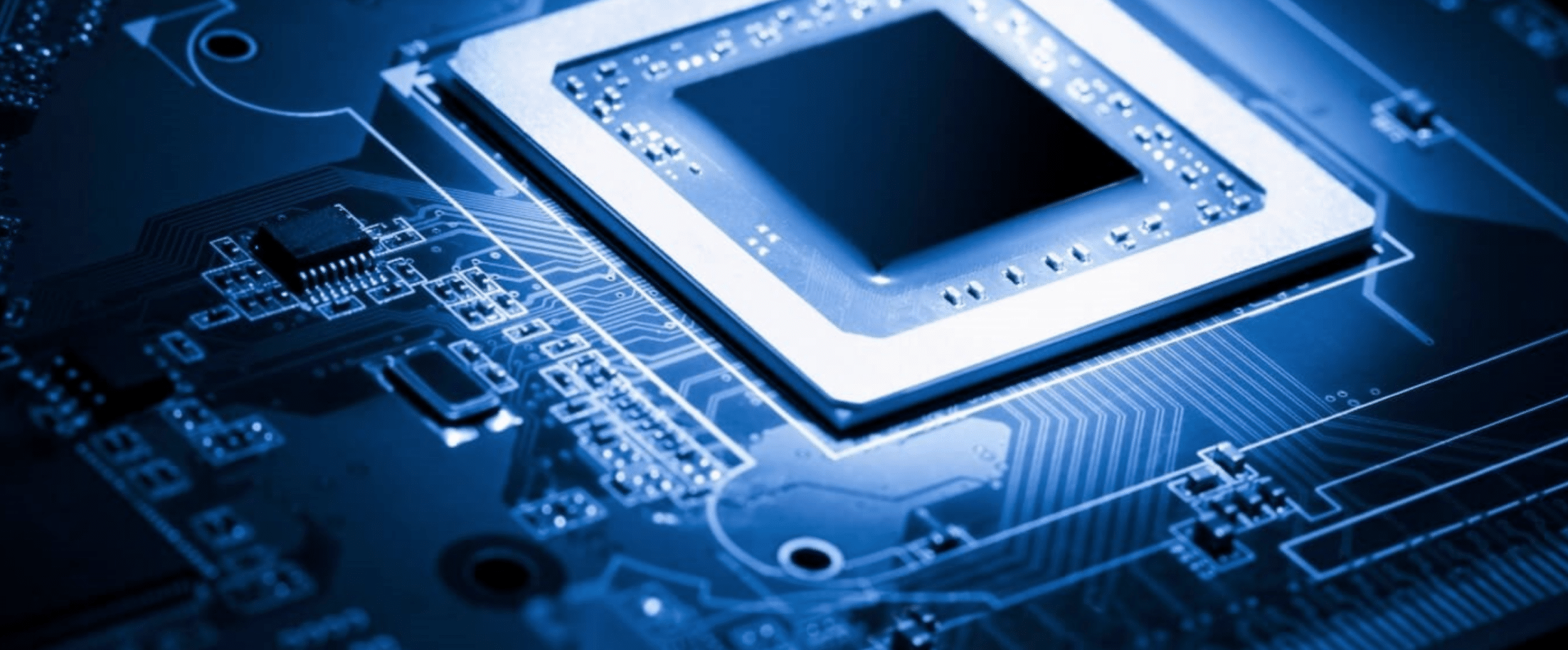
Many products today leverage artificial intelligence for a wide range of industries, from healthcare to marketing. However, most business leaders who need to make strategic and procurement decisions about these technologies have no formal AI background or academic training in data science.
The purpose of this article is to give business people with no AI expertise a general guideline on how to assess an AI-related product to help decide whether it is potentially relevant to their business. These guidelines are not meant to replace the considered opinion of an AI expert. Whenever possible, business teams should involve in-house AI experts in procuring or developing any AI-related product.
However, these guidelines can help a business leader form an informed opinion about an AI-related product and determine if it is worth further investments of time from the IT team, or outside consultants.
After reading this article, a business leader should have an understanding of (a) what specific benefits their company might expect from the AI product they’re assessing, and (b) whether or not it’s worth moving forward to a deeper investigation of that product.
The guidelines provided in this article are based on hundreds of interviews with AI executives and consultants, and over a dozen interviews with IT procurement professionals.
I’ve broken this article down into the following sections:
- Question 1: How does it work?
- What data is used/how is it used?
- How is artificial intelligence used?
- What is the integration/installation process?
- Question 2: “What have been the results?”
- Looking for proof
- Beware of being the guineapig
- Examining AI case studies
We’ll start off by asking the most important first question:
Question 1: “How Does it Work?”
Understanding how an AI solution works implies having an understanding of the role of data, the use of AI, and the integration process involved in actually putting the solution to use in a business.
Without the proper kind data – or the proper amount of data – a machine learning product cannot deliver a result. For this reason, having an understanding of the data required to train an AI solution is critical in assessing whether it might suit one’s business needs.
What Data is Used / How is it Used?
Any business product or solution purporting to leverage artificial intelligence and machine learning will need data. AI uses data to identify patterns, which in turn may be used to predict outcomes or to find a solution to a problem. Any company buying into a product will have to provide the data to train the product.
When trying to find out how an AI-related product works, the first thing to determine is the kind of data required and how it will be used.
The source of data required for an AI-related product will generally fall into two categories:
- Outside Data – Data from the AI vendor or service provider (usually sourced from other customers, or from a proprietary source), and/or
- Inside Data – Data from your own business which is provided to the vendor in order to train their algorithm
Understanding “Outside Data” AI Applications
Some AI-related products will have some degree of optimization already baked in based on the data collected from previous clients, so the data comes from a pool of sources.
For example:
InsideSales – a sales enablement company – takes sales data from all of its clients and uses that data to help any new client to generate more sales and leads. A tool like that would take the sales data from all the companies in a common industry to identify certain patterns to improve and optimize sales and marketing decision for a new client in the same industry.
Because the AI component in the product already has a certain amount of training from the data of past and current users, a new client may be able to get valuable sales insights immediately. The new client will also be feeding their own sales and marketing data when interacting with the product, which in turn improves the artificial intelligence engines to benefit all users. This encourages the “winner-takes all” dynamic of AI business models which we covered in our article called: Should my startup be using AI?.
Other “outside data” might come from sources other than previous clients. For example, a medical diagnostics provider may have its solution trained from a proprietary dataset via a partnership with a large hospital chain. In this case, smaller clinics or healthcare facilities could leverage that proprietary pool of training data without ever having to train the system on their own data.
Understanding “Inside Data” AI Applications
Other AI-related products may not be able to use that type of data pool, however.
Many (some might argue most) business applications must be tailored to the specific business that is using them – thus requiring the product to train on their own data – rather than on a past pool of data alone.
For example:
A company that manufactures motorcycle engines may need a predictive maintenance artificial intelligence application. Because the engine design is unique to this company’s brand, the AI solution can only be trained on data provided by the motorcycle engine client themselves. Data from other predictive maintenance clients wouldn’t translate to the unique patterns that need to be picked up in this specific brand of motorcycle engine.
It cannot use data from other clients because it will not be compatible with these unique machines. The manufacturer will have to train the AI application from the ground up and build the data over time to a point where the application can reliably predict breakdowns and other issues with the machines.
In this second scenario, it is important to note that it will take a significant amount of data to get any value from the AI application. Because the data is limited to one source, it will take considerable time, testing and adjustments to make the system ready, perhaps taking many months. It will also fall on the buying company to prepare the data and build the infrastructure to make it usable with the AI application.
As we addressed in our primer article about AI enterprise adoption, the cost and time of data cleaning and organization is often underestimated by business leaders. A previous interview with machine learning consultant Charles Martin explores more details about the unique data preparation challenges faced by companies looking to adopt AI.
Another factor to take into consideration is the type of data an AI product will need. This will depend on the kind of AI product under consideration. A company may be able to determine this by asking the vendor company, looking through the website, or simply using common sense.
For example:
A marketing application designed to optimize Google AdWords campaigns will need several streams of data. This may include data from the Google Analytics account, company site analytics, sales transactions, and CRM software. All these data streams may help the AI solution identify patterns of buying and site interaction behavior. This will help the company make paid advertising decisions that will result in better returns on investments, so all these data streams should go into the AI product.
Is the Data Accessible?
Assuming identification of the required data streams is successful, the next step is to find out if that data accessible. Is it in a format or structure that is going to be readily accessible for streaming into this artificial intelligence marketing application?
This is an important consideration because even if it is possible, it may take considerable time and resources to prepare the data if it is not already in an accessible form. A company considering a particular AI application should first consult with IT or AI experts to determine if the required data is available and/or accessible before making a procurement decision, and the resources involved in making it so if it is.
There may be cases where an AI solution requires types of data that are not currently being tracked or stored, which might make the adoption of that AI solution impossible.
How is Artificial Intelligence Used?
If the vendor company has a legitimate product, the answer to this question should be quite clear. Using the previous example of the predictive maintenance application, artificial intelligence may be used to collect data from heat, vibration sensors, or motion sensors to determine the patterns that tend to lead to the healthy functioning of equipment and those that lead to breakdowns or other issues in the manufacturing environment.
The role of AI in this product should be clearly stated in the website or product description. Through our assessment of thousands of AI companies, a clear pattern has emerged from our analysis:
Companies that legitimately use artificial intelligence to solve a business problem are generally good at explaining how AI is used in their product in a way that business leaders can understand. Companies who merely claim to use AI – or who use AI but haven’t found a way to consistently deliver value to clients – are often unable to articulate a clear value proposition on their website.
By no means am I suggesting that the above statement is the only litmus test you should use to pick a vendor, but we find it to be a consistent quality signal. Readers with a deeper interest in finding real or fake AI solutions should read our full article titled Separating Artificial Intelligence Hype from Reality.
The same applies to the marketing solution above. A company looking to optimize their marketing strategy should consider AI-related applications that clearly state how AI will help accomplish this in the vendor company website or product description., It should be specific about where AI is leveraging the data to make or inform a paid advertising decision. A call to the vendor company may reveal this information, but it is definitely a negative sign for its credibility if the website does not clearly explain the role played by AI in a product.
What is the Integration / Installation Process for This Solution?
The last thing a business leader needs to consider when assessing an AI-related vendor company is how installation and integration will be carried out. This includes:
- The data processing and cleaning require ahead of time
- Any new kinds of data that will have to be tracked, organized, or sorted in a new way to make this solution work
- The kind of subject-matter expertise required to help program and set up the solution
- The amount of in-house data science expertise needed in order to make the solution work
- The kind of ongoing maintenance required to keep the product functioning once integrated or installed
- Etc…
This information is often challenging to find on the vendor company website. As a first level of inquiry, it may be possible to imagine or get some sense of what it will take to install or integrate an AI product based on its purported function, but it could be costly speculation if such ruminations turn out to be wrong.
The second level of inquiry may be to ask the vendor company for a walkthrough.
A third level to find out reliable information about integration and installation of a product is to find and interview current or past users of the product (i.e. previous buyers of this same solution). These people have already gone through the process and may be able to provide valuable insight from a client point of view.
This can be a critical step in assessing whether an AI-product can provide value to a company. For companies who are new to AI or data science, we highly recommend speaking to multiple existing customers before procuring an AI solution. Since AI products are often very different from normal software or IT procurement, garnering experience from other buyers is critical.
Question 2: “What Have Been the Results?”
The second major question for assessing an artificial intelligence product is determining the track record of the product itself. The easiest way to find this information is to check the vendor company website for evidence that the product worked for past and current users – this may appear in different forms.
Looking for Proof of Use, and Preferably Case Studies
One form is a mention of working with several companies without specifying name or brand. It may be something that sounds impressive such as a “multinational company” or “global bank,” but neglecting to name names is not a good sign. The company might have such clients, but they have not established a relationship with them that would allow them to use the name on the website, which is tantamount to the same thing as not having clients.
Another form is the inclusion of client logos on the website, but nothing else. This is a slightly better sign than generic mentions of companies are, as misappropriating company logos can lead to legal issues for the vendor company. However, this does not actually provide any more information regarding results, and may simply be evidence of a pilot program (or worse, simply having a friend who works at this “client” company who has agreed for the use of the logo as a misleading favor).
The best type of evidence a vendor company can put on the website to show results is case studies. A proper case study should include the following elements:
- The name of the client (no generic or vague mentions, we need real brands and logos)
- A description of the problems and issues that the client had
- The actions taken by the vendor company to address these problems and issues
- The quantifiable results of the product’s use
A vendor company that willingly provides actual results with real companies is most probably trustworthy, and the best way to do that is to have a good number of robust case studies on its website.
Beware of Being a Guinea Pig if You Can’t Afford to Be One
If the vendor company website does not provide any information on past or current clients at all, it is possible that the company is in its early stages, without any proven results thus far to speak of. It has not yet had a chance to deliver results for their product in the real world, and is looking for their first client as a pilot. This does not automatically mean the product is not a good one, or that the vendor company is a bad one. This just means that there is no evidence either way.
If the vendor company and the product seem to be on the cutting edge, it may be worth considering doing a pilot as long as the company provides the product free of charge. Pilot studies often yield poor results because the product is not tested, and it may take much longer to get any type of results. It would make sense to take a chance on an AI-related pilot if the buying company has reason to trust the vendor company providing the product without charge.
It is also worthy to note that some vendor companies may not be able to provide any information on their clients because they provide highly sensitive services, such as data security. Very few such companies can collect testimonials from their clients. The clients would not want to reveal which data security company they use because it will make them potentially vulnerable to cyber attacks. It may be possible to talk to the vendor to ask for private access to case studies is such situations.
The point is, while it is not a good sign when case studies are not available from the vendor company, it does not necessarily mean positive results are not possible or available. However, generally speaking, it is safer for a company to consider a vendor company of AI products that have some evidence of positive results, and the more evidence available, the better. This is especially the case for companies lacking AI experience, or expert AI talent in-house.
Additional Notes on What to Look for in an AI Case Study
It is also important to mention the need to look more closely at two elements of case studies. There are the details of integration and installation, and results.
Here at Emerj has a whole section of our platform for in-depth AI case studies, and we’ve spent the last two years determining the best format and the critical factors to making case studies trustworthy and useful for our global audience of AI-focused business leaders.
Before uploading a case study, each one goes through a thorough vetting and investigation to determine its value. In almost all of these case studies, the descriptions of these two elements are often vague or misleading. Most vendor companies will not want to reveal the hard details of integration and installation, which often involves considerable resources. These companies may also provide a more flattering picture of the results than it is in reality.
When a company finds a vendor company with good case studies, it is important to inquire more specifically on the details of integration and installation, especially if they make it look simple. Integration and installation of AI products is almost always never simple, so it is crucial to peel away the layers of glaze to learn more about the actual process and any issues that may come up.
A company looking to buy an AI product should also pay special attention to the results claimed in the case studies. Never take them at face value.
In most cases, vendor companies will try to word it in such a way that it seems as if the product produced positive results. However, a closer look may show that hard numbers are missing or misleading, and that will not help with decision-making. Any positive results should specifically indicate the role the AI product played in producing those results, and provide the hard proof of such a claim.
Buying companies should make the time to get a clear and concise version of the use case, not the enhanced one. A thorough vetting is in order to get much more detail about the granularity of the results and details of integration. When pressed on these two issues, vendor companies will generally provide a true picture. While they may be considerably less palatable, they will provide a clear picture of the reality. This is essential for an accurate assessment of any product, especially an artificial intelligence product.
Conclusion
Artificial intelligence is in its very early stages of finding successful business adoption. For the most part, it remains a complex technology that requires skills and technical knowledge that simply isn’t required for most other IT procurement decisions.
For that reason it is important to assess the value of AI-related products carefully – and this is especially true for companies who are new to AI. These tips and general guidelines should be useful for business owners with no AI knowledge to do an initial assessment. We hope you’ll find them to be useful.
Header image credit: TechSpot