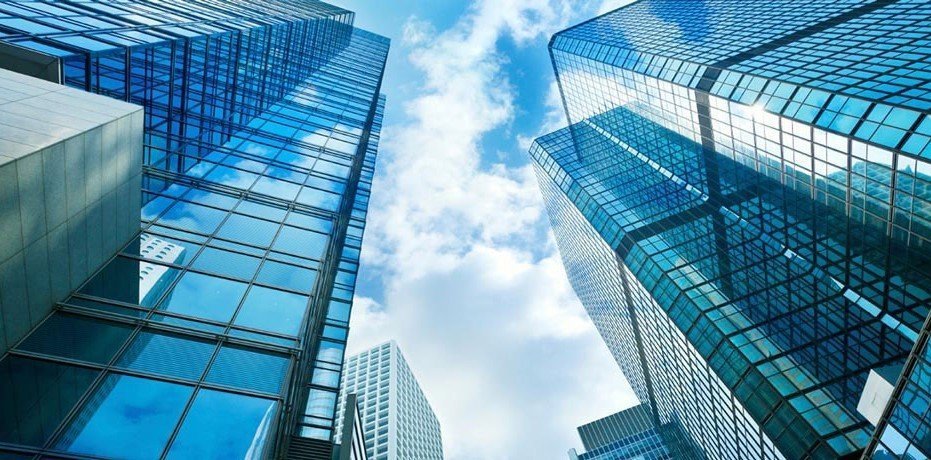
We discussed the difficulties large businesses may have in adopting AI in our previous article; despite this, last month we fleshed out the reasons why it’s still more difficult for small businesses to apply AI than the enterprise and how they might catch up to larger businesses in the future.
In this article, we draw a further distinction between B2B companies and B2C companies. It may be more difficult for B2B companies to adopt AI than B2C companies for a variety of reasons. We will focus on why that might be the case, laying out what we believe to be the starkest differences between B2B and B2C companies when it comes to applying and building AI products that could drive business value. These differences involve six key strata:
- Privacy and Aggregate Data Use
- Data Volume
- Interpretability and Transparency (The Black Box Problem)
- Risk and Experimentation
- Regulation and Legal Concerns
- The Culture of Data Science
That said, we want to make it very clear that this article seeks to provide a very general overview of the possible differences between B2B and B2C companies when it comes to adopting AI. In no way are we stating that in every scenario will B2C companies have an easier time integrating AI into their workflows than B2B companies; we simply believe there to be signs that point toward more difficulty for B2B companies.
Business executives may appreciate these dynamics more by considering that most successful companies that claim to leverage artificial intelligence in their respective industries are B2C: Facebook, Google, Amazon, Netflix, Alibaba, and Tencent, for example. We believe it is not coincidence that dominant companies that claim to do AI like these are B2C. Knowing about the possible barriers to entry for B2B businesses when it comes to adopting AI could help B2B executives make more strategic decisions when considering an AI solution for their business problems.
Privacy and Aggregate Data Use
B2C companies may find managing privacy concerns easier than B2B companies for a number of reasons, the first of which has to do with consent. B2C companies like Amazon and Facebook often have their customers or users provide explicit consent for the way the company intends to use their data, by checking a box or clicking a link that signals agreement with the companies’ terms of service. The B2C company would then have express license to do what it wants with the information its customers and consumers provide assuming they check that box or click that link.
Consumers tend to understand that agreeing to a company’s terms of service means giving the company permission to use their data, including their demographics, on-site search history, and purchase history, to make make business decisions or market to them. However, most consumers are not going to carefully read 30 pages of legal documents to find out exactly to what they are agreeing; they simply want to purchase the product or service.
For the B2C customer, allowing a company to do what they want with their data is a reasonable exchange for access to a company’s product or service, especially since the data the B2C company wants from their customer is generally not nearly as sensitive as a client company’s data.
For this reason, B2C companies may need to worry less about their terms and conditions; consumers are often willing to allow companies to collect data on them for marketing purposes in exchange for the product or service. This is not usually the case in the B2B world where data privacy is of much greater concern to businesses that want to protect their proprietary information and processes.
Typically, if a B2B AI company wants a business client to agree to let the company use its data for marketing, product development, and other purposes, that client is going to scrutinize the terms of that agreement. The client may involve lawyers, ask for the addition of special clauses tailored to it, or request revisions to the terms before anyone signs anything.
For example, if an oil and gas company wants IoT sensors on all its rigging equipment, it may want to know what will happen to the data the IoT sensors collect behind the scenes. This is especially true if that data is collected by a B2B AI vendor company.
In many cases, the client may not permit an AI vendor company to use its data to improve the company’s machine learning model for other clients. Facebook and Google can train their machine learning models on billions of user interactions with their platforms, which improves the models on which their products and services are built. When a client business refuses to let a B2B AI vendor access its data in a way that allows the vendor to train its machine learning models on that data, those models improve less.
In addition, each client is bound to have a unique privacy agreement with the vendor company. Unique client arrangements make it more difficult to train machine learning models. The models may end up biased toward the type of businesses that agree to let the vendor company train its models on their data.
Data Volume
Billions of people use Facebook and Google. This means they have a large volume of data, and a large volume of data is what machine learning models can use to find patterns in a company’s database or make predictions based on that database. This can help them make their products and services better and make the business more profitable, as previously discussed.
The same applies for smaller B2C companies with a large volume of transactions. For example, an eCommerce toy company making $80 million a year by making a hundreds of thousands of sales a month will collect a large volume of sales data. This is likely more than enough data on which to train an AI model, which requires that volume of data to pick up on patterns in the company’s database or provide any acceptable level of accuracy with its predictions.
In contrast, a B2B AI vendor that sells IoT sensors for heavy equipment and also makes $80 million a year might arrive at that level of revenue quite differently. This company might only have 10 clients; each client might use the company’s sensors for different types of machinery. Even in the event that each of the 10 clients permits the vendor company to use its data to train the vendor’s machine learning models (which might not happen), it is unlikely that the vendor company will receive enough data from those sensors on which to train their machine learning models.
In other words, the data from 10 clients is simply less voluminous than the data from a billion users. The machine learning model might not find any patterns within a database of 10 clients’ data, especially when that data is from a variety of machines.
For Facebook and Google, a click or a search are the same thing across billions of users; the data is analogues. For B2B AI vendor selling sensors for heavy equipment, the way oil and gas machinery breaks down might be completely different from the way a construction vehicle breaks down. The data is not analogous, and so if the vendor company’s database serves a predictive maintenance application, the machine learning model might make less accurate predictions.
The fact is that in order to train AI models, a large volume of data is required. B2C companies obtain this data more consistently than B2B companies. A B2B oil and gas company might increase its revenue from $80 million a year to $150 million a year by closing a few deals with a handful of airline companies. That wouldn’t provide the company with as much data as what a B2C business would acquire if it also made a leap from $80 million a year to $150 million a year. The B2C company requires significantly more transactions—more sales data—to increase their revenue that much.
Interpretability and Transparency (The Black Box Problem)
The next difference between B2B companies and B2C companies when it comes to adopting AI is a problem that is central to AI in general: the “black box” problem. The black box problem dictates that no one can explain how or why a machine learning model arrives at the patterns it finds or the predictions it makes. This problem is much more of a challenge for B2B companies to overcome than B2C companies.
B2C companies generally don’t need to explain how or why their AI models arrive at the conclusions they do. For example, consider Netflix or Amazon. Neither company is likely able to explain why its recommendation engines recommend certain content or products to a given customer. That said, neither company is under any obligation to explain how or why for two reasons.
The first is that Netflix and Amazon customers are one of hundreds of millions. Individual customers do not have the power to demand an explanation for their recommendations from Netflix or Amazon, nor do they have access to anyone who might possibly be able to provide that explanation even if they could. This is especially the case because Netflix and Amazon provide free or almost-free services.
This does not mean that these companies do not value their customers, but if one individual were to stop being a customer, Netflix and Amazon are not going to experience any serious financial trouble. As a result, the leverage that customers have over Netflix, Amazon, and similar B2C companies have over the B2C companies from which they purchase is little to none.
The second is that there isn’t much reason for Netflix or Amazon to explain how or why their machine learning models reached the conclusions they did. Most customers won’t question their recommendations, and in fact they will be satisfied with them. It’s of no consequence to a customer why they were recommended a product; they simply won’t buy the product if they don’t like the recommendation.
From a business perspective, all that matters for Netflix and Amazon is that customers watch more shows or purchase more products as a result of receiving personal recommendations. It doesn’t matter how or why a customer was recommended something if they take the recommendation and increase their lifetime value.
In other words, the occasional poor recommendation often doesn’t pose any real risk for B2C companies and their customers. However, Transparency is much more an issue for B2B companies because the risks are higher, and clients have the power to demand explanations in most cases.
For example, let’s say Salesforce sells a CRM to do lead scoring for a client company that pays it $200,000 year. The client is going to want to know how and why the machine learning models behind the lead scoring system are scoring leads the way they are if the client has been missing sales targets for two quarters while using the system. A faulty lead scoring system could result in consequential losses for a client company.
Since the client is paying Salesforce $200,000 a year, it has the power to demand an explanation from Salesforce and to expect one. If Salesforce is unable to explain why its models are scoring leads the way they are, not only might the client company stop being a client, it might sue Salesforce for not following through on its commitment to do accurate lead scoring. Salesforce might then be out $200,00 a year and have to engage in a legal battle with their former client, costing even more.
The case is the same for vendors selling anti-money laundering or fraud detection software to a client bank. If the bank is paying the vendor $1 million, it will want to know why the vendor’s machine learning models are flagging certain transactions as fraud and not others. If the software fails to flag a transaction as fraud or flags a good transaction as fraud, it could result in serious consequences for the bank. In other words, the risks are high.
In summation, a B2B company is more likely to have clients with the leverage to demand explanations, and the software it uses is more prone to causing problems for the company when it makes an error.
This means that AI vendors selling B2B might need to be more transparent about their machine learning models. This is a highly technical problem across AI in general. Some of the brightest minds in artificial intelligence are asking how to achieve transparency in machine learning engines.
In fact, computer scientist and “Godfather of AI,” Geoff Hinton, who splits his time working with the University of Toronto and Google, talked about how we might need to rethink doing machine learning and artificial intelligence entirely because it is not explainable enough. He goes so far as to say, “My view is throw it all away and start again.”
Risk and Experimentation
At this point in the article, we’ve discussed how a machine learning model’s faulty conclusion or prediction could pose serious risks for companies, but discussion of risk warrants its own section.
As previously mentioned, if Netflix makes a recommendation for a movie in which a customer is not interested, there is no real harm done. However, if a bank’s anti-money laundering software fails to flag suspicious transactions correctly, it could stand to lose a significant amount of money, as well as possibly worse consequences, such as a loss of prestige and credibility.
A large eCommerce company can experiment with an AI software that claims to handle a large volume of email tickets to see how much it might save on customer service costs. If the software sends the incorrect canned response to a customer, it might make things difficult for the customer, and it isn’t good, but the consequences are not catastrophic for the company at large.
The company can handle a few mistakes if they’re dealing with a high volume of tickets; that mistake is one out of possibly thousands of positive interactions between the software and the customer. The company might incur costs from the software’s mistakes, but the returns might make those mistakes worthwhile.
On the opposite end of the spectrum, machine learning software that claims to aid doctors in diagnosing cancer can’t make a mistake in diagnosing or failing to diagnose a patient with cancer because the consequences are life and death. From a business perspective, the hospital system that uses that software risks millions in legal fees and settlements when the software makes a mistake.
Regulation and Legal Concerns
Although regulation is less of an issue as it stands currently when it comes to AI technology, there are certainly still regulations that companies may want to consider.
In healthcare, for example, data is much more sensitive than in other industries. This data ranges from medical data to financial data. The Health Insurance Portability and Accountability Act of 1996, or HIPAA, protects the privacy of medical information, for example.
AI vendor companies selling into the healthcare space are often responsible for complying with regulations regarding patient data privacy. If a hospital system with a large number of patients wants to find a way to aggregate patient data to figure out the most cost-effective ways to treat diabetes under different conditions, the AI vendor company that provides a software to do just that will need to comply with HIPAA and other regulations.
The same is often the case in the financial industry. Banking regulations are often strict about the privacy and security of customer data. AI vendor companies offering anti-money laundering software to a commercial bank will have to work with the bank to make sure its software complies with regulations.
The same regulations apply to all businesses with respect to relevant industries. This means both B2C and B2B companies will need to figure out how to comply. B2B companies, however, typically sell into industries with much more stringent regulations than B2C companies.
That said, some B2C companies work with sensitive data. Amazon, for example, collects a large volume of credit card information. As a result, Amazon has had to focus its attention of security. It holds many more patents for security applications than Facebook, for example, because it accepts many more credit cards into its system than Facebook. We discussed the intellectual property landscape of both Facebook and Amazon in a previous report.
In most cases, however, B2C companies have to worry less about laws and regulations regarding data than B2B companies. There are no laws regulating how Netflix and Facebook use the data they gather from clicks or laws regulating how Huffington Post tracks how many articles a user opens. That may change in the future, but it is not the current state of things.
This is not the case for the data B2B companies handle on a daily basis, which is often sensitive. Medical and financial information is very often subject to regulations that seek to protect it.
The Culture of Data Science
Many of the big players selling B2B tend to be older than those selling B2C. For example, Amazon, Google, Facebook, and Netflix are B2C companies, and they are young compared to JP Morgan Chase.
The B2B world typically includes banks, oil and gas companies, manufacturing companies, chemical companies, and construction companies, among others. The biggest companies with the largest market shares in these sectors are much more likely to be companies that are 50 years old or older.
On the other hand, the online media, eCommerce, and retail spaces have seen a significant amount of growth in a comparatively short period of time, and they tend to sell B2C. We see young, successful companies with a firm grasp of data science. This is because they are digitally native.
It was probably very difficult for JP Morgan Chase to switch from paper files to digital files. This is not the case for a company like Facebook, which was born into the digital world in which everything is trackable and testable.
This may change in the next 10 years, but as of now it is more common for a B2C company to be digitally native than a B2C company. Many of the fastest-growing companies doing the most with artificial intelligence are selling B2C right now.
These B2C companies have the kind of appeal and profit potential to attract talented data scientists. The early employees at these companies understood the technical underpinnings of the company’s software. Since their background is in the B2C context, they are more likely to continue developing for B2C companies.
Key Takeaways for Business Leaders
Broadly speaking, B2B companies may face more challenges when adopting AI than B2C companies in the next five to 10 years. We come to this conclusion having conducted hundreds of interviews and read thousands of surveys with and from AI company executives and machine learning researchers. These folks have worked on the business applications of AI and on the hard science. We believe the six strata discussed in this article are representative of the problems B2B executives are facing when it comes to adopting and garnering ROI from AI solutions they purchase from vendor companies.
Once again, this article does not intend to purport that every B2B company is going to have a harder time integrating AI into their workflows than B2C companies. There are many crossover points and gray areas between B2B and B2C companies, and so the ease of AI adoption is largely on a case-by-case basis.
The best way for business executives to make use of the information in this article is to ask how each of the six strata presented apply to their businesses. For example, business leaders can ask themselves how much transparency will be necessary for their clients when it comes to the machine learning models they plan to use. When it comes to the culture of data science, business leaders can ask themselves how difficult it might be to find the AI talent necessary to build a solution for their businesses.
The core hypothesis behind the article and a point that we would like to drive home is that while all business leaders should look into the six strata we discuss, those selling B2B may want to look a bit deeper into some of them.
Header Image Credit: JPCRE, Inc.