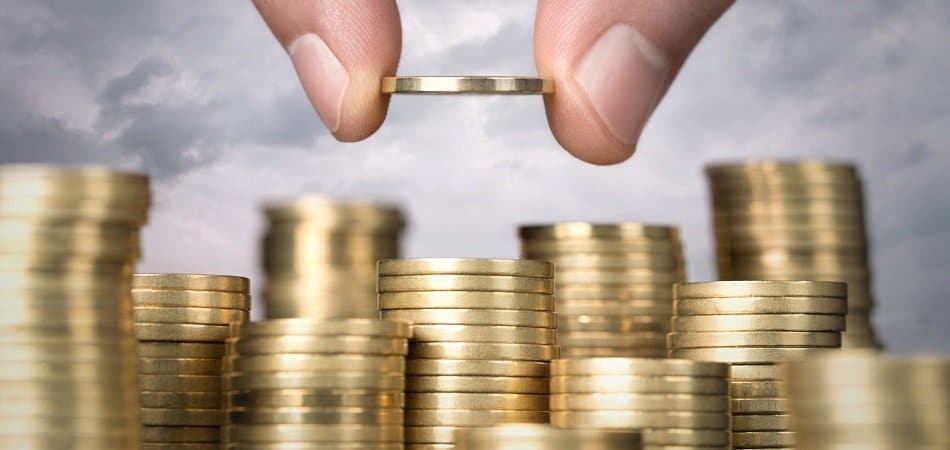
Many business processes in retail banking are ripe for automation with AI. All types of banks may appreciate the use-case of payment processing automation and fraud detection, but retail banks may also benefit from automated credit scoring and customer service chatbots.
We’ve explored AI in the banking space broadly, but in this article, we discuss what’s possible with AI in the retail banking industry specifically. We provide examples of a bank’s success with the software and detail how the software fits into the banking enterprise. Our discussion is organized into sections detailing the following capabilities:
- Fraud Detection for Retail Banking: How AI approaches such as anomaly detection may work to detect fraud and reduce false positives.
- Credit Scoring with Predictive Analytics: How AI can be used to determine a credit score and the data types that go into that.
- Chatbots for Customer Service in Multiple Languages: How natural language processing (NLP) software works to improve the customer experience and save on customer service costs.
We begin our overview of AI software for retail banking with AI-based fraud detection.
Fraud Detection in Retail Banking
There are multiple companies selling AI-based fraud detection solutions to retail banks for their consumer banking services, such as debit cards and mortgage applications. They most commonly use anomaly detection software to make it possible.
Anomaly detection applications for the banking industry are more common than those of predictive analytics. When banks invest in software integration, they need to install the anomaly detection software into their existing fraud management system. Then, the software is trained in real time on a stream of labeled data from transactions or loan applications.
The machine learning algorithm analyzes each new piece of data in order to gradually develop a baseline of normalcy before it can begin labeling deviations to that baseline as fraud.
When any banking event, such as a credit, withdrawal, or charge, deviates from the patterns the software recognizes as normal, it will notify a human employee. That person will have the option to accept or reject this notification.
This would give the software feedback on its conclusion about whether the transaction is fraudulent. The software uses rejected notifications as signals to allow certain deviations from the norm that originally registered as anomalies.
The bank’s payments expert can also improve the algorithm by searching for other instances of the newly recognized fraud method in past transactions and use them as further evidence on how that method can present itself.
One way this can happen is through patterns of customer behavior that can serve to signal an algorithm that someone else other than the assumed user is trying to complete an action. We spoke to Owen Hall, CEO of Heliocor, about the strengths and weaknesses of AI for fraud and risk mitigation on our podcast, AI in Banking. When we mentioned the more traditional rules-based systems, Hall made an observation that can also be true for out of box AI applications:
As people go through their daily lives, they leave more and more ‘breadcrumbs’ about themselves and those become open for people to use in the areas of defrauding people of their money. You’ve…got a whole area in terms of the banks looking at ‘how do I really know that the person who sat online doing a transaction is the person I really think they are.’ I think AI will [factor] into there.
Our readers can find more information about how anomaly detection applications have worked for important banks by, downloading the Executive Brief for our AI in Banking Vendor Scorecard and Capability Map report.
How AI detects Anomalies
Anomaly detection applications could be used to recognize and prevent fraud through numerous data points associated with each transaction. If a customer’s credit card information was stolen and the thief made purchases hundreds of miles away, the software may be able to recognize that the customer is not where the transaction came from and flag that transaction. Similarly, anomalous spending behavior such as a spike in repetitive payments could be flagged as well.
Anomaly detection software could recognize fraud within loan applications and other financial documents in a similar way. It may search for anomalies within financial information a potential customer provides based on a corpus of data from previous customers. This likely includes any financial information the bank already has on the customer so that it can recognize falsifications more quickly.
It is important for business leaders in banking to consider the effect this type of AI application will have on the workflows of their departments. Employees reviewing loan documents and banking history for fraud may need to be trained on the software’s user interface so they can accept or reject fraud notifications, for example. Added friction can cause lower employee productivity even while the fraud detection software is working fine.
We interviewed Muriel Serrurier Schepper, Business Consultant of Advanced Data Analytics & Artificial Intelligence at Rabobank in Holland, about how to integrate AI into long-standing businesses efficiently. When we asked her about the challenges she has experienced in bringing AI into an established company, Schepper said:
A lot of AI companies come in…and tell us there’s no humans needed any more. Then we start a project and oops, we still do need to do a lot of work ourselves…That continues throughout all levels within the company.
Schepper stresses the importance of the fact that companies should not approach AI solutions with the hope to fully automate their desired business process right from the start. In the case of fraud detection, employees will still be needed to have the final say on whether or not a transaction is fraud (at least until the algorithm is trained sufficiently), as well as accept or reject detected instances of fraud during training.
One AI company offering fraud detection to retail banks is Threatmetrix, a subsidiary of LexisNexis. Their Dynamic Decision Platform purportedly improves account authentication and identity verification.
The company claims to use anomaly detection to find a baseline for acceptable customer behavior and detect anomalous transactions that deviate from that behavior. These transactions are then scored on their likelihood of being fraudulent, which likely correlates with how far the transaction deviates from the norm.
Threatmetrix claims the Dynamic Decision Platform pulls data from their Digital Identity Network, a platform they use to store transactional data.
Below is an image from ThreatMetrix’s website detailing the four main components of their Digital Identity Network. These include giving individual customers a unique ID code and employees a visualization of all the credentials attached to a given customer. The system then scores customers on “confidence, or the likelihood of a transaction to be fraud, and “trust,” the relative reputation of that customer ID code.
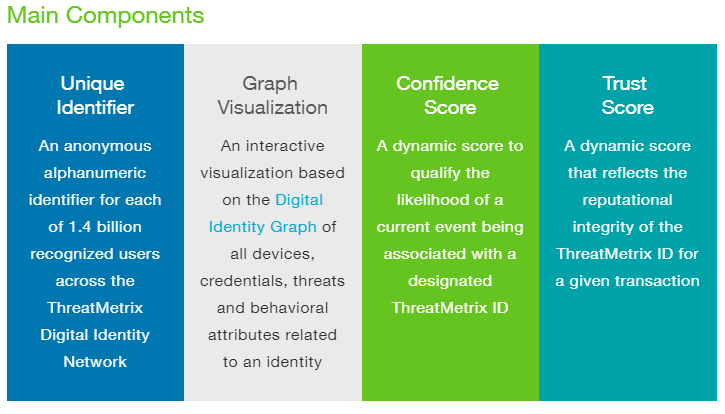
According to a case study from Threatmetrix’s website, the company helped Beyond Bank reduce fraud from stolen financial information by replacing their existing fraud detection solution. The case study states fraudsters were targeting Beyond Bank’s customers that were less accustomed to the digital world and abusing their ignorance online to get their credentials.
After integration, Beyond Bank saw the software recognize 98% of returning customers after being trained on their customer information. The software also rated over 70% of them as trusted, meaning it was able to recognize the bank’s legitimate customers based on their spending behavior. At the same time, customers who did not come back as “trusted” were likely reviewed for past instances of fraud. This resulted in reduced false positive rates as well. Through detecting anomalous transactions, the bank was purportedly able to prevent and stop takeover attacks on their customers’ accounts.
Credit Scoring with Predictive Analytics
In addition to fraud detection, retail banks could also use AI applications to automate credit scoring. Predictive analytics applications in particular may help retail banks estimate the risk of a potential customer with more accuracy. These applications can also use data outside of traditional credit and financial history to determine someone’s credit score.
When determining a customer’s credit score, predictive analytics software would run data the bank has about the customer through its algorithm. The software then calculates how much risk the bank would take if they chose to underwrite them.
This type of application may help retail banks expand the number of people they can give credit due to the wider range of data types they can use for credit scoring. This would include social media posts along with interactivity such as liking, sharing, or commenting. The customer’s online behavior may be an indicator that they will make payments on time and pay their loans back in full.
Extracting data from social media posts would require natural language processing software. This could recognize the content within those posts and determine whether their sentiment is positive or negative. The sentiment of the post becomes a data point which the predictive analytics software can then factor into its credit scoring calculations.
Retail banks using this type of application may be able to accurately score customers who they would not be able to score highly otherwise. Banks may be able to increase loan repayment rates in underbanked populations by leveraging their social media activity and eCommerce internet history.
As AI technology improves and automated credit scoring becomes more widespread, business leaders in banking may want to consider the changing requirements of their staff. For example, if a bank adopted a credit scoring application which could automatically score customers once they submit a loan application, the underwriter may only need to review the software’s report and approve it.
We spoke to Joy Dasgupta, CEO of Empirican about the topic on our podcast, AI in Industry. When asked about the most important employee roles for businesses using AI, Dasgupta said:
What happens to people in processes that we automate using our AI solution and our knowledge-base automation? Our task…is to really automate manual processes, and of course there will be displacement here. and the roles that remain are that of the exception management team…in the new model it’s checkers, and a high-end checker who has a broader view and broader set of responsibility that’s managing their processes.
“Checkers” in this case refer to those who monitor the AI application and approve or reject the incoming notifications and results. Underwriters may need to develop an understanding of AI systems to become them when AI becomes more ubiquitous for credit scoring and other underwriting use-cases.
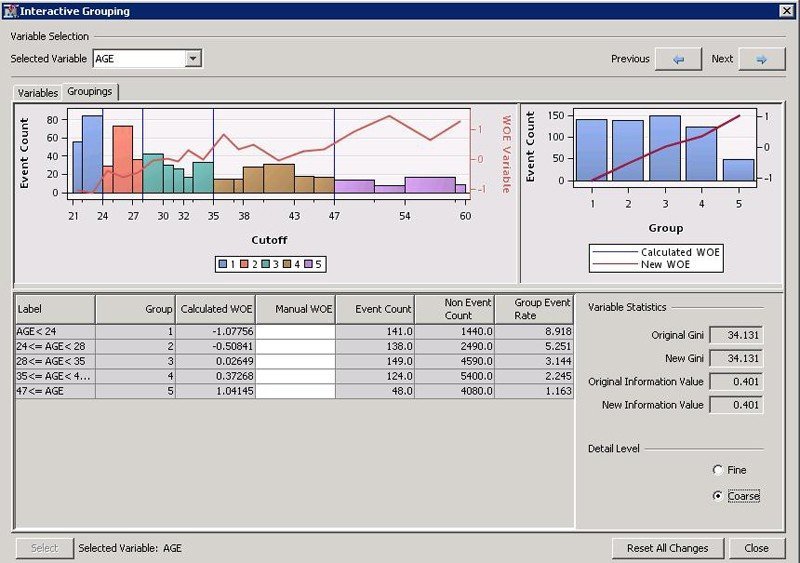
SAS offers a predictive analytics software called Credit Scoring for SAS Enterprise Miner. The solution is an added capability of their larger Enterprise Miner solution. The software’s machine learning algorithm sifts through a client bank’s enterprise data for all relevant information regarding the customer’s financial history. The software then uses predictive analytics to determine a customer’s credit score based on that data.
The image on the right is from SAS’ website, showing the “interactive grouping” capability of their credit scoring software. This shows how the user can change the number of variables being tested for their impact on customer credit scores and compare the results to one another.
The company published a case study in which they claim to have helped Piraeus Bank Group accurately credit score new potential customers.
According to the case study, SAS’ software helped Piraeus speed up their credit data analysis and report generation times by 30%. The bank also purportedly used the software to find the best possible way to project their predictions into the future. This includes the capability to test multiple variables and easily switch between them. Each variable is weighed differently depending on the other variables present in the customer’s data.
Chatbots for Customer Service in Multiple Languages
Some retail banks add a customer service chatbot to their mobile banking app so their customers can find help easily and navigate through the app with less friction. Customers can type or speak questions into the chatbot regarding most banking services. These include checking their balance across multiple accounts, the requirements for mortgage loan approval, and how to cancel a credit or debit card.
Chatbots run on NLP software and determine the intent behind a customer’s question in real time. This would require training the machine learning model on banking terms and which parts of the bank’s mobile app they correlate to such as where to check one’s balance or switch the account they are checking. Additionally, chatbots can continue to improve their answers and expand the types of questions they can handle by flagging new questions to be reviewed by a human employee. The employee can then use that question a training opportunity to teach the chatbot how to respond to that question in the future.
Banking leaders may see some areas where customers are experiencing the most friction and want to create a chatbot that handles those questions. Companies may want to focus on specific customer issues rather than fielding every type of retail banking question. Conversely, a bank with a less robust customer service department in general may want a chatbot that can handle the simplest, most often asked questions by customers to lower the traffic of their call center.
In this example, each of these possibilities may be the right decision for an individual bank. However, neither will be the correct answer for every bank. We interviewed Sid Reddy, Chief Scientist at Conversica, about this, as well as the capabilities and limitations of chatbots in general. When asked about whether it is more important to prepare a chatbot for multiple purposes or to train it to handle one specific task well, Reddy said:
Actually it’s neither. The fundamental reason we have different agents doing different things is because natural language processing and machine learning is unsolved, and it is very difficult to understand natural language the way humans write it. We don’t have a model that understands everything for every context.
So what researchers and companies do is they collect annotation data for a given task or for a given knowledge summarization objective, and they’re trying to model for that particular annotation task, for a given intent, and corresponding set of entities. That particular model can only do well based on the examples it has. So for that reason different companies focus on different intents and entities.
Reddy’s quote makes it clear that training a chatbot is not as simple as deciding what types of questions the bank wants it to be able to answer. Instead, the bank must be constantly aware of what questions their customers are asking, and use those new questions to help the machine learning model adapt to new topics and ways to ask about them.
For example, a bank may have many customers who are confused about how to view their savings account balance when their checking account is the default on the app. If the bank did not prepare for this type of question originally, the chatbot may not be able to recognize what is being asked.
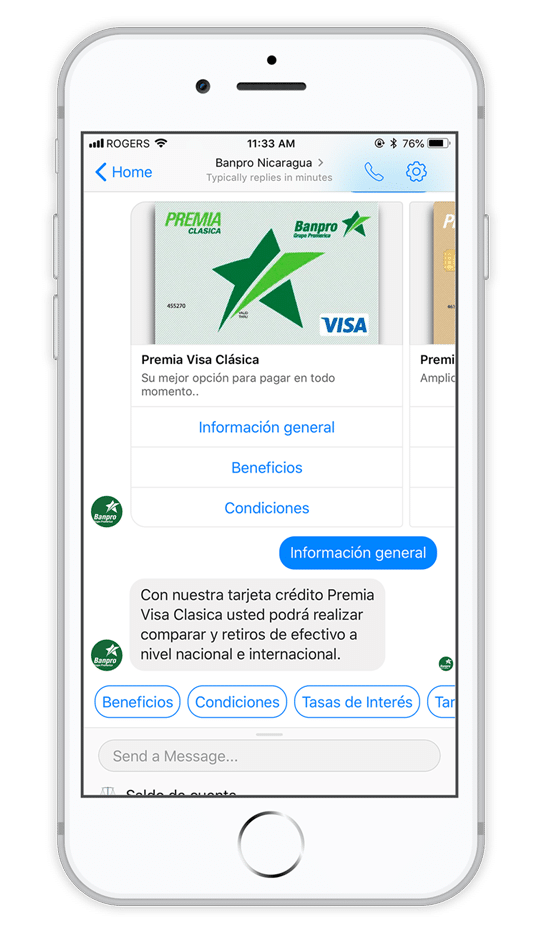
In cases like this, the customer may be referred to a live customer support agent, and the question would be logged so that it can be used to further train the machine learning model behind the chatbot.
Chatbots can also be developed to field questions in more than one language. Finn AI offers NLP software that they claim can help banks develop and train accurate chatbots. They claim their software also features sentiment analysis, which allows the chatbot to determine whether the sentiment behind a message is positive or negative.
This could help banks understand when the chatbot gives helpful answers or when it is frustrating the customer.
Banpro Case Study
FinnAI claims to have helped Banpro, a Nicaraguan retail bank, offer a chatbot that can field questions in both English and Spanish.
This type of chatbot would require a machine learning model that has been trained to recognize topics and phrases from multiple “sets” of words at a time. These sets are categorized as languages, and the software can correlate phrases from either language to data points that may be most helpful.
Additionally, the machine learning model would need to be able to produce answers in both English and Spanish. This would require the bank to decide on a series of scripted answers in both languages as well.
According to the case study, Banpro saw 2.2 million active users of their social media chatbot. This equates to 36% higher customer engagement since 2016. Additionally, their mobile website traffic rose by 32% since 2016.
Header Image Credit: cio.co.ke