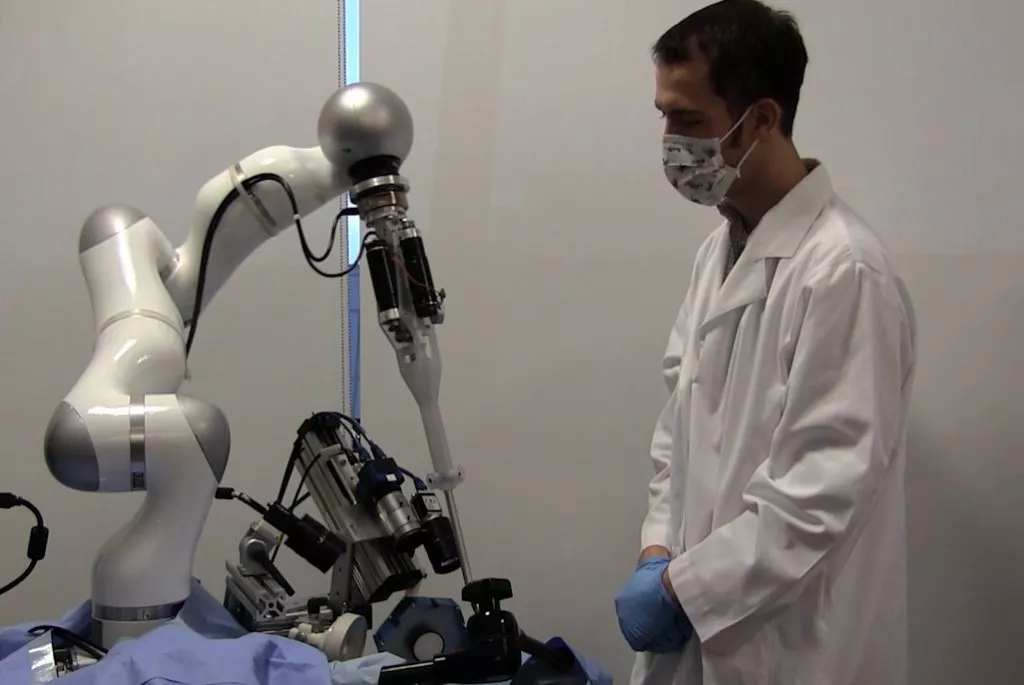
The application of robotics in surgery has steadily grown since it began in the 1980s. In contrast, the integration of artificial intelligence in this sector is still fairly new. As promising applications, predominantly in the research and development phase, begin to the surface we aim to answer the important questions that business leaders are asking today:
What types of AI applications are currently being explored in the field of surgery?
What innovations have the potential to change the industry over the next decade?
What robotic surgery applications are currently showing results?
In this article, we explore current and “near future” examples of artificial intelligence applications in surgical robotics. Based on our research, most related applications fall into the following four sub-categories:
- Automation of Suturing
- Machine Learning for Evaluation of Surgical Skills
- Machine Learning for Improving Surgical Robotic Materials
- Machine Learning for Surgical Workflow Modeling
Below explore examples across these four sub-categories. Each provides a snapshot of how AI and robotics are converging within the surgical specialty.
(Readers with a broader interest in robotics developments may want to read our complete article on machine learning in robotics applications.)
Automation of Suturing
Raven Robot and PR2 Robot
Suturing – or the process of sewing up an open wound or incision – is an important part of surgery but it can also be a time-consuming aspect of the process. Automation can potentially reduce the length of surgical procedures and surgeon fatigue. This may be particularly significant in remote or telesurgery, where any lag between human surgical commands and robot responses can present complications.
In 2013, a team of researchers at the University of California at Berkeley published research on the application of an algorithm for automated suturing performed by robots. The algorithm was tested and simulated on two robot models: the Raven II robot and the PR2 robot. The Raven robot is designed for laparoscopic surgery while the PR2 platform appears to be adaptable across various robotic applications.
The Berkeley research team reported an overall success rate of 87 percent of successful suturing. However, increased complexity of the suturing scenarios tended to correspond with decreased robot accuracy.
These results are encouraging considering the fact that suturing has been identified as a key factor limiting the use of laparoscopy among surgeons. This is despite the fact that the clinical benefits of laparoscopy have been well documented and include “decreased complications, mortality, and [hospital] readmission rates.”
In the 1:50 video below, the research team demonstrates experiments conducted using the PR2 robot.
While partially automated tools are already on the market, the performance limitations of the robots mentioned above (in terms of complex suturing scenarios) strongly suggests that it will be some years before complete automation is achievable in surgeries performed on humans.
STAR Robot
Fast forward to 2016, Johns Hopkins University announced that one of its researches was part of a team which developed a robotic surgical system called STAR or the Smart Tissue Autonomous Robot. The system integrates 3D computer imaging and sensors to help guide the robot through the suturing process.
In the 2 minute video below, scientists from the research team provide an overview of how STAR works:
Using a pig model, the robot’s performance was compared to the work of five human surgeons in three different procedures: “open surgery, laparoscopic and robot assisted surgery.” Overall, the researchers reported comparable or better results to standard surgical performance.
An estimated 44.5 million soft tissue surgeries are performed annually in the U.S. In the case of colorectal and abdominal surgeries, complications such as “leakages around the seams” occur in roughly 20 to 30 percent of cases in human surgeries. Researchers are hopeful that innovations like STAR will help to reduce these complications.
Currently, it is uncertain when STAR will be brought into hospital operating rooms. However, the researchers believe that the robotic surgical system can help reduce operation errors and improve patient outcomes.
This research presents encouraging potential for automated suturing, however, extensive additional research will be required before we will see applications applied to human surgeries.
Machine Learning for Evaluation of Surgical Skills
The evaluation of surgical skills has traditionally been a subjective practice often conducted by other trained surgeons. As robotic technology becomes more commonly used in surgeries, researchers are exploring automated methods of measuring surgical technique.
A study presented at the 2016 World Congress on Engineering and Computer Science discussed using machine learning to evaluate surgeon performance in robot-assisted minimally invasive surgery.
The research team evaluated data collected from suturing performance and classified surgeons into two categories: novice and expert. The machine learning algorithm was developed to measure the following six features:
- Completion time
- Path length
- Depth perception
- Speed
- Smoothness
- Curvature
The experimental evaluation system reportedly classified surgical skills accurately in roughly 85 percent of trials. This is a promising result offering the possibility of more standardized evaluation methods. The researchers suggest that future research efforts should expand evaluation methods to other surgical techniques and larger data pools.
Machine Learning for Improved Surgical Robotics
In the case of neurosurgery where particularly sensitive maneuvering is required, robots often lack the necessary dexterity to operate effectively and prevent injury. Researchers at the University of California, San Diego (UCSD) Advanced Robotics and Controls Lab are exploring machine learning applications to improve surgical robotics.
As depicted in the image below, “continuum robots” are made of flexible robotic material and serve as a core component of minimally invasive surgeries.
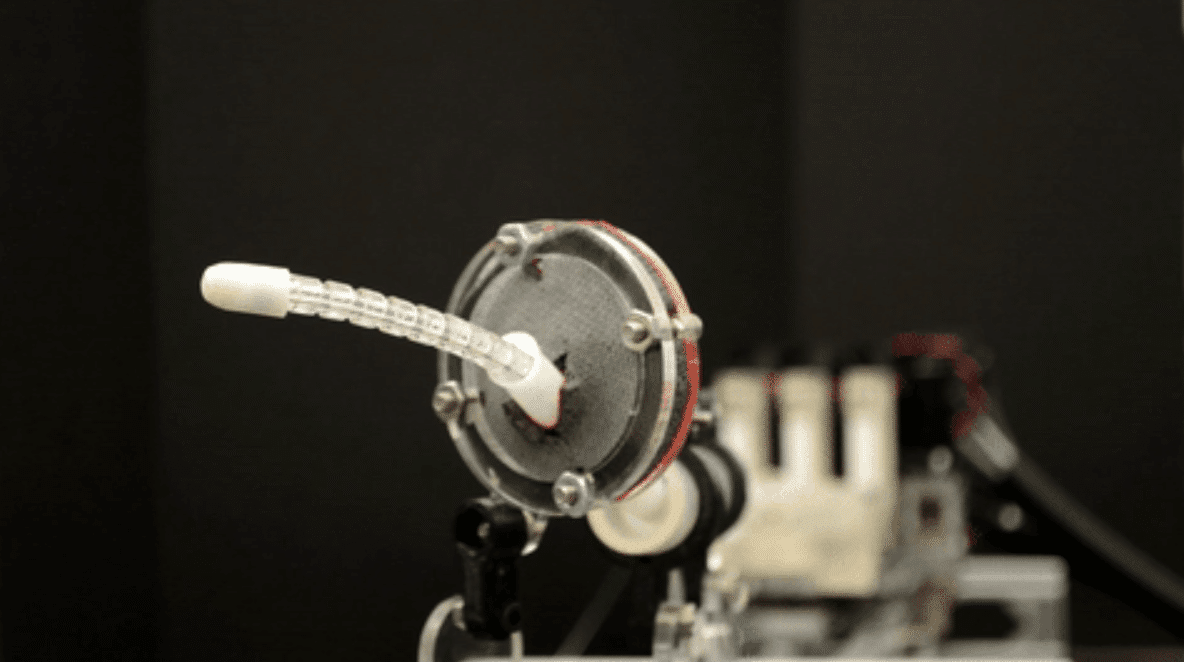
Automation works particularly well for routine processes. However, the surgical environment is not always predictable which negatively impacts the reliability of continuum robots. As a result, researchers are exploring ways to help these robots successful navigate a more complex environment.
Machine Learning for Surgical Workflow Modeling
The interest in Improving efficiency in surgery extends beyond the operation table and translates to the pre- and post-operative experience; both for the patient and the surgical team. The rate of complications in surgery range from an estimated 3 to 17 percent. One study showed a 119 percent increase from $19 626 to $36,060 in average hospital costs associated with patients who experienced complications.
Checklists have been suggested as a strategy to help mitigate avoidable errors and now automation is also being considered as a potential tool for improved surgical workflow.In an effort to improve how clinical reports are processed, a team of researchers developed a clinical information extraction system called IDEAL-X.
The manual process is often time-consuming and does not provide automatic user feedback on how to improve the process. The IDEAL-X adaptive learning platform uses machine learning to understand how a user generates reports and to predict patterns to improve speed and efficiency of the process. The 4 minute video below provides a demo of how the IDEAL-X system works.
In the study, the researchers reported that the system was “highly effective” and achieved an accuracy rate of 95 percent compared to two other methods of clinical information extraction. The researchers conclude that no advanced skills are needed to operate the system, it will be freely available online and that it is very adaptable to improving its performance. These factors position it well for clinical use.
Concluding Thoughts
Potential applications of machine learning in the surgical field are diverse and address multiple points along the surgical spectrum including training, operations and clinical data management. Innovations which can prove their worth over the long haul by consistently saving surgeons time and hospitals money will be most successful.
For example, the machine learning-based IDEAL-X clinical information extraction system has potential for implementation earlier than other applications covered in this article due to its lower learning curve, and usefulness across multiple medical specialties.
In comparison, we would expect automated suturing robots to undergo an extensive testing, review and market approval process which could take years to complete. Costs associated with training surgeons how to use the robots must also be considered.
The current lack of data makes it difficult to predict the length of time that would be required become fully competent in operating tools such as a suturing robot. In fact, certain industry experts have suggested that it may take up to two decades before we see AI fully integrated into the surgical field.
Among the challenges to consider is how AI would work in the surgical environment. Machine learning thrives on robust, abundant data and leans toward pattern recognition. The complexity of surgery often creates a far less uniform and quite unpredictable environment, the very opposite of the ideal AI situation.
Surgical robots may never have the same degree of repetitive feedback and constant wide-scale use as is seen in industrial robotics. Similarly, unlike surgical robotics, industrial applications aren’t always posing a direct threat to human safety, and so they can allow for more open trial-and-error (another significant learning advantage for industrial applications).
Thus, the research occurring in the continuum robots space is of particular significance in crafting tools that are smart, reliable and adaptable to an unpredictable environment. This approach should also inform the kind of data that are selected to train AI models.
Header image credit: PBS