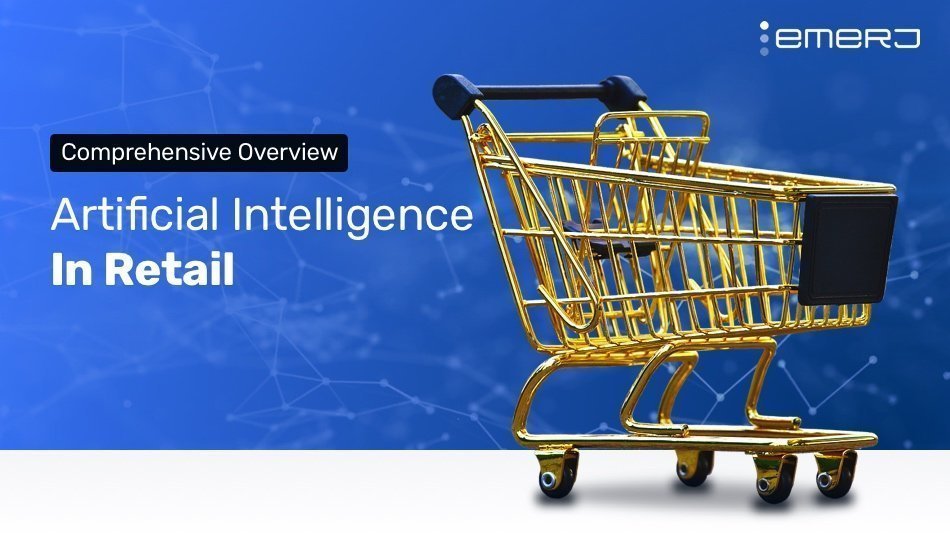
Artificial intelligence in retail is being applied in new ways across the entire product and service cycle—from assembly to post-sale customer service interactions, but retail players need answers to important questions:
Which AI applications are playing a role in automation or augmentation of the retail process? How are retail companies using these technologies to stay ahead of their competitors today, and what innovations are being pioneered as potential retail game-changers over the next decade?
In this article, we cover a variety of examples in which AI is being integrated in the retail industry, broken down into the following sub-categories:
- Sales and CRM Applications
- Customer Recommendations
- Manufacturing
- Logistics and Delivery
- Payments and Payment Services
Innovation is a double-edged sword, and as with any innovation results are a mixed bag. While many AI applications have yielded increased ROI—this case study of AI in retail marketing segmentation is one example—others have been tried and failed to meet expectations, shining a light on barriers that still need to be overcome before such innovations become industry drivers.
Below are 10 brief use cases across five retail domains or phases. Each provides a fraction of a glimpse as to how AI technologies are being used today and which are being created and piloted as potential retail industry standards in eCommerce and brick-and-mortar operations.
Readers may find additional insights in the retail space which were recently covered in our report on business intelligence.
Sales and CRM Applications
Emerj wrote an entire report on current CRM applications at Salesforce, Oracle, SAP using AI and more for further insights on this topic.
Pepper Robot
In 2010, Japan’s SoftBank telecom operations partnered with French robotic manufacturer Aldebaran to develop Pepper, a humanoid robot that can interact with customers and “perceive human emotions.” Pepper is already popular in Japan, where it’s used as a customer service greeter and representative in 140 SoftBank mobile stores. According to Softbanks Robotics America, a pilot of the Pepper in California’s b88ta stores in both Palo Alto and Santa Monica yielded a 70% increase in foot traffic in Palo Alto, and 50% of Neo-pen sales in Santa Monica were attributed to Pepper.
Additionally, the AI creation spent time at hip apparel store the Ave, where the retailer experienced a 98% increase in customer interactions, a 20% increase in foot traffic and a 300% increase in revenue. Nestle announced in January 2016 that it planned to acquired Pepper robots to put in 1,000 of its Nescafes in Japan.
While not the only retail robot in use, in-store robots like Pepper appear to at least initially boost store interest and sales. Whether this is a novel effect that will wear off once retail robots become “the norm” remains to be determined.
Conversica
Conversica “sales assistant” software is designed to automate and enhance sales operations processes by identifying and conversing with internet leads. The sales lead and management company claims the authentic-sounding messages result in an average engagement rate of 35%.
In one case study, Star2Star Communications implemented its Conversica-powered sales rep “Rachel” in 2016 and saw a 30% email response rate within hours. The customizable sales assistant software is also used to cross-sell or re-engage existing leads. New England-based Boch Automotive also employed Conversica software, which it attributed to a an average 60-sale increase per month at one Toyota dealership.
Customer Recommendations
IBM Watson Cognitive Computing
It’s no longer a secret that IBM’s Watson is providing a slew of order management and customer engagement capabilities to eCommerce retailers. In 2016, 1-800-Flowers.com launched Gifts When You Need (GWYN), which the company calls an AI gift concierge.
Through information provided by consumers about a gift recipient, the software tailors gift recommendations by comparing specifics provided to gifts purchased for similar recipients. The GWYN experience (which we included in our more complete article on chat bot use cases) attempts to replicate the role of a concierge at a store through a personal and detailed conversation with users. 1-800-Flowers Chris McCann spoke to Digiday, noting that within two months, 70% of online orders were completed through GWYN.
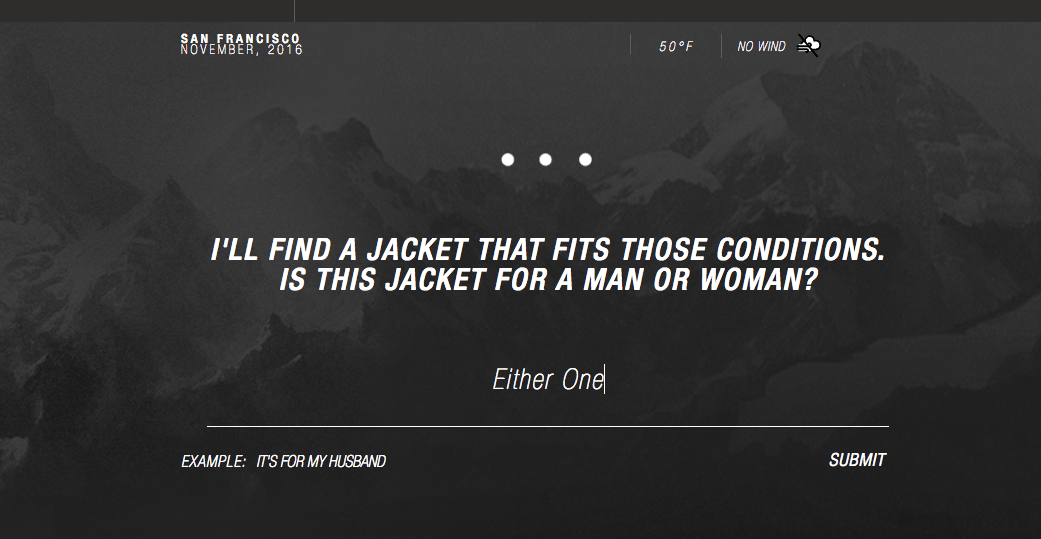
Above is an example of the North Face’s conversational interface, which prompts users with a series of questions related to their purchase. It’s safe to say that similar systems like the one above could be built with simple if-then rules, and no machine learning whatsoever.
The advantage of using machine learning in such a recommendation Q-and-A interface, is that North Face can potentially run tens of thousands of consumers through this conversational engine. At a certain volume of customer interactions, the system might be expected to glean important insights and patterns on suggestions that “work” (high take-rate to purchase, or high cart value), and those that don’t – allowing the company potentially gain higher and higher conversions over time.
Manufacturing
Interested readers may find additional insights on this topic in our machine learning in manufacturing report.
Brilliant Manufacturing
General Electric’s (GE) Brilliant Manufacturing software, in part inspired by GE’s relationships with client manufacturing companies over the past two decades, was designed to make the entire manufacturing process—from design to distribution and services—more efficient and hence save big costs over time. The software includes a suite of analytics and operational intelligence tools appropriate for a range of manufacturers.
The WIP Manager software, for example, provides industrial and discrete manufacturers with plant-floor and plant-wide collaborative visibility of all work in process. An operational supervisor sitting behind a computer can now identify in real-time a floor-based problem that arises in workflow, rather than spending time making time-consuming walkthroughs of entire manufacturing facilities.
Toray Plastics is one example of a company that is using GE’s Plant Applications product, which allows management to collect granular-level data throughout production and reduce defective products and wasted productivity.
Gakushu Learning Software
Fanuc’s Gakushu Learning Software (“Gakushu” means “learning” in Japanese) embedded in manufacturing robots speeds up “smart” operations on a specific task, originally designed for spot-welding and assembly lines. In 2016, Fanuc partnered with Nvidia, with the goal of accelerating deep learning in robots via Nvidia’s GPUs. Gakushu-endowed robots learn a manufacturing task through use of a sensor that collects and stores data.
The robots’ ability to adjust to real-time environmental conditions and adjust motion can (according to Fanuc) result in up to 15 percent cycle-time improvements in spot welding. Once the robot’s learning process is complete (about 18 cycles later), the sensor is removed and the trained robots are then able to complete a task autonomously.
The robots’ learning is paired with vibration control in the form of an accelerometer that learns the robot’s motion and provides increased movement stability. Tesla has employed about 600 Fanuc robots at its factory in Fremont, and according to Bloomberg Businessweek put in a significant order for more robots back in September 2016 in an effort to speed manufacturing efforts for the next slated delivery of its Model 3 in July 2017.
Logistics and Delivery
Domino’s Robotic Unit (DRU)
In addition to Domino’s claims that its prototype delivery robot can keep food and drinks at the appropriate temperature, the DRU’s sensors help it navigate a best travel path for delivery. DRU integrates robotics technology previously used for military combat training. In March 2016, DRU pilots were rolled out in Australia, New Zealand, Belgium, France, the Netherlands, Japan and Germany. Domino’s doesn’t provide dates as to when the DRU might be rolled out on a commercial scale, but it seems plausible that robotic delivery of food—and other goods—could be a burgeoning reality within the next decade.
Amazon Drones
In July 2016, Amazon announced its partnership with the UK government in making small parcel delivery via drones a reality. The company is working with aviation agencies around the world to figure out how to implement its technology within the regulations set forth by said agencies. Amazon’s “Prime Air” is described as a future delivery system for safely transporting and delivering up to 5-pound packages in less than 30 minutes.
The first 13-minute unmanned flight by Amazon took place on December 2016, as seen in the video below. At present, determining proper safety and reliability of operations and systems is Amazon’s top priority. Amazon notes that it’s working with regulators in “various countries”, though there haven’t been any updates on forecasted dates for commercial use. Similar to Domino’s DRU concept, it seems possible that autonomous delivery of goods and food by air could be rolled out at scale within the next decade.
Payment Services
Amazon Go
Amazon’s touted brick-and-mortar locations, known as Amazon Go, employ check-out-free technology that allow customers to shop and leave Customers use the Amazon Go app to check in, but thereafter the entire shopping experience is designed to be automated. Sensors track which objects customers pick up and put in their basket, and customers’ Amazon accounts are automatically charged after exiting the store.
The intended launch hasn’t been without its barriers, and at the end of March 2017 sources close to the retail giant announced that Amazon was delaying the opening of its convenience stores while it worked out “technology glitches” in the automated shopping and purchasing process.
PayPal
Since 2013, PayPal has leveraged fraud detection algorithms to protect customer’s digital transactions. Over the last few years, thousands of purchase patterns or “features” have been learned by the security detection system, which can now (in an example provided by MIT Tech Review) decipher between friends who are buying concert tickets simultaneously and a thief making the same kind of purchases with a list of stolen accounts.
A referenced study by LexisNexis found PayPal’s deep learning approach to transaction security reduced fraud rate to 0.32% of revenue, which is 1% less than the average rate seen my most eCommerce merchants.
Payment Fraud
Fraud and payment security are a massive area of AI investment, and there are plenty of fraud / security companies worth looking at. Sift Science is one of many companies applying machine learning to detecting user and payment fraud – both of which are relevant for retail applications. This will become more so as US eCommerce continues to swell as a percent of retail sales (and that growth is happening consistently, according to the US Census Bureau).
AI in Retail – Concluding Remarks
In many of our interviews with retail-focused AI vendor companies, we’re told that “big box” retailers (Best Buy, Target, Walmart, etc) are extremely slow to adopt cutting-edge technologies. Because it’s mostly large companies that have the budgets and data volume required to make the most of many of today’s best AI technologies, we outright surmise that an “AI revolution” in the retail space is unlikely. It may be another three to five years before most large retailers have substantial, business-critical AI applications in manufacturing, supply chain logistics, or customer service.
Applications that have the highest likelihood of broader retail adoption are those that have a direct, hard-line return on investment. “Improving customer engagement”—even with case studies and examples—is a softer benefit than “reducing lost packages by 6-10%.” Our retail executive guests using AI expect that the relatively stodgy committees in these large companies is likely to be extremely critical, safe, and bottom-line focused (for more insights from machine learning industry executives, visit our AI podcast interviews channel).
As with many areas of AI innovation that are led by bigger industry players, the future will likely be dictated by the retail AI use-cases that are proven effective by leading industry players. It’s safe to say that every at-scale retailer in the world is looking to Amazon for hints on “next steps,” and we can expect that the broad swath of relatively smaller retailers will be looking at Amazon, Walmart, Best Buy, and others for their own ideas on strategy.
We advise businesspeople interested in retail AI applications to look closely for successful traction in AI use cases (i.e. applications that actually drive profitability for the firm, not flashy R&D projects) from Amazon and other large players over the coming 18 months.
Initial research for this article was co-written and primarily researched by Karl Utermohlen.
Image credit: Shutterstock