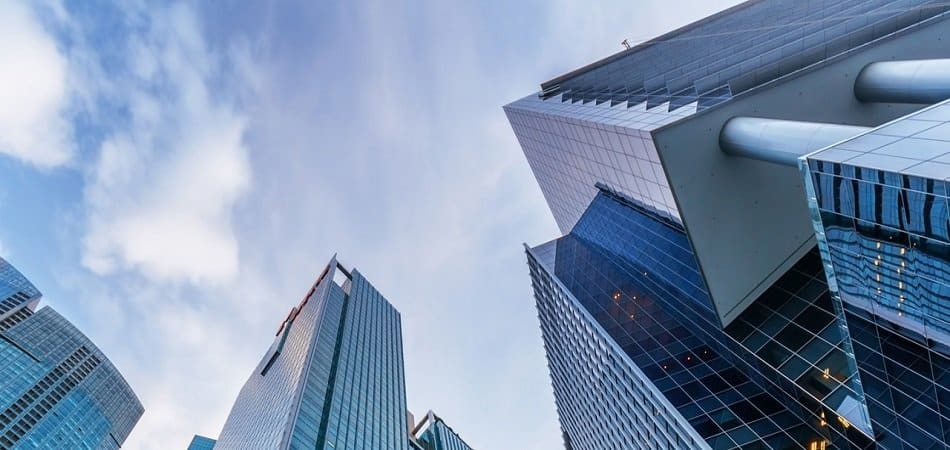
AI software for corporate banks is not too different from those for retail banks, although their data requirements and intentions for the software will differ. AI vendors currently selling to banks typically have clients covering all types of banking, but few specify any of their solutions to be for corporate banking specifically. Instead, they market themselves across the entire industry and give corporate banking details where appropriate.
In this article, we cover the AI applications that may be helpful to corporate banks. We highlight partnerships between AI vendors and corporate banks to add insight on any evidence of success they provide. If a solution would have unique data requirements for a corporate client as opposed to a retail client, we note it as well. We cover the following solutions specifically:
- Document Digitization: Corporate banks may have large backlogs of paper documents stored across multiple branches. Some banks may need to digitize all of these documents for easier searching but also to remain within regulatory compliance for the banking industry.
- Automated Accounts Receivable with Predictive Analytics: Predictive Analytics could automate a bank’s system for checking accounts receivable and attempting previously declined charges from customer credit cards.
- Fraud Detection: How banks could protect the privacy and funds of their corporate clients using anomaly detection and predictive analytics.
The first type of AI solution we cover is document digitization, and we discuss how corporate banks can more efficiently digitize documents that are typically required to be physical before entering the bank.
Document Digitization
Corporate banks can benefit from document digitization solutions similarly to other banks in that they may be able to make large backlogs of physical data more useful. Additionally, banks may want to use AI to stay within regulatory compliance or determine how likely a customer is to pay back their loans or default. That said, they may differ in which documents they need to digitize.
Corporate Banks might use AI document digitization software to add the following physical documents to their digital database:
- Financial loan agreements
- Equipment loan agreements
- Commercial Real Estate documents such as a certificate indicating a passed inspection
- Any documents from customers or clients that contain new information about any cash and treasury management issues
In order to accomplish this, a bank would need to use a type of machine vision software called optical character recognition (OCR). This must be installed into a system where a camera can scan high-resolution images of each document to detect the letters, numbers, and other characters. The software then translates these characters in the same sequence to produce the document in a digital format.
OCR software can be linked to a client’s database of customer information in order to send new information from scanned documents to the right place. This allows the bank to more easily retrieve digitized information like they would any other resource from their database.
This is most helpful for banks with a large backlog of physical documents, and this can be observed tin real estate banking. Home leases and sales typically require signatures, which means real estate bankers find themselves with many paper documents that could be more easily accessed digitally. This can also be helpful to bankers looking for a more robust loan history on a given client to gauge their fiscal responsibility before going through with a loan.
Newly implemented OCR solutions might cause a bank to have a sudden increase in digital data, which would need to be properly organized and stored within a database to be useful. This way, each department may access the new data from a centralized source. That said, this may prove to be a challenge for legacy banks using older data storage methods with large amounts of data already there. We interviewed Muriel Serrurier Schepper, Business Consultant of Advanced Data Analytics & Artificial Intelligence at Rabobank Digital Bank in Holland, for her insight on how business leaders may be able to handle this issue. In our interview, Schepper stressed the importance of making big data useful to the average employee. When organized and cleaned correctly, big data could also be used to help train a machine learning model for another AI application. Schepper highlighted the following steps for a legacy bank to make sure they can prepare this data properly:
- Hiring talent with an academic background in AI that are also disciplined in banking concepts across multiple departments.
- Making sure the AI team and those involved in AI implementation in each business department communicate frequently. This helps all employees familiarize themselves with the software and also uncovers where data needs may overlap and thus create more value.
- Identifying problems in each department so that leaders can see where workflows and practices can improve to make the AI adoption go more smoothly.
- Setting realistic expectations for the technology and the AI team. Even a skilled AI team knowledgeable about banking concepts will still need to work hard to train and implement the machine learning model effectively. Many OCR solutions are not fully automated and will need an employee to direct the newly digitized data to the right area of the database.
Vidado is a good example of an AI vendor offering OCR solutions to financial institutions. The company claims their software can review data for any exceptions to their normal process. For example,a bank could business reply card for a scheduled meeting, but not include a date because the recipient would already know it is for the same day. The system could review the reply card and allow it to enter the database without a human employee to check it because it has “learned” to make this type of exception.
Their AI platform has four distinct parts They have an AI platform that works in four key steps to bring important data from physical media into a digital format for use across all relevant business departments. We have listed these parts below:
- Vision: Human employees scan the bank’s physical documents into the system. Vidado claims the writing scanned does not need to be particularly high resolution or neatly written, but the effectiveness of this is unclear. The vision step organizes scanned documents according to pre-prepared templates.
- Read: The software captures both machine printed writing and handwriting. Vidado claims the software can recognize messy handwriting and low dots-per-inch (DPI) scans of paper documents.
- Review: This is what Vidado refers to as their “exception handling interface,” or the portion of their system that checks for incorrect or incomplete data. Banks can set customized checkpoints for these documents in order prioritize scrutiny when scanning the most important data. At the same time, less important data can be passed through without a human employee’s review.
- Transform: The final step allows banks to validate their data against the rest of their enterprise data or any third party data sources they may have access to. It can also purportedly format the data to be easily transferred through their API.
Vidado has published a customer success story on their website which details their work with MetLife on handling exceptions in their beneficiary designation forms. Many of these forms are sent to the company each day to indicate who will receive the benefits from a customer’s life insurance policy. The company had numerous forms with incomplete fields, bad handwriting, or information contradictory with the bank’s current customer information.
Vidado claims to have installed their AI platform into MetLife’s existing system, and that their exception handling interface was able to contextualize exceptions with the most relevant data. This may help a human employee whose job it is to fix these errors by providing them with the enterprise data likely to be the most helpful.
In addition, Vidado claims MetLife was able to reduce their document processing time by 93%. Additionally, manual exception handling purportedly dropped by 70%. Finally, the company saw a 43% increase in straight-through automation, or the automation of pushing data directly from its source to its intended digital location.
While this example of Vidado’s work was for an insurer, the story contains trust indicators for banks considering AI vendors for document digitization. The software’s ability to categorize scanned documents based on predetermined templates would be helpful for banks taking in multiple types of form. These could be loan applications, equipment loan agreement forms, or real estate documents.
The vendor’s focus on straight through automation of forms may also be useful to corporate banks that may need more granular prioritization of data-related tasks. The most important documents might need more time with a human employee if they have incomplete data. Although less important ones may be automatically corrected by the AI platform for later review when necessary.
Automated Accounts Receivable with Predictive Analytics
Corporate banks could use predictive analytics to automate their listing of accounts receivable (A/R) as well as their order to cash processes. This allows a bank’s system to automatically detect contractual relationships established with customers via new contracts or subscriptions to corporate banking services. Once an order is received, the system uses available data from the sale to decide where and when to fulfill and ship the order.
This can be done once the client submits any necessary payments in the bank’s A/R. If there are payments that have not been made, the system will attempt to run the payment again every so often. The client may need to be contacted by a human representative if their payment is consistently declined.
In order to accurately predict the next step in the A/R and order to cash processes, a machine learning model would need to be trained on thousands of orders from the bank’s database. Each order would be labeled with the client’s name, the product or service paid for, how much the payment was for, and when the payment was made. This would gradually train the machine learning model to discern the differences in individual accounts receivable as events.
Once the algorithm can make the distinction between a complete and incomplete payment, it can begin automating the processing of that payment and send signals to other departments to begin preparing the product for shipping. As automation technology continues to improve, corporate banks could see AI and data analytics solutions to facilitate multiple business processes at once.
We spoke to Ann Miura-ko, co-founder and partner at Floodgate, about how predictive and prescriptive analytics software could continue to benefit banks by becoming more fully automated or “self-driving.” When it comes to payment processing, this requires the software to continually determine the probability of what the next required action will be with each new data point considered. When we asked about how she came to this idea of “self-driving” AI and how she breaks that idea out into other industries, Miura-ko said:
The first time it really dawned upon me, I have a Tesla and I got on to 280 and I engaged the auto pilot and…it was this WTF moment; the piece that was really interesting to me was there’s this autopilot, where you’re actually getting a lot of direct feedback from the actual car, about what it’s seeing, why it’s making the decision that it’s making, and obviously you can override it…for me what it points to is this concept of intelligence and the enterprise needs to become more intelligent overtime, and the enterprise applications need to follow that…
Miura-Ko draws a comparison between AI-enabled self-driving cars and AI for enterprise process automation. As seen with A/R and payment processing, an AI application can automate signals that allow other business processes to start. Right now, these signals are usually for human employees. However, Miura-ko projects that these types of signals could be sent to other AI software, making for a more fully automated sequence of tasks.
Cash and Treasury Management File published a press release which covers the AI vendor HighRadius’ work with Citibank to automate their accounts receivable. HighRadius specializes in cloud-based payment processing, which helps organize a bank’s A/R. The press release states that Citibank used HighRadius’ predictive analytics technology to improve their “Smart Match” platform. This is a payment matching platform for corporate clients that helps the bank check back on unpaid A/R and attempt to run payments again.
At the time of publishing this press release, Citibank saw demand from their corporate clients for innovation in the following areas:
- Cutting costs through automation
- Lowering daily unpaid accounts receivable
- Handling any exceptions or abnormalities quickly so as to increase payment processing efficiency.
HighRadius’ platform uses predictive analytics to match open invoices with received payments from corporate clients based on past events analyzed from A/R data. According to their website, the software analyzes past A/R events to come to an understanding of when an account is paid and what to do next.
According to the press release, Citi Bank helped their corporate clients improve A/R reconciliation and its automated straight-through processing (STP) system. We can infer that Citi Bank’s STP system was also able to more accurately match customers and their required payments.
Fraud Detection
Corporate banks could use AI applications for fraud detection to detect possible fraud cases within payments, accounts receivable, and contractual agreements. Additionally, fraud detection software could be used to detect breaches in company cybersecurity. There are two approaches to AI software for fraud detection: anomaly detection and predictive analytics.
Anomaly detection software is trained in real time by installing it at a bank’s headquarters allowing it to scan each new event for data contained within. For payments, this would be thousands of individual transactions complete with customer, merchant, geolocation, and payment method information.
The machine learning model learns the content of each of these data points and how they relate to one another in real time as it analyzes each new transaction that enters the system. This allows it to come to an understanding of the general criteria of what should be in each transaction, which means it can flag those that deviate too far from the established norm.
Predictive analytics software is not trained with as close a handle on exactly what the machine learning model is scanning for. Instead, the algorithm is allowed to sift through the bank’s database and allowed to make correlations between data points as it comes to its own conclusions.
This allows the software to come to an understanding of what fraud is based on deviations that stand out from others. These include falsified customer information or faked geolocational data. This way, the machine learning algorithm can allow for more mundane deviations that are not actually signs of fraud.
We spoke to Justin Fier, Director for Cyber Intelligence and Analytics at Darktrace, about how new fraud methods continue to get more advanced. When we asked what he referred to when talking about “malicious AI,” Fier detailed how a fraudster could even use machine learning to develop and use new fraud methods:
… we’re going to continue to see hackers spearfishing campaigns with machine learning. We just recently saw a campaign where, after gaining access to a user’s email, the program looked for keywords; file names; language; the way the person types and speaks; and crafted e-mails based around that, because it gave them more of a chance of getting clicks on the other ends, and I think that kind of sets a framework of where we’re going to see ML move in the malicious hemisphere—using the machine to determine patterns of life, really intricate ways to move around internally on the network while going undetected.
New fraud methods such as these are developed alongside the advancement of fraud detection technology. It is because of this that it may be harder for legacy banks to adopt a predictive analytics application over an anomaly detection one. Predictive analytics software may be able to detect more subtle events or changes to data that would allow it to recognize a previously undiscovered fraud method.
One AI vendor for banking fraud detection solutions is Teradata. Their machine learning platform purportedly improves banks’ fraud detection rates with their data analytics software’s ability to identify potential instances of fraud while still avoiding acceptable deviations. This implies a lowered rate of false positives through the software’s ability to discern between signs of fraud and unique but acceptable deviations in banking events.
For example, if a transaction occurred very far away from where a customer works or lives, but the customer is on vacation, the software would accept this anomalous geolocational data point instead of flagging it as possible fraud.
Below is an image from Teradata’s website detailing the value of their AI solutions in improving existing business processes. This value entails recommendations and instructions on how to continue using the AI software most effectively, and easily generated proof of value reports for stakeholders and business leaders. Additionally, the company offers an open source method of developing a platform and dashboard for AI related-processes and data analytics as a service.
Teradata published a case study on their website which details how they helped Danske Bank update their fraud detection process and bring down their 1,200 per-day false positive rate. According to the case study, Danske Bank installed Teradata’s solution and integrated it into their payment processing workflow in order to:
- Remove 60% of false positive flags of possible fraud and expect that to grow to 80% as the software continued to improve
- Reduce false positives by 50%
- Shift their focus towards identifying new fraud methods and keeping their fraud detection process efficient.
Header Image Credit: bankislami.com.pk