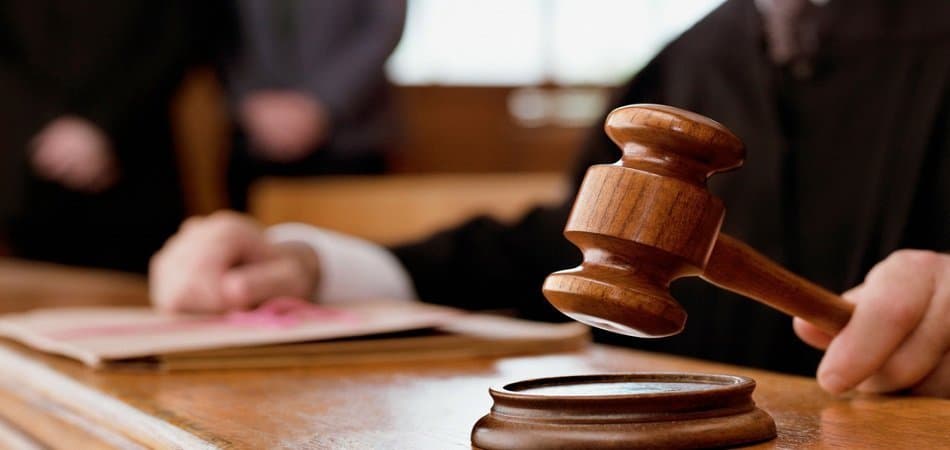
A discussion on AI ethics and the way AI might influence policy necessarily involves three stakeholders: world governments and policymakers, industry leaders and regulatory compliance bodies, and business executives making strategic decisions.
Businesses have arguably started to establish a valid ROI for AI applications particularly in healthcare, finance, and defense. That said, there are questions regarding the ethical challenges that AI is likely to pose in the coming decade. Business leaders and government officials alike may grapple with these questions, and business leaders in particular may respond to them in opposite ways.
The Future Society and Global Ai Initiative recently released the results of their survey, A Global Civic Debate for AI. The survey was open to the public and garnered more than 2,000 participants between September 2017 and March 2018, providing them a forum to discuss various topics on the ethical considerations of AI. The resulting report in part deals with Smart Governance. Smart Governance involves understanding the implications of AI and creating policies to regulate the technology.
We outline some of the ethical concerns that established AI companies and those looking to implement AI may want to consider in order to best serve their business goals and maintain good public relations. At the very least, we speculate on how large and small firms will respond to these concerns and how regulatory bodies may start paying attention to Smart Governance policies related to:
- Preserving Competition in the Market
- Algorithmic Bias
- Algorithmic Transparency
In this article, our aim is to elaborate on each of these considerations and paint a possibility space for what business leaders at both large and small companies should expect from both public opinion and regulatory bodies. Respondents of the Future Society’s survey also provide their thoughts on what to do about these ethical concerns, and we discuss them in further detail below.
Understanding the ethical considerations for what AI may or may not be capable of in the future will likely play a larger role in the future when it comes to successfully implementing this technology.
Preserving Competition in the Market
The emergence of AI is encouraging for venture capitalists; AI might well be a winner-take-all investment opportunity, since the company that has access to the most data and the best AI product is likely going to have access to more data in the future. More data will in turn make its AI product better, and a better product means more users—more users, more data.
“Data is the new oil.” This is a common analogy passed around in data science circles, and it references the potentially disruptive power that comes with leveraging big data in business. That said, not every company has the same level of access to data nor the resources to acquire it. In such a scenario, access to and ownership of data coupled with good AI products might lead to a market where large firms are likely to become “data dominators.” This might stifle competition.
For example, it is unlikely that a general online marketplace will be able to compete at a level anywhere near that of Amazon because Amazon has access to massive amounts of data that other companies in its sector do not. This data fuels its recommendation engines, and as a result, Amazon continues to have the best product when compared to its potential competition.
A Global Civic Debate for AI survey respondents were concerned that data ownership and, in turn, AI technologies, would amass in the hands of a small number of large firms and wealthy individuals with the resources to acquire them. Respondents suggested open source code, more transparent machine learning algorithms, and economic policies aimed at lessening wealth inequality as possible ways to hedge against this future.
How Companies Will Respond to the Ethical Considerations of AI
Large companies such as Facebook, Google, Amazon and even Baidu in China are already the clear data dominators. As such, business leaders can expect these companies to downplay their data dominance. It is in the self-interest of these large companies to avoid attracting attention to the ethically-debatable advantage they have when it comes to AI. Since being the sole data dominator is only going to cement a company’s position as a market leader, it is unlikely that they will be the ones starting the conversations on the fair use of data and data privacy.
On the other end of the spectrum, the vast majority of smaller companies, startups, and non-tech dominated firms don’t have enough data to start any meaningful AI projects. If they do, they’re only just beginning to explore the possibility space of AI in their industry. Large firms like Facebook and Amazon are predicated on AI and are therefore greatly ahead of every other firm when it comes to data access. It might be in the self-interest of these smaller firms to oppose the data dominance of larger firms, bringing into question the fairness of the market into which they sell.
Business leaders may expect this in this coming years, and these smaller firms are perhaps doing what is best for them by speaking out against the data dominators. Joining the outcry of a populace concerned about the data dominators may put pressure on regulatory bodies to lessen the dominance of large, digitally-native companies. That said, if these smaller firms had access to large volumes of data, they may not cause an uproar at all. In other words, it’s possible that any moral outrage on the part of businesses only serves to bolster their business goals.
How Companies Will Respond to AI Regulation
The Future Society report states that businesses have a history of “putting performance over safety.” For instance, finance and healthcare companies might collect sensitive information from their customers, such as their loan and medical histories. In the event of a data breach, such as what happened at Equifax and Facebook, fraudsters could use the companies’ data to steal its customers’ identities.
Security measures may cost a great deal of money and human resources to implement, and companies may not consider them before public opinion on their brand turns against them as a result of a data breach. Companies are just now learning that they need to prepare for and respond to the ethical considerations of an increasingly digitized world, and AI, which runs on big data, is the logical next consideration.
Business leaders can look to existing regulations as a cue on what to expect from government bodies. The U.S Government has antitrust laws, or competition laws, that are statutes to protect consumers from predatory business practices by ensuring that fair competition exists in an open-market economy.
It might follow that these regulations are extended to data and data management. These data policies of the future might depend on how well the larger companies manage to lobby for specific legislation that might be favorable to them. At the same time, they may also depend on how well the startups and small businesses manage to influence policymakers or the populace into implementing some of the suggestions outlined by the survey respondents, such as open- source code policies.
C-level executives at large firms with volumes of data may want to plan for what-if scenarios and develop methods to stay on top of their markets in either case. In case the government decides to extend antitrust laws to data, large companies might need to identify the type and amount of data they are willing to part with in accordance with regulations and which they might want to fight for in court. It might be less important for smaller firms to do the same since they likely store less data.
Algorithmic Bias
Machine learning software, for the most part, can be classified as “Garbage-in, Garbage-out” systems. They are only as good as the data they consume.
The datasets on which machine learning is trained are often collected and labeled by humans. These humans have their own biases and prejudices when they go to collect and label data. This makes it extremely difficult to feed unbiased data into a machine learning algorithm. The resulting model will likely carry the same biases as the humans who collected and labeled the data on which it was trained, even if those humans are unaware of their biases.
AI applications developed with unrepresentative or biased data might likely introduce algorithmic bias to the system, which in turn could unintentionally amplify these inherent biases. For example, the Future Society report points out that some judges use AI to predict a defendant’s recidivism rate. This score informs the way the judge sentences the defendant. In theory, factoring in an objective, statistical method for estimating recidivism over a judge’s intuition may seem desirable and fair.
In reality, the AI is in fact not objective. During the trial run for the software, evidence showed that African American defendants were more likely to be mislabeled as at high risk for recidivism than white defendants, who were more likely to be mislabeled as low risk. This labeled data then fed the algorithm that scored defendants on their recidivism. As a result, the algorithm was trained on a dataset that was racially biased. The humans labeling the data, in essence, instilled their own racial prejudice into the machine learning model.
Contrary to what most people might think, incorporating complex human values in AI projects and expecting them to function according to certain social norms is far more difficult to automate given the current state of AI.
According to Deutsche Welle, algorithmic bias is something researchers have been working on for years to eliminate, but without much success. Business leaders may have to make peace with the fact that the data available to them today may always have some type of bias.
How Companies Can Respond to Concerns About Algorithmic Bias
That said, large companies that are looking to build machine learning models might do well to steer away from datasets involving controversial data points. The bias in criminal sentencing is a public sector issue with no real consequence in business terms such as a bottom line. Negative press on the subject may influence the way people vote, but government officials are less likely to lose their job than had a private company built and implemented the biased model at scale.
Large firms should be aware of the PR risk involved in building machine learning models. When doing so, they could avoid turning the public against them by omitting certain kinds of data from the datasets they use to train their model. For example, they may remove race and gender data from their database before feeding it into a machine learning algorithm. The algorithm might then make predictions based on height data instead, which at present won’t likely get the company in trouble with the populace.
Large companies might want to figure out ways in which they can use the controversial data and still maintain positive public relations. It might behoove smaller companies to play this as a point of sale. They could say that their algorithms don’t factor in controversial data points, for example. The best option for small business executives might be to hope that their business decisions are perceived as good by the majority of their customers. In reality, there is likely no decision that will everyone happy.
Algorithmic Transparency
In addition, building interpretability into machine learning systems is a highly challenging task and one that still doesn’t have a good enough solution for most applications. For example, in deep learning applications, such as image recognition, AI software can be trained to identify people or objects in a video.
But deep learning is inherently complex and in several cases, researchers can only guess as to why the software classified an entity in the video as a person or an object. The complexity lies in analyzing the values of individual computing nodes in the algorithm which lead up to the end result image categorization. In machine learning research, this is known as the “black box” of machine learning.
Algorithmic transparency might be another cause for ethical concern. For example, an AI software might make decisions on where to invest large volumes of investor money. The investment manager may need to explain to investors why the AI made those decisions. If they can’t, investors may seek to sue the firm at which they work. Similarly, a doctor that makes a diagnosis based on an AI software may need to explain to a patient why the software gave them the diagnosis. Patients may likely feel extremely uncomfortable if their fate is determined by a machine rather than a medical professional. Businesses looking to build AI software to make decisions like these may find it difficult to both remedy the PR difficulties that comes with the territory and maintain a product businesses, such as investment firms and hospitals, want to use.
How Companies May Approach AI Initiatives in the Future
AI applications can vary vastly from image recognition to extracting text from documents. Across such a broad spectrum, certain ethical figures of speech might be hard to define. It might seem that creating AI with human values that are free from bias and always used for “good” is the obvious solution to these ethical concerns. But terms like “human values,” “free from bias,” and “good” might only have value when attached to real-world AI applications.
Business leaders may understand that the first layer of thinking about any AI initiative should be in the business context. If an AI application does not offer any returns, a business probably shouldn’t implement it at all, let alone consider its ethical implications.
Usually, companies do not approach AI applications with an ethical tilt. It is more common that a business gets around to having a conversation about the ethical considerations of AI only after they have implemented three of four AI projects. For a small business, there might still be a while before AI ethics become a major issue for them. This may work to their advantage because they can model their PR strategy on the likely inevitable difficulties the large data dominators will face when it comes to PR in the coming years.
Suggestions for Building Ethical AI
The Future Society reports that that only a few survey participants suggested the creation of ISO standards for AI and human rights audits for those standards. However, on the other end of the spectrum, some participants also felt that the ISO itself might not be the ideal barrier for preventing the misuse of AI.
Some respondents also suggested creating punishable rules that are built into AI systems. The AI software might then be able to “learn” the boundaries in where it can operate and understand how to adhere to these rule. This could aid in maintaining an open market and preventing bias. These types of solutions might be valuable in the far future, since any such endeavor requires massive changes to current legal systems and the allocation of liability, according to the Future Society.
Header Image Credit: Western Journal