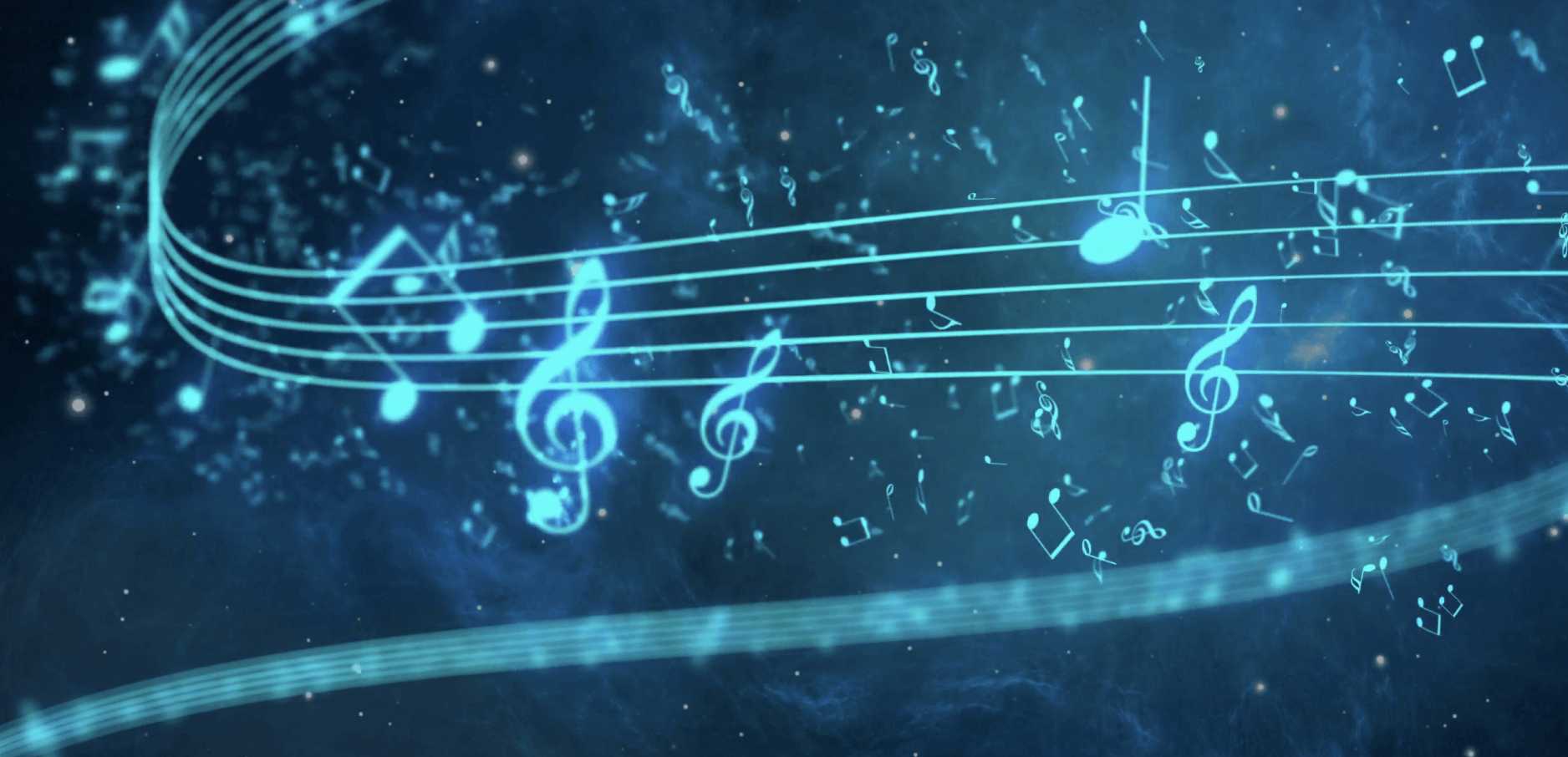
The U.S. music industry is an economic staple generating an estimated $7.7 billion in retail revenue in 2016, according to the Recording Industry Association of America. The year 2016 also marked the first time that music industry revenue was generated primarily from “streaming music platforms.”
To gauge the emerging role of AI in the music industry, we researched this sector in depth to help answer questions business leaders are asking today, including:
- What types of AI applications are currently in use in the music industry?
- How has the market responded to these AI applications?
- Are there any common trends among these innovation efforts – and how could these trends possibly affect the future of the music industry
In this article we break down applications of artificial intelligence in the music industry to provide business leaders with an understanding of current and emerging trends that may impact their sector. We’ll begin with a synopsis of the audio AI application areas we cover in this article:
AI Music Applications Overview
Based on our assessment of the applications in this sector, the majority of music industry AI use-cases appear to fall into three major categories:
- Music Composition: Companies are using AI to create, enhance, and/or complement music content.
- Music Streaming: Companies are using machine learning and deep learning to recommend personalized content based on data from user activity.
- Music Monetization: Artificial intelligence platforms are helping artists monetize their music content and generate revenue.
In the full article below, we’ll explore the AI applications of each application by section and provide representative examples and videos.
Music Composition
Popgun
Launched in January 2017, Australia-based startup Popgun reportedly uses deep learning through a platform called ALICE to accompany or augment musical compositions. The startup’s team, mainly composed of software engineers, argues that the goal of their work is to foster collaboration and “not replace human abilities.”
The company has provided a few demos to demonstrate how ALICE functions. Examples include the following:
- ALICE trying to predict what a musician will play
- ALICE accompanying a musician as they play
- ALICE attempting to improvise upon what a musician plays
Using deep learning, neural networks are trained on thousands songs, varying across multiple genres. This training method allows the networks to interpret the style of a given musical composition, and ‘play along’ in a similar beat or pattern intended to complement or complete a melody played by a human user.
At present, it appears that most of Popgun’s current applications are being trained with an electronic keyboard. According to Popgun’s website, in the future, the company envisions that AI will collaborate with musicians to teach them how to play different instruments faster than currently possible and to enhance music production capabilities by offering new sounds.
It appears that the the team is currently in fundraising mode and no client profiles or case studies are available at this time.
Amper Music
Amper is a cloud-based, AI-driven music composition platform. The system reportedly generates unique musical selections based on the mood, style, and duration parameters selected by the user. Once these selections are made the user can make additional edits before the composition is complete. The company claims that the platform is built on data from a library of music samples.
Amper aims to provide individuals with no musical training and professionals alike, with the ability to quickly compose original music. First, the algorithm is trained on data from a large collection of musical tracks across a wide range of genres. The algorithm can then identify key components of each musical composition and predict the type audio a user wants to create.
In the short 1:33 minute video below, company founder Drew Silverstein provides an overview of the Amper platform explaining how users can generate musical content.
Quite similar to Popgun’s team, Silverstein also emphasizes his interest in using his platform to collaborate with musicians, enhancing their ability to compose music. Venture Capitalist firm Foundry Group was among three investors contributing to $4 million in seed funding.
Music Streaming
Spotify
Recommendation engines are one of the most common AI applications for consumer tech. Leading music streaming platforms rely on the underlying algorithms to suggest content based on user history and activity.
Spotify reportedly combines a deep learning approach complemented by a process known as “collaborative filtering.” Collaborative filtering is a method of classifying information by using the recommendations of other people. Data from the recommendations of a selection of users (who share similarity with the user of interest) is gathered and a weighted average is calculated to make a prediction about the user of interest.
The music platform generated an estimated $3.3 billion in total revenue in 2016, marking a reported 52 percent increase over the previous year. However, with royalty and distribution expenditures nearly 85% of the company’s revenue, profitability at scale seems to be a moving target.
Readers interested in a deeper dive into recommendation engines should reference our previous article Use Cases of Recommendation Systems in Business – Current Applications and Methods.
Pandora
Since Pandora’s originally launch back in the year 2000, today, the internet radio platform claims it has over 1.5 million analyzed songs through its Music Genome Project and uses over 60 recommendation strategies for song selections.
According to Staff Scientist Erik Schmidt, research and machine learning continue to be at the core of the company’s business strategy:
“One of the most fascinating areas of research at Pandora is ‘Machine Listening’. In the music domain, we seek to develop systems that are capable of automatically understanding the musicological content of an audio signal. These systems rely heavily on supervised machine learning, and Pandora’s Music Genome Project provides the largest and most detailed corpus in the world for performing this work, spanning over 1.5 million analyzed tracks. As a result of this dataset, we have been able to develop incredibly rich and accurate machine listening representations.” – Erik Schmidt, February 2017
Pandora’s founder Tim Westergren explains the original vision for the Pandora Genome Project (and how the system was painstakingly trained by human music experts back in the early 2000’s) in this interview video below. The first 5 minutes are the most relevant:
Above (starting around the 6-minute mark), Tim also explains that personalization will be the differentiating factor that sets Pandora ahead of its competitors. His description of how the company is approaching recommendations, however, doesn’t seem drastically different from what Spotify and other streaming services do. The company’s stock price has dropped from around $38 to around $9 in the last two years, and the board seems to have moved Tim out of the CEO role and off the board altogether.
In June 2017, Pandora announced a strategic alliance with SiriusXM accompanied by a $480 million investment in Pandora and an estimated 19 percent share of the company. It has been suggested that the move is part of Pandora’s efforts to improve its competitiveness against Spotify.
Sustaining a profitable revenue model seems to be a challenge for some music streaming services. The company reportedly experienced an increase in losses from $170 million in 2015 to $343 million in 2016.
Music Monetization
LANDR
Professional quality music recording is not cheap and certain music equipment alone can reach upwards of $10,000. For the average independent artist, many technical components of the music production process are cost prohibitive. Companies like LANDR are attempting to level the platform by giving musical artists the ability to master or professionally complete their songs at affordable rates.
According to its website, LANDR reportedly uses machine learning algorithms trained on the standard steps music engineers follow to master music. The web-based platform allows users to drag-and-drop a rough track, and get an “instant mastered preview” which the company claims can be download in minutes. Mastering of tracks is free for MP3 tracks up to 192kbps in size. Three subscription packages are offered at $4, $9 and $25 per month.
The company claims that its AI-driven mastering engine is trained on “data from millions of mastered tracks” combined with a large repository of knowledge from music engineers. The algorithms classify a track based on the elements of its production style. This process also informs the post-production processing that would be best suited for the track.
The company also appears to offer distribution services to the leading streaming services.LANDR claims artists will keep 100 percent of sales and rights. A private sharing feature was recently launched to help users share their music online more safely.
The 36 second video below offers a quick overview of how LANDR’s drag and drop interface functions and briefly mentions the role of AI:
CloudBounce
CloudBounce is reportedly a fully automated mastering platform that appears to integrate machine learning which is part of machine listening. Comparatively, CloudBounce’s prices are commensurate but slightly higher than LANDR’s. It appears that one free track upload is offered as an introduction to the service.
The 2:28 minute video below demonstrates how to navigate CloudBounce to master audio content.
Concluding Thoughts on Musical Artificial Intelligence
The use of AI is expanding beyond recommendation engines and offering promise of a more accessible and democratized industry for professionals and nonprofessionals alike. Among platforms aiming to help automate the process of developing audio content, there seems to be a deliberate emphasis on collaboration.
The designers of these platforms seem to understand the importance of ensuring audio content creators that these innovations will not replace them but rather assist and improve their process. Otherwise, we would expect that these platforms would be met with a great deal of resistance.
By eliminating the need for technical training, the music composition platforms we have discussed in this article are positioning themselves to be readily accessible to clients. However, these platforms are still in the early stages and their revenue models are not yet clear. Additionally, it is too soon to say with certainty who the target client will be for these companies. These factors will inform the long-term viability of these music composition platforms.
AI is playing a role in driving recommendation engines behind leading music streaming services like Spotify and Pandora. Interestingly, profitability at scale seems to be one of the main challenges for these companies. It will be interesting to see if AI could also contribute to addressing revenue challenges.
Music monetization is by far one of the trickiest aspects of this industry. Independent musical artists face major challenges producing, protecting and bringing their music to the marketplace. The lack of data on client outcomes makes it challenging to predict the impact of these platforms at this time. Nevertheless, it is possible that we’ll see similar companies appear on the horizon to tap into this captive market. We will continue to monitor how AI continues to emerge in this sector.
Header image credit: BlockMusic