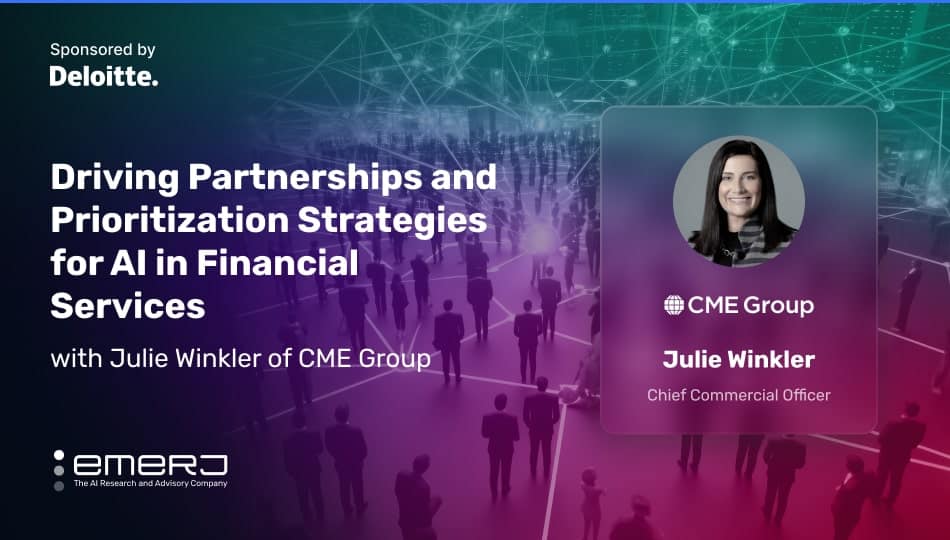
Where new, emerging capabilities in AI mean new opportunities for financial institutions – especially those that operate financial exchanges, where data and predictive analytics are paramount – the palpable risk of failure in AI adoption initiatives can prove a significant hurdle.
According to MIT Sloan’s Artificial Intelligence Global Executive Study and Research Project, 40% of the organizations making significant investments in AI do not report business gains from AI. Another survey from the AI Infrastructure Alliance (AIIA) published in 2023 found that 63% of respondents reported their losses were $50 million or higher. Additionally, Cognilytica, a research firm trusted Deloitte and other Fortune 500 brands, regularly cites statistics that AI project failure rates remain in the 70-80% range, with many projects either never completed or falling short of meeting their objectives.
For those firms operating financial exchanges, these risks are well worth the potential benefits. A 2021 report from the OECD highlights the value of AI in financial markets for policymakers, including the potential to improve decision-making, enhance efficiency, and reduce costs. AI and machine learning models can analyze vast amounts of data, enabling more accurate risk assessments and better investment decisions. These technologies can also help investors of all backgrounds make more informed trading decisions and potentially improve trading performance.
Emerj CEO and Head of Research Daniel Faggella recently sat down for a conversation on the ‘AI in Business’ podcast with Julie Winkler, Chief Commercial Officer of the CME Group — which operates a number of derivative exchanges in the Chicago area, including the Chicago Mercantile Exchange and the Chicago Board of Trade, among others. Together, the pair discussed strategies for implementing AI initiatives effectively within an organization while prioritizing impact and efficiency.
The following analysis of their conversation examines key insights on overcoming two main obstacles to AI adoption:
- Prioritizing impact and efficiency: Prioritizing AI initiatives based on the time and effort saved by implementing technology solutions, focusing on areas with the highest potential impact and transparency in decision-making processes.
- Fostering adaptive learning for AI initiatives: Cultivating a learning culture in the enterprise by allocating dedicated resources to AI initiatives that ensure the agility to navigate challenges and seize opportunities in the rapidly evolving AI landscape.
Listen to the full episode below:
Guest: Julie Winkler, Chief Commercial Officer, CME Group
Expertise: Innovation Management, Growth Strategies, Financial Product Development
Brief Recognition: Julie Winkler leads CME Group’s sales, product marketing, research and product development, as well as the organization’s retail, data services, data science, and innovation lab. She holds a bachelor’s degree in business administration in finance from Valparaiso University and an MBA in finance and derivatives markets with highest honors from Loyola University.
Prioritizing Impact and Efficiency
Julie begins her podcast appearance by reflecting on both the importance of, and her firsthand experiences with, engaging a broader stakeholder group in the adoption of generative AI (GenAI) technologies. She emphasizes the need for excitement and buy-in from various departments, recognizing that the potential use cases of AI span across different areas within the organization.
She describes how data teams at CME Group were able to secure senior sponsorship to ensure effective implementation, with the Chief Information Officer and herself leading the initiative. They divided the work into different areas of deployment, such as code development and go-to-market strategies, aligning responsibilities with their expertise.
She acknowledges the necessity of involving support functions like information security, compliance, and legal teams along the journey. However, Julie also highlights the importance of a small decision-making team for finalizing policies and making critical decisions integral to their success:
“I think, in taking full advantage of AI – the machine learning aspects, and also the GenAI tools – because it’s going across multiple functional areas and applications, the fact that we had already moved a lot of our data into Google Cloud and had some central sourcing has allowed us to just move much, much faster. You’ve got to find the teams that have the bandwidth to do that. For us, that has also meant moving into a more agile approach and an operating model that dedicates more of our resources to working on these things, which I think is also important. What we found in some previous models is we were expecting people to dabble in a lot of different things and it wasn’t helping us get the velocity, the throughput, and the focus that we’re now seeing as we change more of this operational structure.”
—Julie Winkler, Chief Commercial Officer, CME Group
She then explains at length how CME Group came to leverage AI for market regulation and integrity for over a decade. She sees the potential for commercial applications, particularly with GenAI, where they envision significant value added to current systems. As mentioned in the quote above, Julie attributes part of their success in these areas to their partnership with Google Cloud, which has provided them with early access to GenAI tools.
She also highlights their organization’s focus on providing products and services while managing risks in a constantly evolving industry. Given their large customer base and relatively small sales team, Julie sees AI as a means to achieve scalability more rapidly than traditional methods. At which point, as she says, “Deployment is just around the corner.”
For Julie and her team, the journey with AI began with a focus on centralizing the gathering and study of use cases across the organization. A centralized approach to the process allowed them to effectively assess each use case’s potential impact and strategic importance.
Working with CME Group’s Chief Information Officer, Julie’s team prioritized these cases based on available resources and overarching goals. Doing so ensured that resources were allocated to the most critical areas of the project while acknowledging the importance of long-term strategic planning:
“As teams would bring their use cases to us for consideration, one of our initial questions back to them was: How much time is your team spending doing this work without this technology today? And that became a really difficult question for teams to answer [by] measuring the productivity across teams, looking at how intensive these manual processes were, but that, for us, was a good northstar [for] identifying then what rose to the top, and what were some things that we were willing to invest in out of the gate.”
—Julie Winkler, Chief Commercial Officer, CME Group
Promoting Adaptive Learning for AI Initiatives
Julie mentions numerous policy points and strategies that helped her team build suitable AI projects from their gathered use cases:
- Clear GenAI Strategy: Aiming to position CME Group “in the middle of the (adoption) wave,” neither ahead nor behind, but transparently within the innovation curve.
- Transparency and Communication: Being transparent with the organization about their list of use cases and intentions, ensuring alignment and clarity across the organization.
- Exploration of Proof of Concepts: Identifying opportunities for proof of concepts with external vendors to test potential solutions and gain insights.
- Supporting Experimentation: Supporting and enabling teams to experiment with AI technology to understand its potential and determine where to invest resources.
- Agility and Adaptability: Recognizing the need for agility and the ability to pivot, acknowledging that frequent ‘micro-pivots’ are inevitable in such wide-ranging initiatives.
- Learning Culture and Dedicated Resources: Emphasizing the importance of promoting a learning culture and dedicating resources to AI initiatives that facilitate adaptation and growth.
- Building Strong Relationships: Highlighting the critical role of strong relationships with other functional teams the enterprise, such as legal, compliance, and information security, in overcoming challenges and obtaining necessary approvals.
Julie further explains two main areas where her team implements AI solutions: CME Group’s virtual assistant and internal use cases, particularly in marketing and sales.
Firstly, she discusses the challenge of managing a vast amount of information on their website, which currently comprises 68,000 pages. The website aims to provide comprehensive information and educational material about their products and markets to existing and potential clients.
However, with such a large content volume, it takes time for clients to find the information they need effectively. While measuring the exact difficulty clients face in navigating the website is challenging, CME’s data team recognizes that the existing search functionality needs to meet their needs effectively.
Secondly, Julie highlights the internal use cases, particularly within the marketing and sales teams, where AI can significantly impact productivity. These teams spend considerable time summarizing, mining, and merging vast amounts of data to gain insights into customers and prospects.
Tools like Salesforce provide a baseline for understanding the time spent on these tasks. The aim is to leverage AI to transform that data into actionable insights, enabling the sales and marketing teams to act more quickly and efficiently.
Julie emphasizes the importance of involving end-users in developing and testing these AI tools. By including those who will ultimately use the tools in their day-to-day work, they ensure that the solutions meet their needs and are user-friendly. That feedback loop, she says, is critical in making incremental adjustments along the way, ensuring that the AI solutions effectively align with the team’s workflows and objectives.
Julie outlines critical factors for successful AI deployment, summarizing them into several points:
- Clear centralization: Establishing a transparent, centralized approach for evaluating and prioritizing use cases that ensures all relevant stakeholders are involved and aligned in decision-making.
- Senior sponsorship: Support from executives like her and the CME Group C-suite is essential for driving AI initiatives forward and gaining organizational buy-in.
- Evaluation process: Establishing a robust method for evaluating use cases based on factors such as commercial value, feasibility, and alignment with the organization’s risk appetite. A more robust approach ensures that resources are allocated to the most impactful and achievable projects.
- Comprehensive education program: Ensuring employees understand the capabilities and risks associated with AI tools. Employee education programs also help build confidence and adoption among users, especially customers.
- Automated testing process: Monitor model changes that ensure testing doesn’t have to start from scratch with each model update. Partnering with experts can streamline the process and drive the velocity of changes effectively.