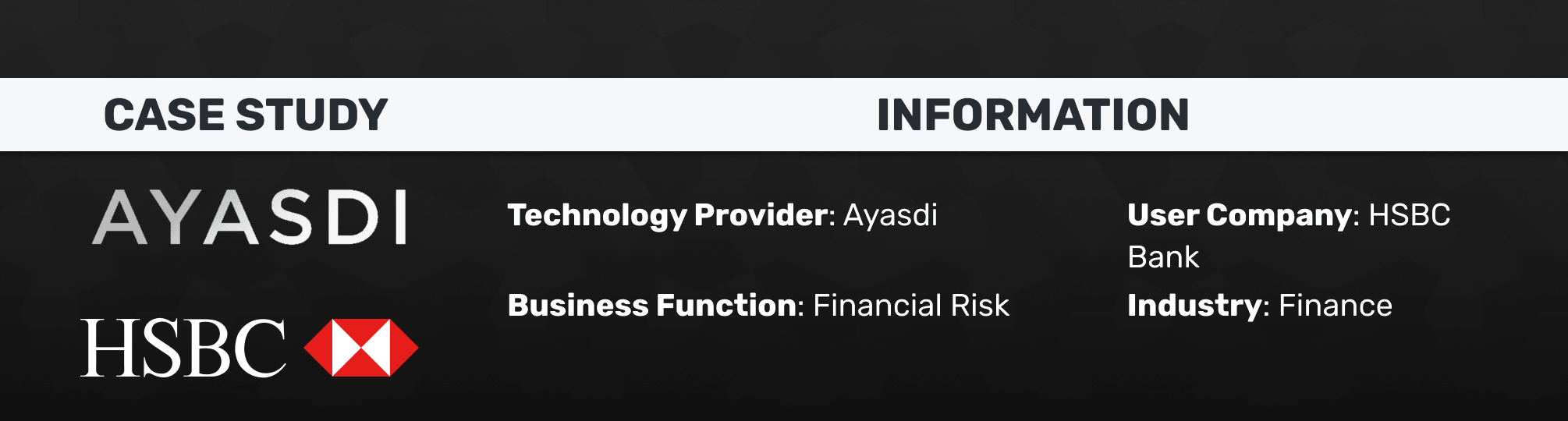
Technology Provider: Ayasdi
User Company: HSBC Bank
User Company Description: HSBC Holdings is a British multinational banking and financial services holding company, with offices all over the world and over 200,000 employees.
Industries: Finance
Applications: Financial Risk
Problem
Industry standard approaches to transaction monitoring and other kinds of financial crime risk detection are largely inefficient, even when they meet regulatory expectations. To become more efficient and target potential criminal activity more effectively, HSBC realized that it had to invest in “automating anti-money laundering (AML) investigations that have traditionally been conducted by thousands of humans” and is working with a number of companies, including Ayasdi, to support this approach.
Estimating the cost of AML efforts is challenging, though a 2006 University of Maryland study estimates laundering-related transactions to equate to around 2 percent of global GDP, or roughly $1.5 trillion annually.
Ayasdi’s Chief Customer Officer, Sangeeta Chakraborty, told us (in an interview specifically for this case study) with AML efforts, Chakraborty tells us, the onus is on the bank to show that they did all that they could to prevent such transactions. Most banks apply myriad rules as filters on transactions (i.e. if you normally do small $200 jewelry transactions, and all of a sudden you send $21,000,000 to Romania, a system might flag the amount, the destination, or both).
These rules, says Chakraborty, are not able to be updated enough (and are not built to be flexible enough) to handle laundering threats adequately. Chakraborty further states that most banks have been employing manual workers to analyze the data and construct new rules, an approach that simply doesn’t scale.
Actions Taken
Ayasdi staff involvement: Data scientist (looked up data / did feature engineering… ran it through the Ayasdi platform. Chakraborty states that the platform enables an unsupervised way of running the data through a variety of algorithms – all with the goal of finding unique groupings and relationships in the transactional data.
HSBC staff involvement: Risk area of the bank. One person who knew the transaction and AML data. The bank also involved it’s IT team to help Ayasdi access and organize the AML data. Chakraborty also reports that all large banks have an internal modeling review group. This groups serves to replicate the efforts of authorities in attempting to validate what the algorithms are predicting, so that it can be understood and explained as easily as possible. Such a group was involved in this application at HSBC.
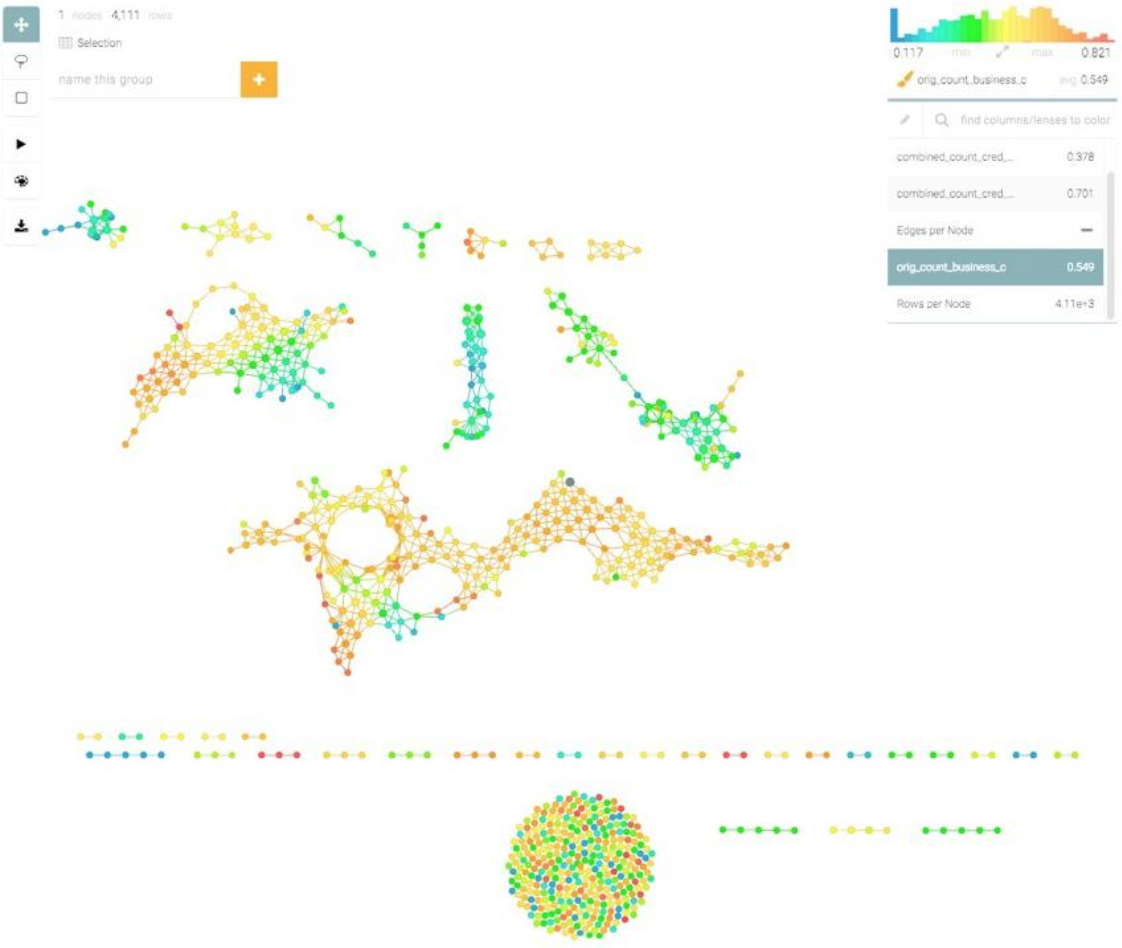
Ayasdi helped to sort it’s analysis into a format so that the bank can make sense of it. This displaying and organizing of results is done within the Ayasdi platform. Ayasdi creates a decision tree to show how the decisions are made within the system, aiming to avoid the “black box” problems of machine learning, which cannot be allowed in the tightly regulated finance sector.
Ayasdi’s expertise is not specific to AML, but in AI approaches and clustering of results. The analysis that follows involves the AML-specific skills and experience of the HSBC teams, who are able to determine the usefulness and meaning behind Ayasdi’s “clusters”, allowing them to strategically decide which of these unique patterns should be permitted to update their AML rules and systems.
Results
Ayasdi claims to have unearthed many new cases and patterns directly correlated to fraud – as well as reducing HSBC’s false positives (cases when HSBC’s existing rules would have flagged for laundering risk when no such risk actually existed) by 20%.
Transferable Lessons
Detecting patterns is what machine learning does best, which is why both fraud detection and cybersecurity applications are increasingly integrating machine learning. In circumstances where adversaries are always trying to find new ways to game the system, machine learning excels in detecting new patterns of behavior and anomalies in behavior.
In addition to the money laundering application above, here are some other areas where machine learning can detect fraud or unwanted customer or user behavior:
- Detecting fraudulent transactions on a credit card
- Detecting fake vs. real user accounts on a social network
- Detecting fake vs. real backlinks as a search engine (e.g. the applications of Bing and Google)
- Detecting unusual user on a company computer, cutting off access if it seems that the user is not who they say they are (for example, if a hacker accesses and employees account and begins using different programs and accessing different files than that employee would normally access)
- And more…
(Note: In 2017 Ayasdi’s Sangeeta Chakraborty was a guest on our AI podcast. You can see her episode published here.)