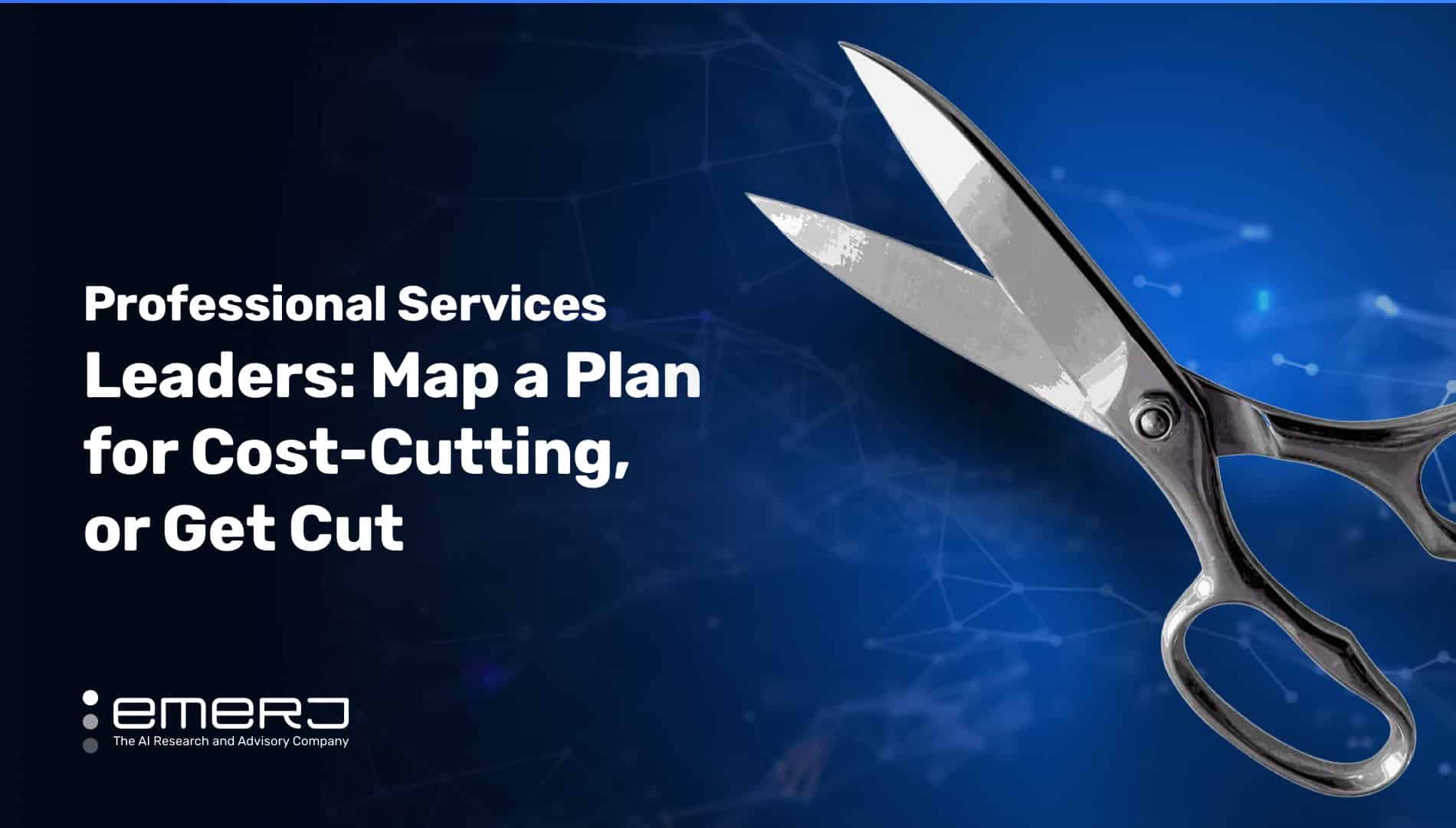
What to do when your clients want to cut costs?
Help them cut costs, and be part of a bigger vision beyond cost-cutting.
The coronavirus hasn't been friendly to most consultants and professional services leaders - as enterprises clamp down on spending. It's hard to make strong predictions about what the coming months and years will bring - and the only certainty most companies have is the need to conserve capital.
While there might be less money circulating in the economy, there are new sets of technology and strategy priorities emerging (a topic we've covered in our previous Emerj Plus articles) - which present new opportunities to professional services firms that are smart enough to get ahold of them.
In this article, we covering three critical factors that will separate the winners from the losers in terms of AI ROI during the crisis - and we explore four unique categories of cost-cutting AI and automation applications - including reference links to help readers find sam...
You've landed on exclusive content for Emerj Plus Members
Emerj Plus Membership
In-Depth Analysis
Consistent coverage of emerging AI capabilities across sectors.
Exclusive AI Capabilities Matrix
An explorable, visual map of AI applications across sectors.
Exclusive AI White Paper Library
Every Emerj online AI resource downloadable in one-click
Best Practices and executive guides
Generate AI ROI with frameworks and guides to AI application