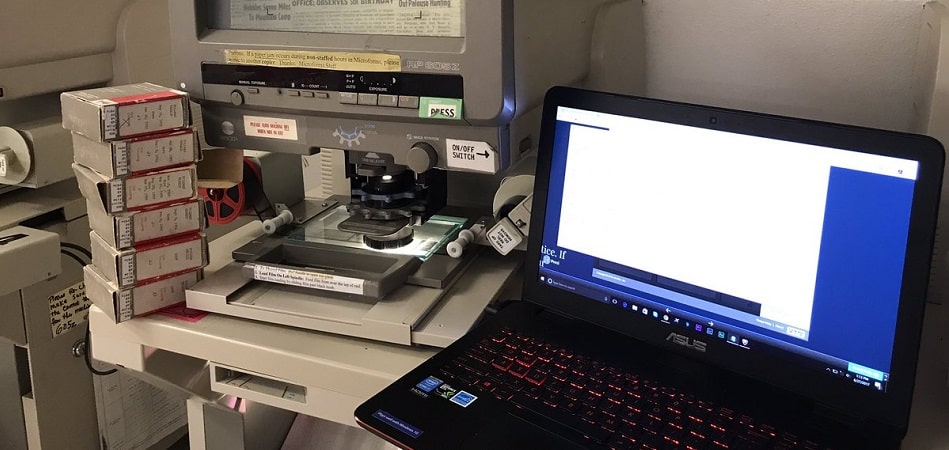
There’s an entire artificial intelligence ecosystem for enterprise search. Most of this is in a purely digital world. Most vendors help with a layer of AI-enabled search that understands terms or phrases and is able to return the results or answers to questions that someone types in. But the problem is compounded when it comes to searching the physical world.
Large banks might need to look for financial records going back 40 years to handle a lawsuit, for example, and they might have to investigate not just digital files but microfiche and printed paper in different storage facilities in various off-site locations.
This is a challenging problem, but an important one for firms that don’t want to spend two weeks locking their lawyers up in a room for them to figure out some legal issues or find certain clauses within past contracts. Banks would like to be able to search for them, whether they’re physical or digital, and find what they’re looking for much quicker.
That is the topic of this week’s episode of AI in Industry. Our guest is Anke Conzelmann, Director of Product Management at Iron Mountain. Iron Mountain is a four-billion-dollar physical and digital storage company based in the Boston area.
They handle the records of some of the largest financial, healthcare, and retail brands around the world. Conzelmann speaks with us about the future potential of artificial intelligence for search within an enterprise, not just of digital files, but across formats.
For a more in-depth analysis of AI search applications in banking, download the Iron Mountain-Emerj co-branded white paper on the topic.
Subscribe to our AI in Industry Podcast with your favorite podcast service:
Guest: Anke Conzelmann, Director of Product Management – Iron Mountain
Expertise: Enterprise adoption of AI-based search applications
Brief Recognition: Anke has been Director of Product Management at Iron Mountain since 2003. Prior to this, she was Product Manager for Dragon Systems before they were acquired by Nuance Communications.
Interview Highlights
(2:3) Where do you see most of the opportunity for AI-based search in legal?
AC: It’s actually a great use case, the contracts. Every company has them, and we all hope that we have lots of them.
Having more customer contracts is good, but it also means that we have oftentimes a very distributed set of sources. [It] could be by product…by region…It’s really hard to answer really simple questions like, “where are all the contracts for this particular customer? “It gets even harder when you think about answering questions like, “which of these customers have non-standard payment terms?” Generally speaking, now you’re starting to read contracts. Of course, that doesn’t scale.
The other problem is that you may not necessarily be sure that you caught them all. So every company has this problem, and every company could really benefit from using some of that machine learning and AI-driven classification and metadata enrichment in order to make those kinds of questions easy to answer.
(4:30) What are some other interesting use cases that aren’t going to scale with people, but searching those contracts would make sense with machines?
AC: Another really good one is actually in HR…Every customer has an HR department. Every customers’ holding employee data. So with the privacy laws that are already enacted in Europe with GDPR, that are coming in California…and in Brazil next year, this is just going to essentially become a universal requirement. The requirement is pretty hard to meet unless you actually can do these things at scale.
Where is personal information? Where do I have employee information, and can I find it? Can I produce it when the employee requests it? Can I remove it when I need to remove it?
One of the things that are changing with these privacy laws is that the “I keep everything for every retention policy” is, frankly, just not viable anymore. Oh, by the way, complying with these privacy regulations: if you can’t do it, they’re really big fines. You’re looking at 2% to 4% of revenue or €10 to €20 million for GDPR. The higher of the two, by the way, not the lower, so pretty big fines coming.
So being able to identify where personal information is in documents both physical and digital. For example, I’ve been at Iron Mountain for a long time. When I first came, there were physical pieces of paper that were filled out. Those are still sitting somewhere, but there’s also digital information that was collected when I had my review last month. So how do I go across those different repositories of information in physical and digital? Some of it may be Office documents, some of it may be images, some of it may be who knows what.
So different kinds of content, and really go across that and be able to answer the questions for each and every one of those source files. Things like, “where is the personal information?” Being able to say, “Okay, give me this employee’s personal information across all of those different repositories that I ingested from.” Finding that reliably, being able to keep it in an appropriate way from a security and access control perspective, and applying retention policies so that, if I quit tomorrow, seven years from now the retention on these documents might be up, and the company is obligated to get rid of this information when it doesn’t have a business need to keep it anymore.
Privacy in HR documents is absolutely another use case that you can’t really get your arms around with just people.
(8:00) What would be the instance where a company would need to do one of these bigger searches and dig through paper, digital, images, the whole nine yards?
AC: It’s for existing employees even. As an employee, or as a customer for that matter, you have the right to ask a company you’re either employed by, or you’re doing business with, what information they’re keeping about you. First of all, you need to be able to answer that question and answer it in a timely fashion.
The second thing is, as an employee, I now have the right to rectify. Meaning, I should be able to tell you, “Hey, this is wrong. You need to correct it.” And then I also need to actually be able to get that stuff. For me to be able to say, “Okay, Anke, here you go. Here are all of the documents that we have that contain personal information about you.” You need to be able to produce all of that information.
Then, finally, it’s the right to be forgotten, is what it’s called. That’s the piece where once there is no business, that original business purpose for which you collected the information is no longer relevant…[an employee] no longer work[s] for the company, now I have to actually destroy that information. I have to do that in a way that I can prove that I did it according to the policy.
I need to be able to show that I actually started my clock ticking from a retention perspective based on the termination of that employee. Whether they quit, or they were let go, doesn’t really matter. Then having that clock run out, and for me to have a standard process where I’m now going through and destroying the information. Whether it was physical, whether it was digital. I need to be able to do that consistently across where I’m holding that information.
That’s where, again, ingesting all of that into an end-to-end platform where I not only can take the physical information and digitize it and now potentially get rid of the physical so that I don’t have to deal with that down the line. I can take all of the information that’s coming out of digital repositories, could be file shares, could be SharePoint sites, HR shared drives, and pulling that all into a common repository so that I can then very easily comply with what I need to do from a policy perspective.
That’s another benefit of finding all of this stuff, being able to ingest it all, being able to enrich it, pulling out key information like, “what employee’s this document for? What kind of document is it? Does it have personal information?” Now I can treat it appropriately with the policies that need to be applied to it.
(12:00) Are there other use-cases you feel are worth pointing out?
AC: Absolutely. There are some really simple ones. When you start talking about contracts, you think legal, but it’s actually more than just legal within a company that’s interested. You have the really simple question of perhaps a salesperson who’s just been assigned a new account being able to say, “Okay, show me all of the contracts for this account.” That’s one.
Then more in the legal realm, let’s say you went through an acquisition, and you now have a whole pile of contacts from that acquisition where, frankly, you really have no idea what kinds of clauses are in there and how they might compare to your clauses. That’s another use case: being able to actually compare something to your standard and being able to find areas where clauses are substantially different from your standard, being able to zero in on that. In other words, give the lawyer access to the ones that actually might be problematic or most important to be looked at.
Then another one is limit of liability. That’s a really popular one. The GC walks by a junior lawyer and says, “Hey, for this recent acquisition, what’s our exposure from a limit of liability for data breach across these customer contracts?” How do you answer that today? Well, you lock a whole bunch of these lawyers into a room for could be days, could be weeks reading contracts. You can extract that information and make that available for really a few clicks in the visual search UI to find those contracts that have liability above a standard term or that have liability that would concern us from a specific clause, like the data breach limit of liability.
It does go across. It’s really a question of thinking through what the questions are you’d love to answer and then enriching the information using machine learning and AI in order to be able to answer those questions.
The reality is, sometimes we negotiate different terms. Sometimes we use the customer’s paper because that’s what ends up happening. Sometimes you have stuff that comes from margins and acquisitions that looks completely different.
Really, the power of machine learning and AI is that you can do this even when you’re dealing with very disparate-looking content, so being able to find that right clause that I’m trying to look at. Yes, it does require training, but being able to do that in a platform where we approach it as a pre-trained model that gets refined for a given customer, as opposed to a customer doing this on their own.
(16:00) Iron Mountain is doing work in financial services, banking, insurance, and energy. What are some of the interesting ways where search and discovery AI fits in those vertical examples?
AC: Actually, energy is a great one. Again, I think it illustrates various parts of the things that we’ve talked about. One use case in energy is, you’ve got your geoscientists who are looking for all the different assets that the company has in order to drive decisions around, “where should I drill?” Or, “what’s the value of a given well?”
The kinds of stuff that you’re dealing with might be huge maps that are paper, so digitizing those and being able to leverage those. They have seismic data on tape. There’s vasts amounts of seismic data on tape, so being able to crack those open, ingest that content, and make that available to the geoscientists, as well as all of the digital-born information that’s available. So vasts array of information that one of these geophysicists needs to be able to leverage.
The way that they need to leverage it is based on, “show me everything about a certain spot on the map.” So the difficulty that they’re facing is where they’re spending half of their time searching versus actually analyzing the information is going across all the different repositories, and, frankly, not being sure that they found all of them.
Some of this data is very, very expensive to generate, so you really want to make sure that you’re using what you already have as opposed to potentially not finding it and having to generate it again. From an input perspective, various sources across physical and digital that are important here.
Then from a processing perspective, it’s all about adding the right kind of metadata, enriching the information in the right way. For this particular use case, you’re looking for geolocation. You’re looking to determine what area of the globe this particular map is for, this seismic is for, this well log is for. You’re looking to extract that information so that now the geoscientists can actually access it by location. They can essentially point to a spot on the map and say, “Give me all the assets for this spot on the map so that I can determine where we should drill, or I can determine the value of a well.”
Another piece that is important here is there is metadata available already. If it’s physical assets, our customers are keeping lots of metadata about assets that they store with us. It’s important to be able to marry that up with the digital or digitized contents so that you’re not losing any of the information that you do have about these assets as well as potentially external sources. So for oil and gas, maybe an IHF database, etc.
Being able to take what we generate from a machine learning AI perspective, in terms of net new enriched metadata, alongside the existing metadata, and being able to create those relationships between all of that metadata and across all of these assets is really where the power comes in of very, very quickly enabling those geoscientists to find the right stuff and spend most of their time analyzing and very little of it trying to pick together all the information that they need.
There is another really power thing for oil and gas, which is finding similar images…Perhaps there is a geological intrusion, and that’s a great sign for potentially finding oil. Well, you want to be able to find any other images that you have that look like that so that you can identify potentially good spots on the map where you might want to drill. That would be a great example for oil and gas, in this case, for subsurface records.
Clearly, faster analysis, better decisions is what you’re looking for in terms of an outcome. I think, obviously, a lot of those use cases still have to be flushed out because of how new that is to this oil and gas sector. Leveraging image assets in any way other than slowly yanking the meta of a digital file or a physical file and looking at them manually is going to be new.
But clearly, if we have that kind of visual data inside a whole bunch of physical locations where we’ve done our drilling, maybe we’ll have an idea of where our higher yield domains might be, and we can allocate our funds in a more efficient manner.
(21:00) What did you mean by metadata, and could that be from within or without?
AC: It can be from within; it can be from without. It could be metadata that you have in a repository already; it could be metadata that’s available out in the market for purchase, it could be publicly available information for certain properties. There is lots of public information. Really, the key is to be able to create the relationship between all of these different bits and pieces and making it all part of the metadata that’s attached to an asset.
So now I know that this map pertains to a certain spot on the globe, and I also know that the well logs are from that same spot, and I also know what IHS data is available for that spot. That’s exactly the power is really adding to the metadata and creating that relationship so that you can go across the different asset types and search in a way that’s conducive to quickly finding the right information for the decision or for the question that you need to answer.
It’s really thinking through what the questions are you’d love to be able to answer, and then backing into, “what kind of information do I need to pull out of the different documents as part of the processing that we do with the machine learning in order to have that?” Or, “where is that information today that I already have? How do I now marry that up with these assets?”
It’s really starting from the end and starting from, “what questions do I want to be able to answer? What problems do I want to be able to solve? What manual steps do I have in the process that are slowing down that process?” And working backwards to, “what do I need to do in order to be able to speed that up, to be able to provide that information and answer those questions?”
(23:30) What are some specific use cases within banking or insurance?
AC: Lots and lots of banks have microfiche, decades worth of microfiche that may be account statement images. They took all of these account statements, they put it on microfiche. For those people who don’t know, that’s like an index card size sort of fiche that has hundreds of images, like pinhead size images.
So why would you want to get at that stuff? Because, clearly, that’s not last year statements or last month statements.
It might be an estate coming back to the bank and saying, “Hey, we think you owe us X million dollars for this particular estate.” And having to be able to go back and produce, for example, a couple of years worth of statements. Think through that, so go find the right microfiche, in the right box, put it on your little microfiche reader thingy, find the right square on that microfiche, get that digitized, and then do it again. That was month one. Hopefully, you only have one-page statements because if it’s two pages, you’re doing it twice on that particular month. So doing that for 24 months takes a really long time.
By the way, you’re not adding any value through this. All you’re doing is responding to a request from a customer. Being able to extract all of that information, and in this case it’s actually quite illustrative, it’s very simple, really, use case. I want to be able to search for an account number across a bunch of different years worth of data and find all of the relevant statements for a date range. It’s a very simple problem, but it’s very hard to do with physical microfiche. Again, this is very prevalent in the financial services sector.
Another example is mortgages. As a customer, a lot of this stuff is digital now, and so we might think that there really isn’t too much paper involved anymore. Yet, sometimes when you end up sort of at the end of that process, you still get a stack of papers. Oh, by the way, the back-end processes often times still involve literally printing that stuff out and having physical paper sort of moving around.
So this process, first of all, takes a long time. It takes longer than it should, and part of the reason for that is that there is a lot of sort of manual intervention. Really, what are you trying to do? At the front-end of the process, you’re trying to figure out whether you have all of the information that’s required to make a decision about providing someone with a loan.
This is really illustrative of what do we do with classification and extraction? Classification answers the first question, which is, “do I have all the documents that are supposed to be part of this loan file?”
Once I’ve answered that with a yes or no, then I go to the next step, which is, “is this all filled out completely and accurately? So do I have a signature, or I expect a signature? Do I have the same name and the same social security number across all the documents in this file? Do I have the same APR or are there any documents that have a different name, or a misspelled name, or a misspelled social security number, what have you?”
Finding the information across the documents, that’s the extraction. I’m pulling out the name. I’m pulling out the social security number. I’m pulling out the APR. I’m pulling out all of these relevant bits and pieces to be able to answer the second question, which is, is it filled in completely and accurately? What you’re looking at here is a process going from north of a week to less than a day. Very powerful in terms of the customer experience, so presumably I’m going to get my loan faster, my approval faster, but also from an obviously process-efficiency perspective for the actual vendor, in this case.
Then the other thing is, now that I’ve done all of this work, I have really rich information that I can use in a secondary way. For example, am I compliant with fair lending laws? I now can actually answer that question as well. On top of having improved my process, I now have the ability to think about other secondary use cases that I can support so that I can answer questions like, “am I compliant with fair lending laws?”
Yeah. All of this seems to tie to the big point that you’d mentioned around energy, where we really do need to ask ourselves, what are the questions we need to ask? What are the processes we want to enable? Because when you talked about lending, in order to set up something of that kind, of course, we’d want to know where we store all of those certain kinds of files, or what certain kinds of terms we’re looking for. To build out the process that would get something that would, like you had said, maybe take a week down to a day, we would need to do the strategic thinking ahead of time and set up the system so that search could work there.
(28:30) What kind of strategic thinking does a company need to do to integrate an AI search application?
AC: You want to have the right folks around the table and multiple interested parties potentially around the table early on so that you can make sure that, as you’re processing all of this information, you’re actually adding the right kind of metadata so that you can answer everybody’s questions down the line.
Now, that being said, you can certainly start with what is the most pressing, or the most urgent, and then add over time. So it’s not an issue of “I have to think about all of it at once.” In our example, we may come to the realization, once we’ve done this for the mortgage process, that we actually do want to be able to answer that compliance question as well.
We can do that by essentially sort of refining what we’re doing from a processing perspective and reprocessing the assets that we’ve already ingested to add that additional metadata that we’re now looking for. So you can absolutely come at it sort of bit by bit and solve the most pressing problems first, and same for things like policy.
Maybe initially you’re automating a process. You’re not thinking about applying your retention policy, but you may want to. Now that I know what I have, I can actually figure out how long I should be keeping it and process it for destruction when I don’t need to keep it anymore so that we start really getting our arms around this big, huge pile of unstructured data. Also, from the perspective of “should I be keeping it anymore?”
If you think that you’re going to want to go broader, the part that you should be thinking about early on is, “what does that means in terms of the kind of tool that I need? If I need to go across physical and digital information, what does that mean? If I need to go across video, images, and Office documents, what does that mean?”
So not getting yourself sort of in a corner where you go, “Okay. Well, we have this one thing that we want to solve, so let’s have this very targeted sort of solution.” But then, you turn around, and you go, “Okay, I’d love to solve the next problem as well.”
And now you’re looking at, frankly, having painted yourself in a corner where it’s like, “Well, we can’t process videos on the same platform.”
Now we’re rolling out a second one, and a third one, and a fourth one. So it is important to think about where you might want to go with it, but you don’t necessarily have to sort of start with everything all at once. You should be thinking about it as a first, second, third, but really do make sure that you understand where you’re ultimately potentially going to want to go so that, as you’re deciding to put in solutions, you’re not narrowing your choices down the line.
Subscribe to our AI in Industry Podcast with your favorite podcast service:
This article was sponsored by Iron Mountain, and was written, edited and published in alignment with our transparent Emerj sponsored content guidelines. Learn more about reaching our AI-focused executive audience on our Emerj advertising page.
Header Image Credit: Twitter