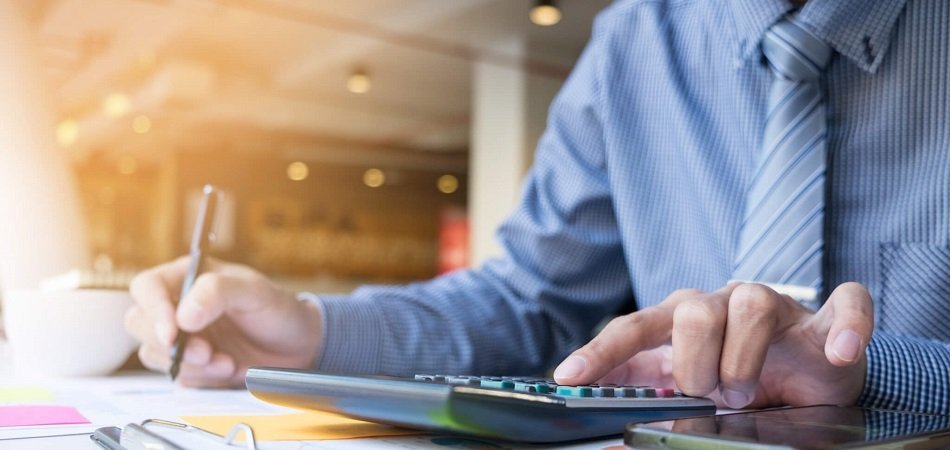
In the banking sector, supervisory organizations create and oversee the compliance rules that banks and other financial organizations need to follow. These compliance regulations are important for companies to carefully abide by, since non-compliance can potentially result in large fines or in extreme cases, even loss of banking licenses.
Compliance regulations are also subject to frequent change, and banks need to constantly update their processes and workflows in accordance with these regulations. Additionally, with fraud and cybersecurity hacking attempts becoming more sophisticated, many regulatory authorities in the banking sector are placing the onus on banks to mitigate these risks effectively.
What this means for bankers is that, in addition to dealing with day-to-day banking operations, banks need to keep track of compliance mandates that are applicable to them. In the last couple of years, new banking regulations such as the GDPR and BASEL IV have made banks scramble to overhaul some of their workflows to meet these new requirements.
In general, most banks have an internal compliance team for this purpose. The compliance teams at banks are tasked with scouring through thousands of webpages and internal documents in order to stay updated with the latest changes in these rules.
Compliance officers also need to make accurate decisions on which compliance rule changes require immediate action from the bank. This takes time, and none of it offers additional revenue to the bank.
AI-based software can help banks and financial institutions improve the accuracy in identifying the rules that apply to them. AI software can help augment the skills of each compliance officer while simultaneously allowing them to scale their operations to do more than what is possible when manually searching through digital documents and government websites. Banks could potentially use AI software to automatically scour through the web and identify only the most relevant ones for each company.
Banks have historically tried to reduce the costs associated with compliance because they don’t drive revenue. Unsurprisingly, banks are already investing money into automation efforts to augment their compliance teams, making them as efficient as possible in accurately identifying which regulations need actions from the banks and in what order.
The figure below, from our AI Opportunity Landscape in banking, the number and percent of AI-based compliance applications across AI vendor product offerings in banking. This is a slice of the full splay of where AI is being applied in banking:
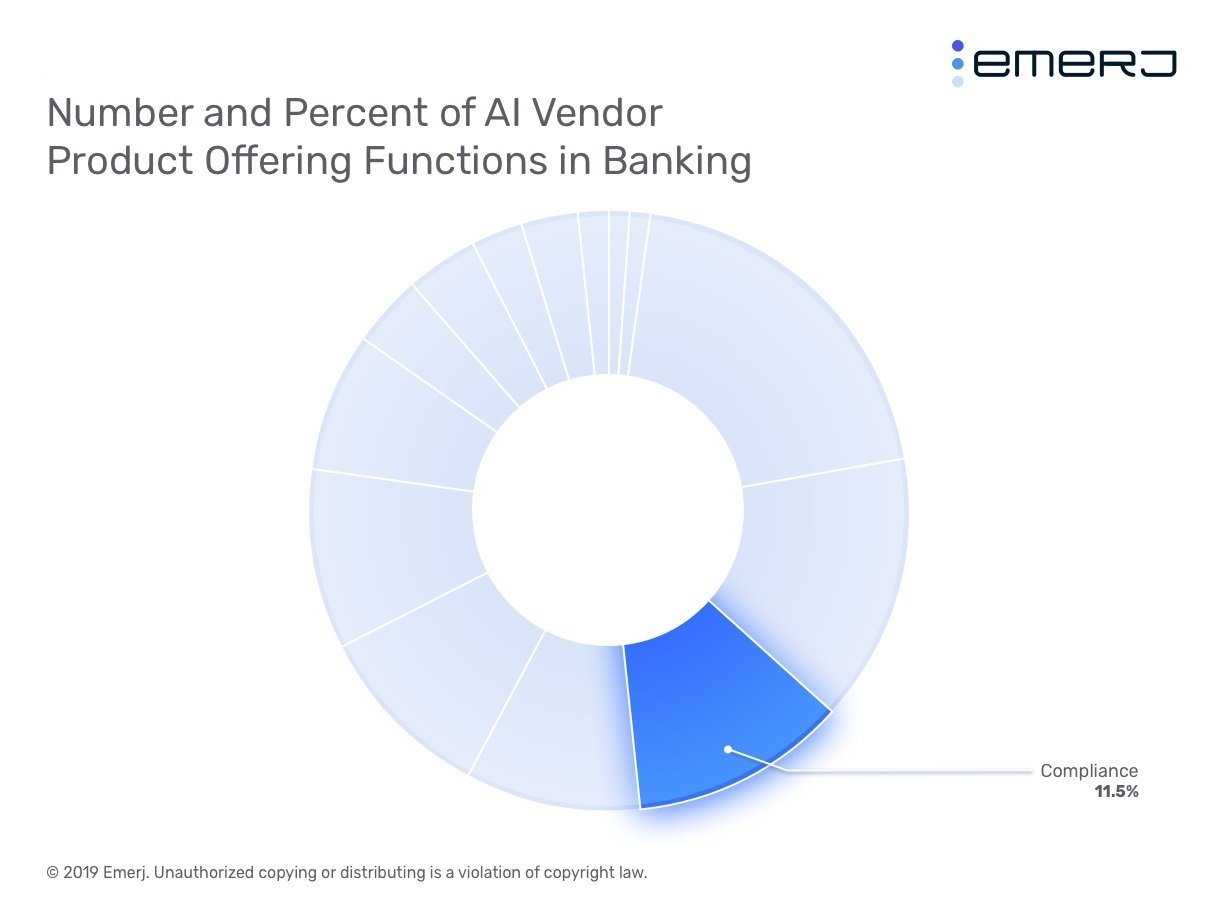
Compliance as a category was ranked third-highest by the number of AI vendor products in banking, accounting for 11.5% of them. In addition, AI vendors offering products for compliance received the second-highest total funding amount ($489 million) compared to other banking functions.
In addition, AI vendors offering products in compliance on average had the highest density of AI talent on their leadership teams and higher than average funding compared to other functions. Of the top six banking functions in our report, Compliance scored the highest on its Average Expertise and Funding Score (3.3 out of 4.0), revealing that AI vendors offering products for compliance are relatively credible and capable firms.
The banking experts we interviewed, including the former head of AI at HSBC, insisted that compliance was one of the main areas of automation focus for banks right now.
In this article, we delve deeper into the following compliance applications in banking using case studies from AI vendors in this space as representative of what is possible with AI today. Specifically, we discuss:
- Natural Language Processing Applications for Compliance: Narrative Science’s Quill and Digitial Reasoning’s Conduct Surveillance for Suspicious Activity Report generation and monitoring employee conversations with clients respectively
- Case Study – Ayasdi: Ayasdi’s case study with Citibank in which the vendor helped the bank pass its Comprehensive Capital Analysis and Review test
We begin our analysis of AI in the compliance in banking space with natural language processing applications for compliance processes at banks.
Natural Language Processing Applications for Compliance
One company offering NLP services for information retrieval in compliance applications is Narrative Science. The company offers Quill, which they claim is an automatic report generation tool. The company claims users of their software can select a data chart automatically generate a few sentences explaining the insights one could garner from the chart. For example, the company claims they worked with an unnamed bank in a project where they helped the bank generate Suspicious Activity Reports (SARs).
SARs are mandatory reports that banks and financial institutions need to file with supervisory bodies. These are reports of any incidents of fraud or cybersecurity threats that a bank has investigated. Narrative science claims Quill-generated SARs are robust enough to assist compliance teams and internal audit teams in ensuring a bank remains in compliance.
Digital Reasoning offers a product called Conduct Surveillance, a natural language processing tool that can purportedly analyze human conversations that have been recorded in either text or voice to determine whether or not a bank’s employees are acting within compliance when they interact with clients. The company claims their software is used by NASDAQ to extract information from employee work interaction records and ensure internal compliance.
Case Study: Ayasdi
AI vendor Ayasdi claims its product, Model Accelerator, uses machine learning to generate AI models appropriate for the client company’s use-case and then allows subject-matter experts to select the most accurately performing models. They claim their Model Accelerator is useful for risk modeling in compliance and regtech.
In a case-study, Ayasdi claims Citibank used the Model Accelerator to help comply with the Comprehensive Capital Analysis and Review, that was instituted in order to prevent another crisis like the one that happened in 2008. In the CCAR test, banks need to forecast and provide a justification for supervisory bodies that their revenues and capital reserves are solid enough to withstand any volatile market conditions.
Ayasdi claims the bank used their software input with historical financial performance data to predict what the impact of different types of volatile market conditions might be on their operations. According to the case study, Citibank had failed the test two out of three times before working with Ayasdi and were able to pass the test successfully after the integration.
Existing Automation Efforts Could Help With AI Adoption
Banks allocate existing effort to compliance automation; they have large IT budgets for these efforts and often sizeable wings of their compliance departments. What this means is compliance is likely one of the first banking functions to undergo transformation through artificial intelligence, along with fraud and cybersecurity.
Banks won’t need to set up new business infrastructure, hire an entire department’s worth of people, and figure out where they can fit in budget for automation like they would in banking functions such as marketing and wealth management. Instead, banks can focus on hiring data science talent and upskilling their existing IT employees, who are perhaps working on automation efforts such as robotic process automation (RPA).
That said, RPA is not AI, and existing IT employees are unlikely to know how to “do AI:” working with data to build machine learning models and then iterating those models. This is to say nothing about the cultural shift that needs to take place at a bank before it’s ready to work with artificial intelligence. AI is not IT; it’s not “plug-and-play.”
Automation wings of compliance departments need to involve both data science personnel and subject-matter experts in compliance, who will often need to be pulled away from their usual work to brainstorm with data scientists and provide them a bounding box for the kinds of models they can build. We discuss this further in our executive guide on the critical role of subject-matter experts in bringing machine learning projects to life.
Challenges With Legacy Systems
The largest banks have been around for, in some cases, over a hundred years, and this affects not only the bank’s culture but its digital infrastructure. Banks still retain many paper documents, and their digital systems and data infrastructures are hardly as up-to-date as tech giants like Facebook and Amazon. Integrating AI into these old systems can be a challenge, and oftentimes, a “ground-up” approach is necessary, in which the bank organizes data lakes and warehouses.
Documents at banks, including those relevant for compliance personnel, have not historically been stored in ways that would make it easy to feed them into an AI algorithm. In other words, banks do not usually have the appropriate digital infrastructure necessary to implement AI search functions for augmenting compliance processes. Laying the groundwork for some AI projects may involve data science expertise and, in fact, other AI applications, such as those for document digitization.
Uncertain ROI and the Importance of AI Leadership
Also, banks might still find that AI projects might not necessarily start giving returns on investment immediately. AI projects can last anywhere between 2 and 6 months before they can be deployed in corporate environments. Even in such cases, banks need to be aware that after all the investment and effort, there is still a chance that existing technologies might perform just as well as the new AI system, at least for an initial period of time during which the AI has not had enough data inputs to learn all the nuances of compliance monitoring.
As a result, risk-averse banking leaders might not be inclined to move into AI until there is a clear, established evidence of returns in the market. Banks might need to hire leaders in their compliance departments who understand what goes into an AI project, with a view of a more long term overall data strategy. Our survey of banking experts found that most cite a lack of a culture of innovation at banks as the major challenge to overcome before banks start adopting artificial intelligence.
Given their focus on automation at present, compliance departments are perhaps among the most likely to hire leaders that can steer the department toward AI adoption. These leaders can serve as a champion for AI, making sure that department personnel understand that AI projects are iterative processes that are not guaranteed to yield an ROI while maintaining enthusiasm for experimenting with the technology. They can hire data scientists and advocate for budget to upskill existing IT personnel so that they might help those data scientists clean and harmonize data for machine learning projects.
That all said, despite the associated uncertainty with AI projects, engaging in these projects is the fastest way for banks to understand what goes into developing such as system and gain data handling capabilities that will serve them well in the future.
Header image credit: Analytics Training Blog