges for SMBs in Food Services
Among the barriers that face AI in food services, these are the most formidable:
- Most Innovation is for Bigger Firms: Vendor applications in food services (as with most other sectors) are costly and almost exclusively being developed for larger firms. These custom applications, such as video intelligence for assessing food quality, or food services robotics, are highly complex and require experienced machine learning experts on staff, plenty of resources, and/or huge volumes of data (neither of which are common for small or mid-sized food services businesses).
- AI is Resource Intensive, and Complex: The talent, budget, and time requirements to build AI and robotics applications is much more than most companies expect. SMBs are in no position to attract the best AI talent straight out of college and to compensate them with handsome salaries and clever perks like Google or Facebook. Even for restaurants with more than a hundred locations, data science talent is probably scarce or non-existent. AI not being a core or mission-critical part of operations makes it difficult to cultivate and develop from within a food service business.
- Food Services Isn’t an Machine Learning Innovation Hotbed, for Now: The food services industry receives little attention as a distinct niche for AI, unlike eCommerce, pharmaceuticals, banking, telecommunications, among others. Whatever scarce AI talent that exists is not beating a path toward food services – and the industry isn’t likely to see the same degree of innovation as many of the sectors listed above. This doesn’t mean that innovation will not exist in food services, but that we should expect a slower pace of innovation and adoption.
Use Cases of Machine Learning and AI in the Restaurant
Note that restaurant is not listed specifically, though customer service and marketing applications are relevant to this sector.
1 – Robotics
As far as we can see, robotics has no relevance for SMBs in the near term due to issues with scalability even for the biggest companies. However, SMBs may consider robotics as an alternative workforce if minimum wages for human talent continues to increase, and indeed raising minimum wages are likely to spur more investment in the robotics space in the years ahead. Some use cases in this area include convenience store chain 7-11 which has adopted streetbots and drones in its delivery services.
Here’s a short video of how 7-11 uses a drone to make food deliveries:
CaliBurgers’ Pasadena location started using Flippy, the artificial intelligence-driven robot developed by Miso Robotics, a robot that can grab and flip burger patties, handle buns and other ingredients to create hamburger sandwiches. As of March this year, Flippy has only started working in Cali Burger’s Pasadena outlet, and if successful, will be deployed in 50 restaurants by the end of 2019.
Another application is the Moley Robotic Kitchen, a pair of robotic hands that replicates human movement to prepare home-cooked meals, from a library of stored recipes in its system. The developers intend for the Moley robot to be for consumer kitchens when it is released this 2018, but enterprise-scale use has yet to be proven. A check of the website showed that the product is still under development, and interested clients are directed to a pre-sales link that leads to a blank page.
See the Moley Robotic Kitchen in action:
2 – Smart Kiosk Technology
AI-integrated smart kiosk technology has potential, but is speculative and still in its pilot phase, even for the large food chains. For instance, McDonald’s self-service kiosks recommend products depending on a season, weather, and new or repeat customer’s preference, through a digital menu board. As well, this touchscreen menu board offers personalized options from which customers can choose their preferred amount of salt in fries, sauce in burgers and sugar in cold drinks. It also allows them to order and pay for their food, expediting process at point of sales process, reducing human error while giving customers control over their food choices.
Additionally, a mobile application will enable customers to place orders from their phone for pick up or synchronize with a kiosk to recognize their app profile, their favorite orders and preferred payment methods. McDonald’s had aimed to launch mobile ordering and payment in 20,000 restaurants by the end of 2017.
Here’s a view of how the self-ordering kiosk works:
The technology hub at Wendy’s, meanwhile, was set up to create innovations in internal technologies for business intelligence and communications. The company is currently testing mobile-, voice- and beacon-based ordering; self-order kiosks; and a loyalty program.
In Asia, KFC in January 2017 had planned to deploy kiosks in China with facial-recognition software to predict customer orders based on age, gender and mood. However, this was met with some resistance in Hong Kong as customers continued to line up at the cashier.
Clearly, smart kiosks are not set to dominate the food service industry just yet. The big boys will need to first sort out the knots in innovation and customer behavior, and show that it can support the bottom line before AI technology makes its way to the little guys. Only then will more investment come in.
3 – Chatbot / Conversational Interfaces
Chatbots have received some attention recently recently, not only because these allow restaurants to enhance customer relationship, but also help change customer behavior, reduce order-taking and possibly garneri expanded market share among mobile-savvy users.
In January 2017, Starbucks announced voice ordering capabilities within the Starbucks mobile iOS app and the Amazon Alexa platform. This new tool extends adds a new layer to the barista and customer interaction, even before customer reaches the store.
For sure, it is a win for Starbucks in terms of technology innovation. But other than the press release, no numbers have been released to show this new interface has gained traction with customers.
Domino’s pizza chain rolled out its Facebook Messenger chatbot in Feb 2017, as an alternative to ordering via the phone or online. Prior to this, Domino had also introduced the pre-ordering via the AnyWhere platform, Twitter, Apple TV, Google Home, Amazon Echo, Ford Sync, SMS, Samsung Smart TVs, smart watches, an in-app voice assistant, and more. Likewise, Subway in April 2017 launched a chatbot at more than 26,500 domestic locations.
While high-ROI chatbot applications are the exception (our research seems to show that most chatbot initiatives are done as a PR stunt, or never produce meaningful returns), Domino’s seems to be among the few who are driving the technology forward. Domino’s also has the benefit of a limited menu size, and many repeat orders of simple favorites – making the programming and training of a chatbot more easily achievable.
How long before chatbots and other low-ticket applications become common for ordering food, delivery or otherwise, is anybody’ guess. What is clear is that large companies will mostly have the budget and data volume to build more effective bots and that chatbots are applications that are already in customers’ hands rather than developed by the food service businesses.
4 – Customer Recommendation Platforms
Consumer platforms will expectedly impact more restaurants in the next two to three years, but “adapting” to these apps probably won’t be much different than when restaurants learned to make “findable” listings on Yelp, Google Maps, and Halla.
For SMBs that want to be involved in customer recommendation technologies, it is imperative that they explore ways to achieve better reviews and ranking from customers to ensure they show up in the platform’s recommendations.
5 – Analytics Solutions and AI
There is almost no hype or current applications of analytics solutions in the food services space, but it might not be long until restaurants partner with technology companies to use predictive analytics to help forecast visitor traffic, food orders, inventory needs, as well as revenues and costs. Among the companies that provide analyics services:
- Venga offers solutions that collect, analyze, and use data related to purchases, preferences, and habits to enrich the dining experience, personally engage customers, and ultimately increase repeat visits and sales.
- OpenTable connects diners with restaurnts by allowing to find a restaurant and reserve a table
- UpServe’s restaurant management platform is for easier payment processing, point of sale, reliable payments and in-depth analytics that can boost margins and save valuable resources
- PosIQ provides cloud-based Big Data solutions that collect customer data in real-time to help improve restauarnt businesses and customer experience.
Final Insights for Restaurant Business Leaders
In conclusion, AI is not expected to make its way into SMB food services in the near term. Some marketing automation tools will may integrate AI in the next two to three years, and some consumer recommendation apps will use AI to connect users to restaurants, but SMB food services leaders are unlikely to get their own hands on AI software (due in part to the challenging nature of these technologies, the cost of related AI talent, and the huge volumes of data required to make them work).
Meanwhile large firms will continue to explore viable AI applications that can leverage big company data, the results of which will eventually trickle down to SMBs. For now, AI applications in food services will use currently available consumer technologies, rather than in the hands of restaurants.
We don’t recommend that SMBs jump into the AI bandwagon just because it seems cool and “everybody is doing it”. This is an illusion, and a waste of time and resources. In truth, 95+% of SMB food services companies will be “late majority” adopters on the adoption curve, with only some in the “early majority”.
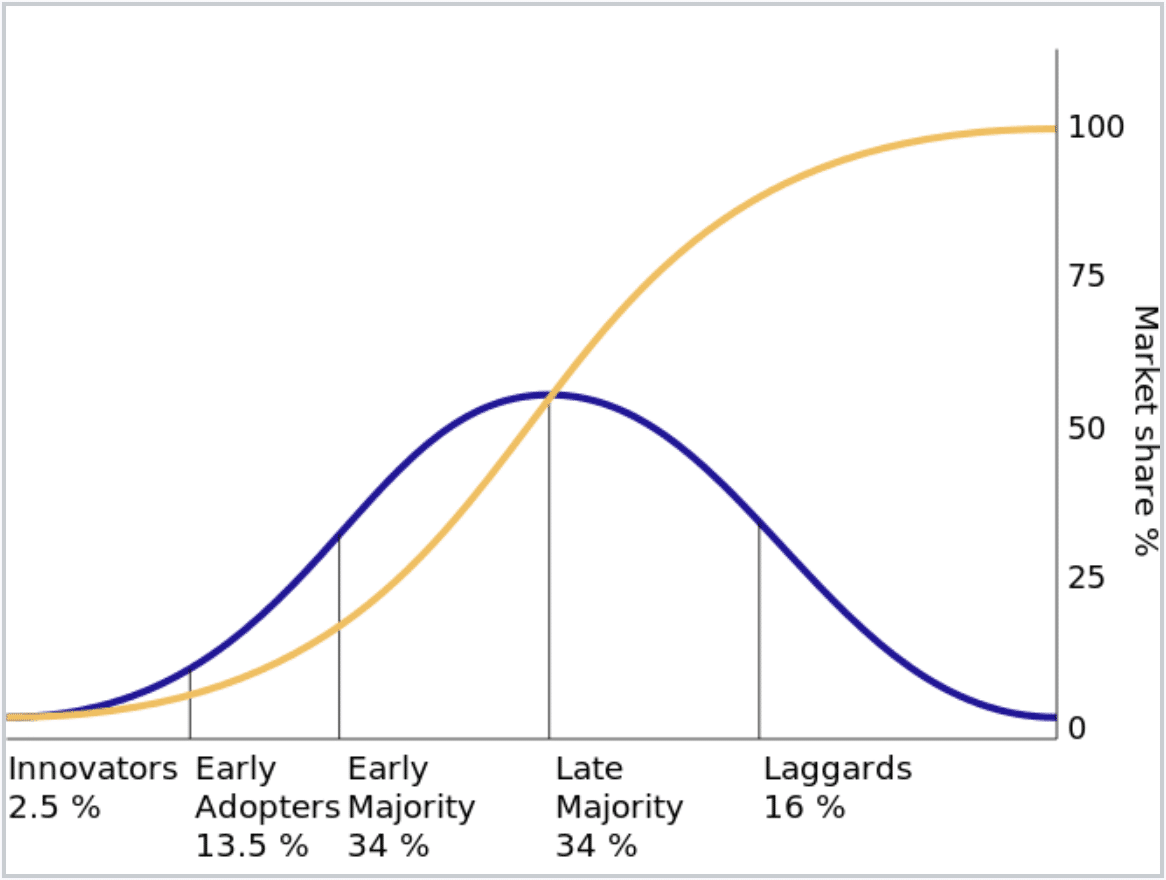
Here are some steps that food services leaders could take with respect to AI now:
- Consumer Apps May Be Relevant Near Term: Be mindful of and to carefully explore apps and consumer platforms that are becoming common or gaining popularity, and to allocate reasonable resources to those that might deliver value to your firm. Apps like Yelp (and it’s newer competitors) will likely become more effective recommendation channels.
- Most AI PR is Hype: Understand that most AI press releases are hype, and that most AI applications in food services are merely very expensive pilot programs, usually with little direct evidence of ROI. It behooves companies to seem “innovative”, and there is a felt social pressure to put on this appearance when their competitors and doing the same.
- Follow the Giants of Your Industry: Follow the big food services giants (McDonald’s, Subway, etc) to stay on top of where machine learning is becoming an important part of their business. Once an AI application begins consistently driving ROI to the biggest players in the industry, then we might expect the same technologies will become viable to the mid-size market. The best view to the future of AI in the food services sector will probably be looking at the successful AI applications of the industry giants. Until it works for them, there’s no good reason for small business owners to be concerned about them.
Related Food Services Articles:
- Fast Food Robots, Kiosks, and AI Use Cases from 6 Restaurant Chain Giants
- Examples of AI in Restaurants and Food Services
This article was written by Ayn Veronica De Jesus from an audio transcription of Dan Faggella’s presentation on “AI in Food Services” for the Ohio Restaurant Association.
Header image credit: CNBC