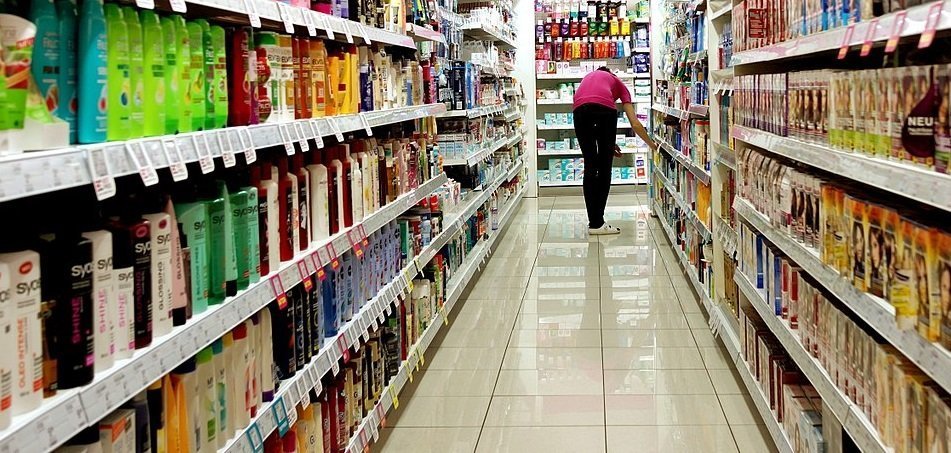
There are several companies claiming to offer AI solutions to consumer packaged goods (CPG) companies. We found that these solutions are intended to help CPG companies with at least one of the following business problems:
- Trade promotion optimization (TPO)
- Understanding customer opinion and preferences
What Business Leaders in Consumer Packaged Goods Should Know
AI solutions for business problems in the CPG industry appear to be less legitimate than we first thought. All of the companies discussed in this report employ relatively credentialed people in their C-suites, but their AI experience is generally lacking compared to other sectors we’ve covered (in terms of AI-related talent density, and experience actually using AI).
As we explained in our detailed article about cutting through AI hype, we use the following three signals as major indicators that a company is offering a product that genuinely leverages artificial intelligence:
- Strong AI leadership from experts who have either:
- Robust academic experience in AI or related fields (MA or PhD in physics, computational biology, computer science, or statistics)
- Robust experience as part of an AI team at a marquee AI innovation company like Google, Amazon, Baidu, or IBM
- Tens of millions in funds raised from experienced venture capital firms. We prefer to look for the most tech-savvy firms such as NEA, Sequoia, and Andreeson Horrowitz.
- A clearly stated value proposition, explaining (a) the main problems the company solves, and (b) how artificial intelligence is used within the company’s offerings
The companies we examine in this report are older firms, who, unlike some of their startup competition, have no founding team members or C-level leadership with a strong background in AI. Many of the firms featured in this article, however, have hired experts in AI to run their AI practices and build AI-related products and services, but others have not hired any such experts to back up their claims of AI use.
Fractal Analytics employs a Head of Artificial Intelligence with a PhD in Computational Neuroscience and Machine Learning that he earned from Caltech in 2007. This kind of credential is the strongest trust signal we use to determine whether or not self-purported AI companies are lying about doing AI, and for this reason, we trust Fractal Analytics with providing a real machine learning solution.
Whereas Fractal Analytics has a value proposition that is predicated on AI, Genpact and Wipro are only now starting to adopt AI into their suite of business solutions and consultancy offerings. Founded in 1997 and 1945 respectively, these companies are enterprises with close to 300,000 employees between them. It’s likely that their foray into machine learning is a way to maintain relevancy as opposed to how Fractal Analytics have involved AI in their value proposition since its inception.
However, Genpact and Wipro are similar to IBM in that they have long been servicing IT firms and providing IT solutions. This differs slightly from the way large enterprises in industries like auto insurance are hiring data scientists and AI experts for the sole purpose of using the AI buzzword on their websites. Oftentimes, these enterprises may not release an AI product for years after they hire their data scientists; machine learning simply isn’t a priority for them the way it is for AI startups founded this decade or digitally-native data dominators like Google, Amazon, and Facebook.
Despite their age, Genpact and Wipro seem to be among the only legitimate providers of AI solutions for trade promotions. Genpact’s solution for matching contracts to invoices is more administrative than Wipro’s solution, which intends to determine the best promotional sales for a company’s customers based on historical data that feeds a predictive analytics model.
Fractal Analytics makes use of relatively new social media data, mining social media posts for mention of a company’s products and analyzing the sentiment behind those posts using natural language processing; Fractal Analytics claims this can inform the kinds of products a company decides to develop.
Understanding What Customers Think About a Brand’s Products
Fractal Analytics
Fractal Analytics offers a software called dCrypt, which it claims can help CPG businesses mine social media posts to see what people are saying about their brand using natural language processing for sentiment analysis. This analysis could then inform successful marketing strategies.
We can infer the machine learning model behind the software was trained on thousands of snippets of conversation from emails and messenger apps, as well as social media posts like a facebook status or a tweet. These snippets could be related to how CPG products worked for them, if they liked the price compared to competitors, or if they will buy the same product again. This text data would have been labeled as a positive or negative experience with the product, as well as by what the customer specifically thought was wrong.
For example, a customer could tweet about how the client company’s garbage bags are not perforated. A human would then label that tweet as a negative sentiment. The labeled text data would then be run through the software’s machine learning algorithm.
This would have trained the algorithm to discern the chains of text that, to the human brain, might be interpreted as a positive or negative comment about a product as displayed in the form of a tweet, instant message, email, or other social media post.
The user could then upload text-based conversations that are not labeled into dCrypt. The algorithm behind the software would then be able to find keywords, topics, and phrases within the text and determine the sentiment behind them. The system then provides those topics as categories for the text-based conversations, likely subcategorized as positive or negative based on the sentiment.
These categories are organized to find outlying conversations about a product, which could lead to important insights. For example, if a customer tweeted about finding an alternative use for a product, the CPG company could evaluate if that alternative use is worth capitalizing on.
Fractal does not make available any videos demonstrating how dCrypt works.
Fractale claims to have helped a leading CPG company gain a better understanding of mouth pain in the public, with the intention of addressing that issue with their products. The client company integrated Fractal’s software into its website and social media data about customers and their dental products. According to the case study, the client company was able to detect ten new topics concerning mouth pain, which could have an effect on the market share for its dental products.
Fractal also lists Kimberly Clark as one of their past clients.
Prashant Joshi is Fellow and Head of Artificial Intelligence and Machine Learning at Fractal. He holds a PhD in Computational Neuroscience and Machine Learning from the Graz University of Technology. Previously, Joshi served as a research fellow at the Frankfurt Institute for Advanced Studies. We interviewed Joshi on an episode of our AI in Industry Podcast: How Machine Learning Could Help CPG Companies Beat Out Their Competitors.
Trade Promotion Optimization (TPO)
Genpact
Genpact offers a software called Contract Assistant, which is a sub-product of Genpact Cora. Genpact claims Contract Assistant can help CPG companies stop overpaying retailers for carrying out promotions such as sales or in-store displays. The software uses natural language processing to match trade promotion contracts to invoices.
We can infer the machine learning model behind the software was trained on thousands of digital trade promotion documents involving retailers, contracts, and payments. This text data would have been labeled as contracts, trade pay, proof of performance, or payment, among others.
The labeled text data would then be run through the software’s machine learning algorithm. This would have trained the algorithm to discern the chains of text that, to the human brain, might be interpreted as contracts, trade pay, proof of performance, and payment as displayed in digital trade promotions contracts, invoices, and compliance templates.
The user could then upload trade promotions and contract data that is not labeled into the Contract Assistant. The algorithm behind the software would then be able to evaluate the necessary type of contract, price, and quantity of the product, and payment amount for the promotion. The system then provides a finalized contract document between the company and the retailer and uploads the contract, invoice, and compliance template for validation. After all three of these are validated, the system processes the payment.
We could not find a demonstration video available for the Cora Contract Assistant.
Genpact claims to have helped a major North American food company automate promotions processes that are susceptible to human error. The company manually processed over 300,000 transactions across 150 retailers per year, but they found it impractical to audit every single transaction, which resulted in overpayments. The client company used Genpact’s software in combination with its own trade pay data, which accounts for contract data, customer invoices, market data, and payments to retailers.
According to the case study, Genpact used the Contract Assistant software to make integration simple for the client’s employees to use. This included an assessment of which digital tools the company would be ready to integrate and an implementation plan for those tools. Genpact also provided the client company a 10-week example exercise that automated the process for two retailers.
The client company moved forward with Contract Assistant as their automation solution. The Client is projected to save $21 Million per year from reduced promotion overpayments. However, the name of the client food company was not disclosed.
Harit Nanavati is SVP of Product and Technology and CTO at Genpact. He holds a MS in Computer Science from the University of Arkansas at Little Rock. Previously, Nanavati served as co-founder and CTO at Akritiv.
Wipro
Wipro offers Promax Optimize, which it claims can help CPG companies optimize future trade promotions using predictive analytics.
We can infer the machine learning model behind the software was trained on hundreds of thousands of past revenue data points from promotional sales involving complementary products, those which are often purchased together, and millions of customer demographic data points. The data would then be run through the software’s machine learning algorithm. This would have trained the algorithm to discern which data points correlate to the highest ROI for a promotional sale.
The software would be able to predict when to launch a particular sales promotion to generate the highest ROI, which customer segments to target for the promotion, and which kins of promotions might result in cannibalization. Cannibalization occurs when a company’s product dips in sales because customers are purchasing another of the company’s products. Users could then access the prediction and information about cannibalization risk on the software’s digital dashboard.
The user company may or may not need to upload information about its upcoming promotional sale for a particular kind of product into the software beforehand.
There are no demonstration videos available showing how Promax Optimize works.
Wipro claims to have helped one of the largest global manufacturing and marketing companies in the Consumer Packaged Goods industry. However, the name of the manufacturer was not disclosed. The manufacturer integrated Wipro’s software into its trade promotion management system. According to the case study, the manufacturer saw a $3 Million incremental net revenue increase in six months, an 18% reduction in promotions that resulted in a loss, and an undisclosed amount of increased ROI from promotions.
Abidali (Abid) Z. Neemuchwala is CEO at Wipro. He holds a Master’s Degree in Industrial Management from LIT Mumbai. Previously, Neemuchwala served as Vice President at Tata Consultancy Services.
Header Image Credit: Wikipedia