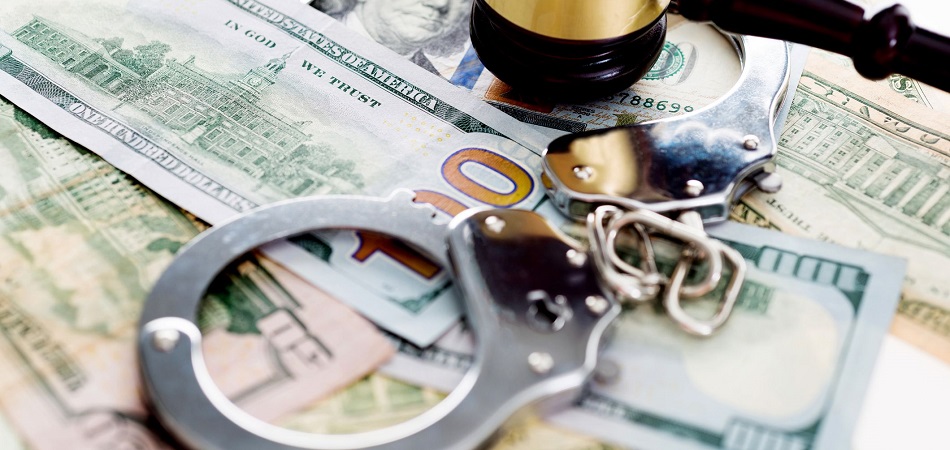
Money laundering is a financial fraud method unique to what AI vendors and other solutions providers refer to in their “fraud detection” offerings. This is because the technique centers on making fraudulent money transfers that appear to be validated by two willing parties.
Instead, fraudsters can attempt to take over the bank accounts of unsuspecting customers or clients and transfer money directly to them. However, AI and other technologies continue to develop and improve at the same time as fraudsters try to find new ways to commit crime.
In this article, we offer two examples of AI-enabled anti-money laundering solutions. Each of the companies claim their software can purportedly deliver a lower rate of false positives to their clients. The anti-money laundering software solutions we explain include:
- Ayasdi AML: Ayasdi’s anomaly detection-based solution that could identify changes in customer behavior and analyze them for patterns related to fraudulent money transfers.
- SAS Anti-Money Laundering: SAS’ solution which could be built upon their data warehouse platform to facilitate data transfer from multiple sources.
We begin our explanation of these anti-money laundering solutions with Ayasdi’s current offering.
Ayasdi’s Anti Money-laundering Solution
Ayasdi is one vendor that offers an anti-money laundering solution to banks, financial institutions, and healthcare companies. The software is an AI anomaly detection application that could detect deviations in customer behavior.
It purportedly analyzes each entity involved in a transaction to ensure a customer is willingly making a payment to a legitimate company or individual while reducing false positives that might be detected with traditional fraud detection methods.
False positives could result in more work for the rest of the team fighting money laundering alerts. Individual banks may not require a perfect false positive rate. However, it is in the best interest of vendors to offer a service that could discern between fraudulent payments that appear legitimate, and legitimate payments that appear fraudulent.
Ayasdi claims their solution could do this by recognizing potentially fraudulent patterns in customer behavior as they develop over time. Ayasdi AML contains five core capabilities:
- Auto Feature Engineering: Detecting data points within transactions and customer behavior with a high potential fraud likelihood.
- Intelligent Segmentation: Benchmarking certain behavioral patterns based on the transaction history and real time behavior of the client’s customer base.
- Behavioral Insights: Tracking daily changes in customer behavior as well as which customers have deviated the most.
- Intelligent Event Triage: Determining whether a given deviation in customer behavior is acceptable or if it needs to be investigated further.
- Contextual Alert Information: Automatically providing the correct information to allow human employees to escalate an alert to investigators.
The graphic below from Ayasdi depicts the flow of data through each of these capabilities in order to provide clients with accurate results. Our readers may find it helpful to refer back to this graphic while reading about each capability in order to get a better sense of how they link together:
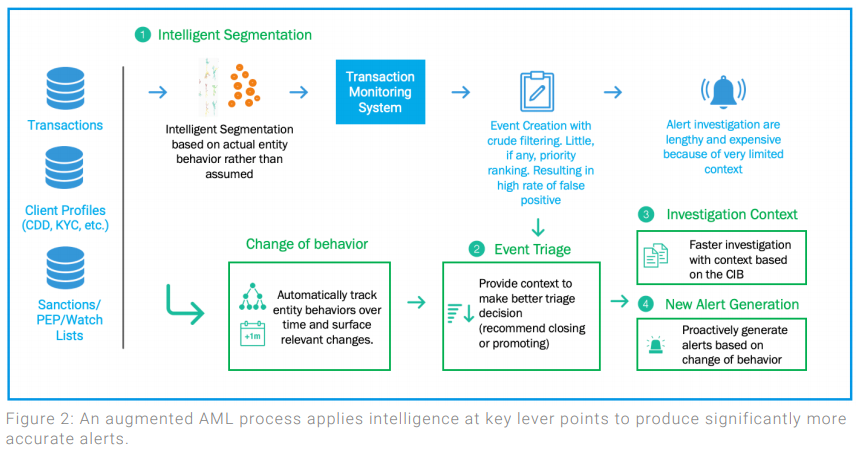
The first of Ayasdi AML’s core capabilities is auto feature engineering which is a core function of finding potential money laundering attempts.
Auto Feature Engineering
Ayasdi’s Auto Feature Engineering tool enables the AML solution to recognize aspects of transactional data that might be considered anomalies related to fraud, but on a more granular scale. This is done so the software could exclusively recognize patterns that are potentially harmful and not acceptable deviations in transaction data. These types of patterns could serve as early indicators of money laundering, and some clients may find it important to keep track of them as they emerge.
Intelligent Segmentation
Intelligent segmentation is another way of exploring how to divide up a customer base so that the machine learning model could analyze a sizeable amount of data at a time. This is done by creating thresholds of customer behavior based on the rate of deviation from each customer’s typical behavior.
Each threshold carries a different level of priority, which allows client companies to focus on accounts that have seen the most deviation first. Ayasdi claims this could also help their clients reduce false positives because more specific customer segments could lead to a more thorough analysis.
Below is another graphic from Ayasdi which shows how more typical customer segmentation tools could produce uneven groups of individuals. This means each threshold must be set lower than the client knows is necessary, which leads to less accurate results and more false positives:
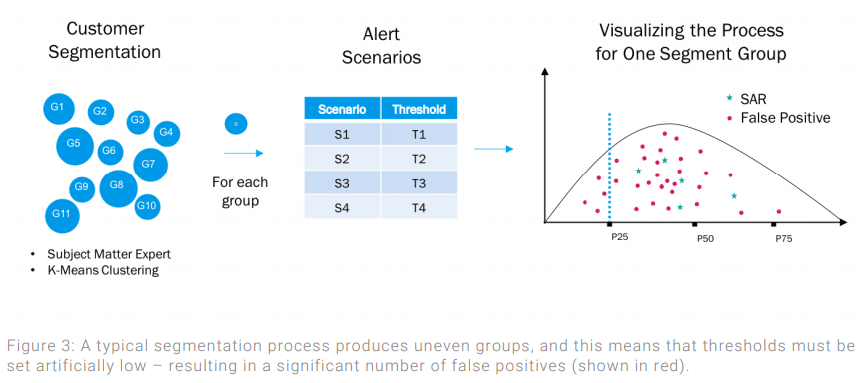
Behavioral Insights
Behavioral Insights is an analysis portion of the Ayasdi AML dashboard which produces daily lists of any changes in customer behavior across all of their transactional data.
Behavior metrics could include how far each customer deviates from their normal behavior over time, or how that rate compares to other customers. This comparison could be scaled to only cover customers in the same segment or could be brought out to the entire customer base.
Using the behavioral insights interface, analysts could organize transactions showing behavioral deviations based on how much their patterns have changed. They could also organize them according to how much each transaction is worth and compare that to the rate of deviation.
This likely helps users close in on individual money laundering attempts as one could track the dollar amounts as they rise along with a rise in transactions with new or suspicious entities.
Intelligent Event Triage
Ayasdi AML comes with an event triage tool that allows for identification of the most important information for investigators to use or analyze in the future. This purportedly results in a smaller amount of events extracted from transactional data than other solutions. That said, these results are purported to have a higher chance of being used to discover money laundering or indicative behavior.
Contextual Alert Information
Money laundering investigators need sufficient context on an alert in order to be able to track down threats. If there is no data that can serve as evidence of an attempt at a fraudulent transaction, an investigator would not be able to find a relevant starting place to do their job. This information might include the ID numbers and account history of the affected accounts, and the exact time the alert occurred.
Ayasdi claims their solution provides this context which also allows them to make faster decisions. Each alert purportedly comes with a visual representation of the behavioral pattern that the solution marked as suspicious. The company also claims the software supplies relevant data regarding this suspicious behavior so that investigators have an audit trail to work from.
Evidence of Success with Ayasdi AML
Correspondent Banking
According to a case study from Ayasdi’s website, the company helped a correspondent bank adopt their anti money-laundering solution. The bank’s initial goal was to improve efficiency in stopping money laundering by 3-5%. That said, they also needed to adapt an AI solution which was a more complex user experience as opposed to their previous manual operations.
Ayasdi worked with their client to analyze the financial messages sent through banking code, or Swift messages, that were related to their customers’ transactions. The company claims this extra source of data helped the bank find new transaction criteria to analyze such as specific attributes of transactional data, geographical data, and chronological data. All of this also made it easier to customize customer segments.
The case study states the correspondent bank reduced their required amount of human investigators for money laundering by 20%. The initial partnership was an eight week process requiring two data scientists working with an Ayasdi project manager.
Two domain experts from the bank were present, which most likely means they advised the Ayasdi staff on individual banking issues. The client bank purportedly moved forward with Ayasdi’s solution and employed it across their entire enterprise.
HSBC Case Study
HSBC Bank worked with Ayasdi to adopt the company’s AML solution, and we have conducted a case study which describes this process in detail. According to our findings, Ayasdi’s solution reduced the bank’s rate of false positives by 20%. Additionally, the bank said that the new technology helped them identify several cases of money laundering as well as behavioral patterns that had direct links to laundering attempts.
HSBC’s IT team worked closely with Ayasdi so that the vendor could access their prior AML data. The bank’s internal modeling review team helped create models for customer behavior that could be easily read by both HSBC’s data scientists and bankers.
SAS Anti-Money Laundering
SAS offers an anti-money laundering solution which they claim can use data sources to find trends pointing to financial crime. A client insurer can use the software to analyze transactions based on data and trends from their existing systems such as CRM, another customer record system, or those that drive customer-facing web applications.
The solution consists of anomaly detection software accompanied by a dashboard with multiple visualization capabilities.
In addition to alerts and event reporting, the software can visualize threats and any associated transactions or customer behavioral trends that may add context. Analysts can use this dashboard to detect possible financial crime before they receive an alert from the software.
Below is a graphic from SAS that explains how users can track which accounts are transferring money and where that money goes.
The “Sankey diagram” purportedly provides records of credits and debits associated with each account in the timeline along with the variation in volume of funds within each entity:
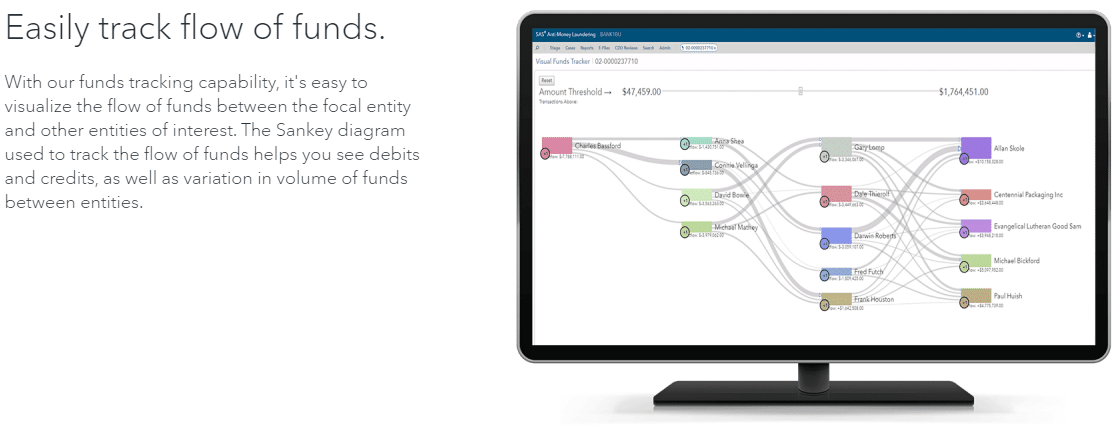
SAS’s website lists a case study in which they claim to have helped Ayalon Insurance in Israel, which is a member of the country’s Financial Action Task Force (FATF).
In order to remain compliant with these new regulations, Ayalon chose SAS as a platform on which to anchor new anti-money laundering and fraud initiatives.
This project was likely facilitated by Ayalon’s previous work with SAS in which they developed a new data warehouse made to organize data more efficiently.
According to the case study, thousands of Ayalon’s employees were already using SAS’ data warehouse daily prior to the adoption of the anti-money laundering solution.
Ayalon states they chosen SAS’ new solution because it can handle highly complex transactions with multiple criteria to check for validation. They also claimed SAS was able to help them finish the project within a short deadline that did not allow for experiments.
That said, AI-powered anti-money laundering solutions would usually require some time for the machine learning model to adjust to the idiosyncrasies of each client data stack. Because of this, Ayalon likely means that the software did not take long to adjust to their current workflows.
Header Image Credit: Investopedia