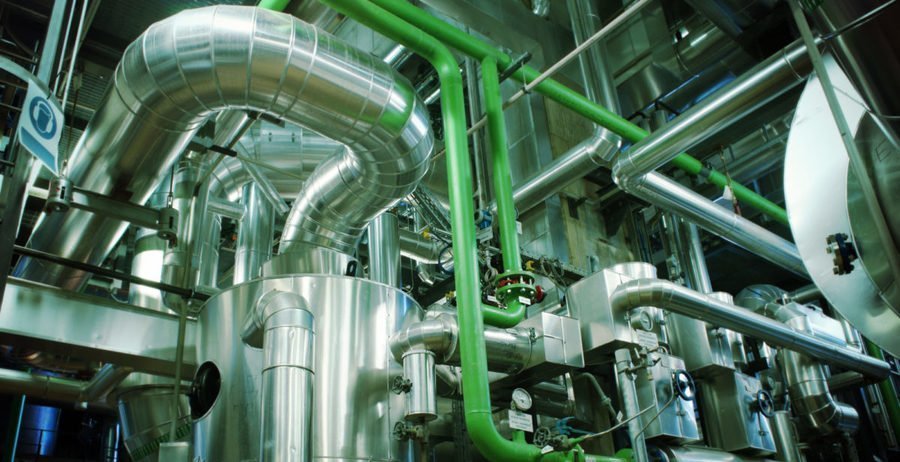
With the entrance of artificial intelligence and its capabilities of recognizing temperature, vibration, and other factors from sensors pre-built into machinery and vehicles, business leaders in heavy industry might be interested in the possible opportunities of predictive and preventative maintenance applications.
While predictive maintenance allows manufacturers to attempt to predict how long a piece of machinery will last, preventative maintenance involves repairing the machinery to keep it working longer.
A 2017 report from Plant Engineering found that 51% of manufacturing companies now use a computerized maintenance management system (CMMS).
With the digitization of many manufacturing plants and the reported costs of reactive maintenance needed to fix machinery in mind, we have put together a list of five companies that claim to offer software using AI for predictive maintenance.
These companies offer software for use in two applications:
- Asset Performance: The company’s primary goal is using sensors or other data to determine and notify manufacturers when a piece of machinery is broken or not working.
- CMMS Systems: Systems that both monitor asset performance and allow users to assign and track repair work orders.
With this report, we hope to give business leaders in heavy industry insight on how companies claim to use AI to help manufacturers avoid high maintenance costs.
Asset Performance
Maximo Asset Health
Launched by IBM, Maximo Asset Health uses data from IBM Watson and sensors prebuilt on to machines to determine if and when a piece of machinery or part will need maintenance. IBM claims the software also takes factors into account including the machine’s age and maintenance history.
While we could not find a demo showing what the interface looks like for a user, the 1-minute video below demonstrates how the product might be used:
Maximo Asset Health lists clients such as AGR Group, Australian Maritime Systems, Brisbane Motorway Services, ConnectEast, CNR International, Drax Power Station.
According to a case study, AGR, which provides on-site maintenance services for oil and gas sites, approached IBM with interest in upgrading maintenance and workflow systems on their clients’ worksites. Because the systems were out of date, the study claims that finding data and problems with machinery became time-consuming and costly.
The company upgraded their clients to Maximo and was, according to the study, able to consolidate all of the sites’ maintenance systems into one. They were also able to identify and report machinery problems on different sites more quickly and in a prioritized manner based on the readings from the software.
Many team members affiliated with IBM Maximo do not have a master’s in computer science or a related AI field but have held software engineering roles within the company for many years.
Progress DataRPM
In 2017, Progress, which provides IoT and data-driven solutions to clients in various industries, purchased the DataRPM predictive maintenance software for $30 million.
The resulting Progress DataRPM product allows those working with machinery to track a machine’s health through data collected from sensors pre-built within the machine, according to Progress.
A demo video or simple how-to guide could not be found for connecting and implementing the software. However, once it is connected, it seems to be similar to Maximo in that the company claims it uses sensor information on machinery to determine if the machine is slowing down or may need maintenance before it breaks down on the job.
DataRPM said they assisted companies in connecting the sensors prebuilt into the companies’ machinery to their software. The company claims they can format the data collected from those sensors into formats such as a data lake or a user-friendly dashboard.
After integration, a user, such as a manufacturing supervisor, could log into a panel with an Insights tab. From the tab, they can click on a machine and see its “Storyline,” which shows each of the software’s analyses of it.
Each analysis shows how many anomalies were expected based on factors of the machine, such as age and maintenance history. After showing the expected anomalies, it will then show the number of anomalies that actually occurred during the test the software runs on the machine. If more anomalies occur than expected, it would signal to maintenance personnel that a repair may be necessary for the machine.
In a case study, one unnamed Fortune-100 manufacturing company approached Progress looking to optimize and quicken the pace of machinery fixes. The company was also looking to decrease funds on the inventory of spare parts that were not needed but purchased as a precaution, according to the study.
The study did not give any further details about how much time or money these fixes could have been costing the company, but it noted that spare-part inventory costs were lowered by 57% as “worker efficiency” increased by 8%, although we do not know how “worker efficiency” was measured. The DataRPM software allowed the company to assign other tasks to employees with downtime during a planned fix. As such, the unnamed company claims this increased productivity of workers by 38 percent.
Progress does not list clients for DataRPM. This could be due to their recent acquisition of the software.
Manufacturing clients who use Progress’ other data-driven products include the International Energy Service, FDM4 and Parker Hannifin. Progress’ automotive clients also include Toyota, Kauffman Tire, and ASA Automotive Services.
DataRPM co-founder and CTO Shyamantak Gautam previously served as a technical architect at IBM. We could not find evidence of his academic credentials, and so we can not confirm if he has a firm background in artificial intelligence.
While Progress has multiple data scientists with academic backgrounds in computer science, we could not find direct leadership with a robust AI or machine learning background. Dmitri Tcherevik, CTO of Progress, received his MS in Computer Science from National Research Nuclear University MEPhI.
Predii
Predii offers a platform where a user can log in and see alerts about maintenance that may be needed to prevent machinery breakdowns or a guide to fixing a piece of machinery.
Like the software detailed above, the company claims it integrates with sensors already built into the machinery. The company claims to assist machinery supervisors in areas relating to automotive, maintenance and repairs, and manufacturing.
It seems that Predii’s software is first connected to a fleet of machinery and its sensors. Then, a user who works with the machinery can open a dashboard which will notify them if a piece of machinery is not working or could be failing based on data collected by its sensors. From this notification, it seems that a user can click on a guide that tells them which specific parts must be replaced or how else they could fix the problem.
While we could not find a demonstration showing how to use the software, this 4-minute promotional video briefly shows screenshots of the product and explains how it can be used:
The company does not list any case studies nor clients on its website, but Predii claims that its leadership recently met with NASA to discuss Big Data. The company also claims to have a partnership with Snap-On Diagnostics, a car diagnostics software company which uses Perdii to power their predictive maintenance application.
The company’s CEO, Tilak Kasturi, holds an MS in Computer Science from the Florida Institute of Technology. He was also the Chief Architect for Time Warner’s interactive TV platform. We previously interviewed Kasturi about predictive maintenance on an episode of our podcast, AI in Industry. Hieu Ho, Predii’s Director of Engineering. holds an MS in Software Engineering from Santa Clara University.
CMMS Systems
GE Asset Performance Management
GE claims its Predix Asset Performance Management software is used to help automotive companies determine what parts may need to be fixed or replaced on machinery like planes, trains, and cars. According to GE, this tool connects with sensors already built into a vehicle and its parts.
After integration, a user can log into the Predix app and see a list of parts in a vehicle that the software flags as showing anomalies. A user can then click into different parts of the list and get information on them, such as their age or how long they have been working improperly.
When a user clicks into a specific machine’s dashboard, they can see information such as its temperature and prioritized alerts. When they click on an alert, they can get specific details. For example, the platform could alert them that a part within the machine is experiencing a higher vibration or temperature than usual.
GE also claims its software allows users to create and assign work orders from a smartphone or tablet.
The 2-minute video below details the software’s dashboard:
GE’s website presents a video case study on the Grove City Remanufacturing Facility, which repairs freight trains that travel throughout the US. The Facility, which began using GE’s software in 2013, is 240,000 square feet and holds over 350 employees which rebuild more than 1200 freight train engines a year, according to GE.
GE’s Predix software was implemented following a mass freight train digitization, requiring all trains passing through the Remanufacturing Facility to be tagged with a barcode and connected to the software.
Today, when a train arrives, GE says the Predix software is first used to analyze sensor data about how the train was running in the past and if certain parts of it are damaged or newer than others. Through text-based alerts, the software can also show a factory employee what types of repairs it will need aside from replacing broken parts.
GE claims that the Remanufacturing Facility is required to have a train fixed within 10 days of arrival. This includes the time needed to analyze the problem, which they claim took more than five days before the digitization. Now, GE says the sensor analysis can take place in real time, which may eliminate employee downtime.
The video case study is provided below:
Predix’s Vice President of Engineering, Jake Johnson, is the former CTO of GE Intelligent Platforms. He has also served as a software engineer at Intel and holds a BSE in Computer Engineering from the University of Michigan.
Emaint
eMaint offers a predictive maintenance and task management software which similarly uses sensor information to determine when an item should be fixed.
The company claims that after connecting machine sensors to the software, an employee that manages machinery can log into the software and see the anomalies the software found in the machine. The software then provides suggestions on when the machine should be looked at or fixed. When a user is looking at a page detailing one specific machine, a user can click to schedule a maintenance appointment or assign a task to an in-house technician to look at or fix the machinery.
The company claims it also offers condition monitoring, which allows a user to click on a part or piece of machinery on a desktop or mobile app and see charts and visualizations showing its rate of “noise, vibration, temperature, lubricants, wear, corrosion, pressure and flow.”
eMaint claims its system can also identify if a new part is needed and connect with the manufacturing company’s part suppliers so a user can purchase or order a new part. The user can then track the part’s delivery directly from the software.
We could not find a full demo of how the software is used or set up.
eMaint claims that Westwater Treatment Plant came to the company with challenges related to machinery compliance, aging equipment, outdated technology and high-energy costs.
Along with sensor data, eMaint built a customized platform with data from the plant’s previous system. While the study did not give specific results, it reports that management at the plant was able to track and lessen the time between a piece of machinery’s logged failure and its repair. The system was also used to order and track new parts when failures were detected.
eMaint was acquired by Fluke for an unspecified amount in September 2016.
Fluke’s CTO, Oliver Sturrock, was formerly the manager of CIO applications at Accenture. That said, we could not find anyone on Fluke’s leadership team with a robust AI background This may be something business leaders in heavy industry may want to consider before working with the company.
Takeaways for Business Leaders in Heavy Industry
It seems that marquis companies like IBM and GE have the most mature products. Similar technology created by smaller companies is of interest to bigger companies, in the case of Progress’ DataRPM acquisition and Fluke’s buyout of eMait.
Whether they provide just asset management or also added maintenance management features, all of the applications claim to leverage already-built sensor data on machinery. All of them also seem to be compatible with at least one brand of smartphone, tablet, or personal computer.
While most companies covered in this report provided demonstrations, case studies and walkthroughs of how the products worked after implementation, none of them provided the steps needed to connect the software to a piece of machinery’s sensors.
Business leaders should take note that it is unclear how much time it might take to integrate one of these applications to a fleet of machinery. Additionally, while some companies provide the cost of their software up front, they do not note if the help they claim to provide clients with integrating their software comes with its purchase.
Header Image Credit: Australian Valuations