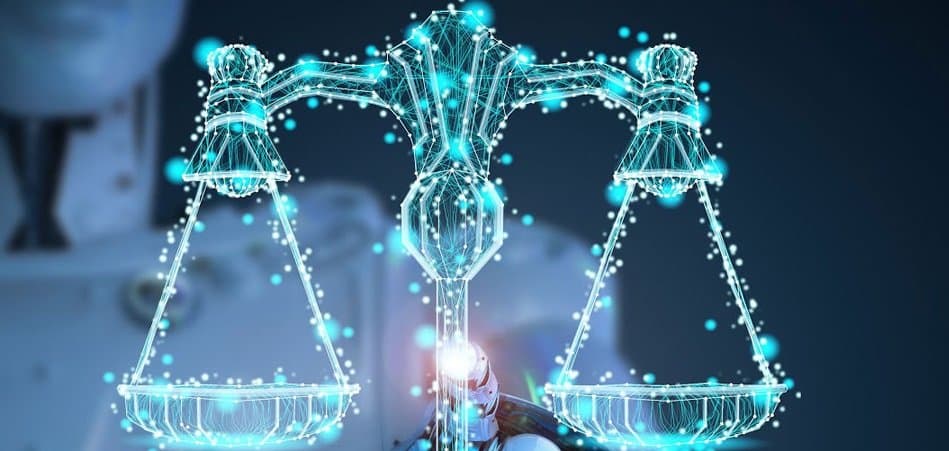
Daniel Faggella, CEO and founder at Emerj, kicked off the Technology Association of Georgia‘s first major event on AI Ethics in May 2019. While he could not be physically present at the event, Daniel addressed the audience through a short video on AI ethics.
In the video below, Daniel talks about transparency and accountability in AI systems and the important conversations AI leaders should carry out when it comes to ethics.
This article captures the essence of Daniel’s talk in the video. Specifically, it addresses the following questions:
- When should an AI system or a process be transparent?
- Who should be accountable for the decision-making processes: human or machine or both?
- Why are both transparency and accountability considered gray areas?
- What are the important questions business leaders should consider before and while incorporating or addressing ethics in AI?
Daniel has addressed the above-mentioned topics in detail in previous articles, such as The Ethics of Artificial Intelligence for Business Leaders – Should Anyone Care? and Managing the Risks of AI – A Planning Guide for Executives.
In this article, we will delve into how business leaders can incorporate transparency and accountability in AI systems according to business phases and levels of risks they entail.
Transparency: How does an AI system arrive at its decision?
Daniel talks about how most organizations view the concept of transparency in AI systems or processes as either black or white, meaning that a certain business process must either be completely transparent or it is considered fishy.
Daniel advises that this perspective is not sustainable. Businesses could look at an AI processor functionality in terms of phases, as opposed to viewing it as a whole.
According to Daniel, each of these phases in the business process may or may not be transparent, depending on certain criteria.
At the high level and in actually bringing AI to life within an enterprise, transparency and accountability are the two ethical considerations that come up most frequently. Often, it’s considered that there’s a business process or a function within a business that either needs to be completely transparent or it can be completely non-transparent. When, in fact, we think that it happens to be more productive to think about businesses in terms of phases.
For example, consider the medical process of diagnosing and treating disease.
The phases of this process could be broken down as:
In order to correctly diagnose the patient’s disease or illness, in many cases, relevant information is needed. The AI system may unearth relevant medical literature to enable the doctor to be equipped with more knowledge and context, in terms of genetic conditions or potential diseases the patient may have.
This phase of the process may or may not need to be transparent because the system is simply enabling the doctor to make a more informed decision, in a systematic manner.
The middle ground situation in this process, Daniel elaborates, might entail computer vision, if we are looking at X-rays or MRI scans using an AI system. For example, depending on the situation, we may or may not want to know how this particular system claims to know for certain that the condition might be a cancer or a fracture.
We may not need transparency here, in Daniel’s opinion; however, if the only alternative is that the doctor had to look at the MRI scans or X-ray reports to arrive at a conclusion based on intuition or knowledge, then this computer vision could be considered as a layer that augments the doctor’s intuition or knowledge. The system is not making a call here.
It is simply calling attention to something that might have been missed out on.
A phase that would warrant for absolute transparency in this process is when the AI system recommends a course of treatment. Daniel says that it is absolutely imperative that we know what factors—genetic, disease-wise, health history—led the machine to recommend a particular course of treatment.
Daniel concludes on transparency in AI as:
So, in this case, in transparency, we’ve thought through the phases of what diagnosis means as opposed to looking at diagnosis as somewhere where we either need transparency or don’t need transparency. Often, the two do end up going together well, if you can figure out what phases of the process require it. So, thinking through business phases is often a way where we can make AI fit its way into a process even if there are some barriers to get there in the first place.
Accountability: Who pulls the trigger? Man or Machine?
According to Daniel, the idea behind accountability can be answered by asking three simple questions:
- Is the human making the decisions?
- Or is the machine making the decisions?
- Ultimately, who is accountable for the decisions made?
The general notion across the top management or the C-suite, Daniel says, is that humans will be in control of a business process “X” and machines will be given control over a business process “Y.” However, the idea of accountability is more complex than that. Dan advises looking at accountability through a lens of risks entailed.
To drive home his point, he takes the example of the process of approving a loan application.
A loan approval process involves a lot of decision making. The process comprises instances where control can be given to humans, as well as instances where control can be placed in the hands of machines.
If we compare both humans and machines trying to detect anomalies in loan applications, then the accountability should lie with the machine. Although it is a complex process, the machine is not making any important decisions in this phase of the process.
The risk level is comparatively low. Humans will have to coax through random data points making it a time consuming and error-prone process.
To strike a middle ground with accountability between humans and machines, Daniel offers the example of a tested and well-trained AI system, which believes that a certain loan application for tens of thousands of dollars is not going to be approved.
In this case, we may or may not require that a human ultimately sign off on every microloan with a very high confidence rate from the machine.
We may only need to inspect, for instance, one out of 50 of such cases where confidence is very high. Instances such as this depend on a variety of business decisions. Daniel explains that:
In terms of risk, the risk of letting that [micro loan applications] go might end up being low enough where we’re ok to let them go.
In certain cases, we can definitively draw a black-and-white line when it comes to making such critical business decisions, according to Daniel. For example, a loan application of more than half-a-million dollars must be signed off by a human.
In this particular case, the levels of risk involved are higher and, therefore, accountability lies with humans.
The accountability in these types of situations can differ throughout a business process based on varying levels of risks that we are operating within.
Daniel says that:
In the enterprise world, at a high level, in terms of applying AI to existing business processes, transparency and accountability are the two big ones [ethical considerations] and thinking through phases and thinking through levels of risk is often easier than black-and-white thinking when it comes to finding a way to find a place where AI can be productive in a business process.
Asking Questions That Matter: Are We Solving Problems or Feigning Virtue?
According to Daniel, AI ethics events such as this TAG event, help business leaders to explicitly focus on solving business problems by holding pragmatic conversations and wide networking. However, in Daniel’s experience, a potential downside at some AI ethics events, is a posturing dynamic that shuts down fruitful conversation around AI ethics.
Daniel further explains that when businesses try to solve a problem, or improve business outcomes, or improve employees’/users’ lives, they have to come up with a lot of ideas.
We have to generate many ideas around where AI might find a home to improve our efficiencies, optimize our processes. And something that shuts that down is a particular kind of posturing when it comes to AI ethics.
The hard part about AI ethics is asking how can we navigate from where we are, to finding where AI can apply itself where we’re not breaking the law, we’re not breaking our own values, and we are driving value for the company and for our customers.
That’s hard. That’s very hard work. What’s not hard work is to act indignantly at any kind of AI idea that could be in some way interpreted to be immoral.
So there’s there’s two kinds of ethical insertions into conversation, sort of clashes, that can come up and we’re talking about ethics: one could be frankly addressing an ethical concern so that we can navigate through it, so that we can figure out how to move around it, so that we can figure out how to achieve that outcome improving business outcomes, improving customers lives, the hard work of really thinking through ethical problems. The other reason to bring up an ethical problem is simply to state frankly how moral we are, how concerned for others we are.
Daniel segregates the questions business leaders should ask themselves and types of conversations they should be having when it comes to AI ethics in two:
The safest way to do that is not to solve a problem but simply, to frankly, bring up a concern and ring an alarm in a way that maybe is even accusatory of those who are bold enough, selfish enough to think of that AI idea in the first place. And this is the enemy of coming up with good business ideas.
According to Daniel, business leaders in AI ethics events must bring up ethical concerns in order to work around them and discuss and find a way to achieve efficient and worthwhile outcomes. Dan advises business leaders to wary of parlor soldiers:
We have to brainstorm. We have to share ideas. So, if you see that kind of what Emerson would call parlor soldiers, that sort of preening of morality without actually stepping into the ring, and making a firm judgement call about how this could improve a business process or improve lives, be wary of that.
Header image credit: SVFG