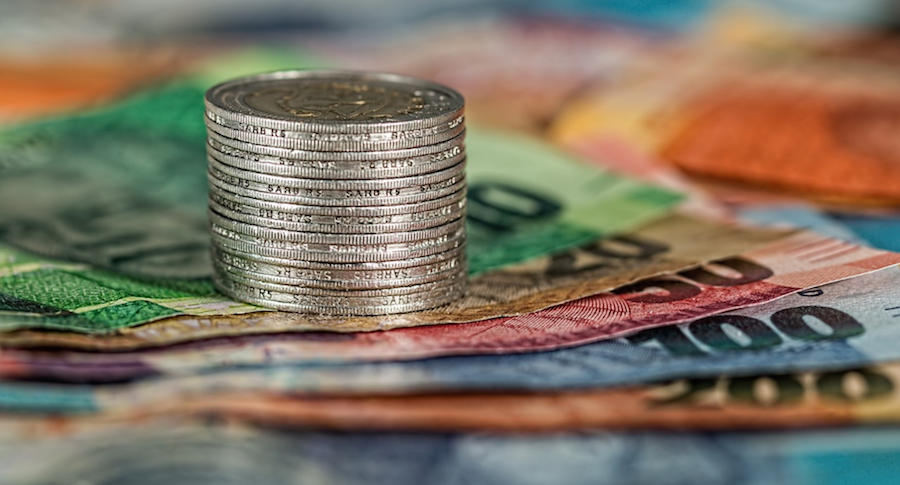
In a 2017 symposium in Harvard’s Institute for Applied Computational Science, R. Martin Chavez, Deputy Chief Financial Officer of Goldman Sachs explains that the company’s US cash equities trading division used to employ over 600 human traders back in 2000. Today that number is down to just two human traders, with the rest of the jobs being taken over by automated trading platforms that are managed by around 200 computer engineers.
Automation and AI already have several applications in banking and finance, and over the last few years, the fintech sector has been witnessing a spate of new artificial intelligence applications due to the availability of new data sources, and the proliferation artificial intelligence tools and techniques.
From our research, we’ve determined that the possibility-space for what AI can do for investment banking is somewhat nascent. Although there have been inroads for this technology in the banking industry, they have mostly been transferrable use-cases from other branches of finance.
In this article, we’ll discuss five current AI applications in investment banking. We’ve separated the researched applications noted in this article into three categories:
- AI for Trade Processing
- How Predictive Analytics Vendors can help Investment Banks
- Automating Market Data Collection for Investment Firms
With this research, we hope to give members of the industry a look at the near-term applications of AI, as well as take-aways that may assist in a company or banks future AI-related decisions.
AI for Trade Processing
Man AHL by Man Group
Man AHL was founded in 1987 in London and currently has over 330 employees. The company claims to have built a platform that can potentially identify systematic investment strategies and automatically execute trades over several global financial markets.
Man AHL offers quantitative investment management for which the company claims to be using machine learning to optimize trade execution from different channels. We explain in further detail below:
- When traders place a buy/sell order, electronic routing algorithms are used to find a match from stock brokers, stock exchanges or alternative trading systems that can fulfill the order. In many cases, this electronic routing of the trade by brokers may potentially limit liquidity and increase costs for the trader.
- Man AHL claims to be using machine learning to optimize the routing of trades generated by their platform from three possible execution channels – internal execution algorithms, external dealer algorithms or the firm’s trading desk.
- According to CEO Matthew Sargaison the machine learning algorithm directs trades through one of the three channels, based on past experience (Ex: historical data of how much commissions were paid through each of the execution channels) calculates the probability of the outcomes (in terms of cost and liquidity) for each execution route
For more information on how AI can facilitate wealth management and other aspects of trade and finance, download the Executive Brief for our AI in Banking Vendor Scorecard and Capability Map report.
According to Man, their platform can be integrated with existing trading systems and can automatically route trades that an investment firm’s traders are executing. Whenever a trade is executed either by the internal algorithms, external dealer algorithms or from a trading desk, the order is first sent to the AI platform which routes the trade in the most efficient and cost optimized manner by breaking the order into smaller trades in many cases.
In addition to automating the individual steps within a given trade, AI applications can also serve to help trading customers complete tasks within a user interface. We spoke to Ohad Samet, Co-founder and CEO of TrueAccord, about the ways in which machine learning can facilitate debt collection. In the interview, Samet emphasizes the importance of highly personalized user experiences as AI and other technologies begin to transform banking. When asked how these experiences can be personalized, Samet said:
“We see in [collections] where treating consumers the way they want, through the channels they want, through mobile access [or] email-first communication, allows us to collect better and have much higher NPS and so on. Having an experience that you [the consumer] want, choosing the product you want, engaging the way and at the time that you want, and having everything on demand just increases engagement and affinity to a brand. And for banks their brand and the products they can actually sell to their consumers is their lifeblood.”
It is apparent that Samet thinks facilitating user engagement with both a bank’s brand and their commerce channels is of great importance when considering how to better collect funds from customers. AI applications can further these types of developments by automating the transactional steps that tend to cause the most friction for customers.
Man Group collaborated with The Oxford University to create the Oxford-Man Institute (OMI) with Professor Stephen Roberts as the Director of OMI, Royal Academy of Engineering and Man Group Research Chair in Machine Learning.
The 6-minute video given below from Man AHL explains how their fund manager uses AI in quantitative finance:
From out preliminary research we see that Man AHLs fund performance has had mixed results since its inception in 2010 although there seems to be a definite positive performance trend over the last 3 years (apart from a slight slump in 2017. Although we could not find much information about how much money the company might have saved by using machine learning for routing trades.
Smart Chaser from BNP Paribas Securities Services
BNP Paribas is a leading bank in Europe that was founded in 1848 and is headquartered in Paris. BNP Paribas Securities Services (which provides treasury finance and advisory services for investment managers and firms) announced the implementation of a trade matching tool called Smart Chaser which uses predictive analysis to automate trade processing services.
Thomas Durif, Global Head of Middle Office Products at BNP Paribas Securities Services, said:
“We estimate that up to 30% of the trades processed on behalf of asset managers require manual intervention in order to complete. This is an industry-wide challenge which is often caused by counterparties holding mismatching data for the same trade.”
- Trade-matching is a key part of the trading process for investment managers where trade details between the client and its broker are compared with each other. If matching is not performed at the right time, the trade may require human intervention or in some cases may fail.
- BNP’s Smart Chaser used historical data records of trades made through BNP Securities Services, to identify patterns in trades that lead to a trade failure or manual intervention being needed. For example, like identifying that human intervention or failure is more likely if a trade is not settled on the day of execution.
- BNP claims that Smart Chaser can then predict the likelihood of a particular trade needing manual intervention and will prompt investment managers through a dashboard to take action if the predicted likelihood is particularly high. The company claims that to have achieved around 98-percent prediction accuracy (which might have to be taken with a grain of salt since there is no evidence presented at all for this claim).
Investment managers can use the Smart Chaser software while trading live to receive actionable insights on avoiding trades that might require manual intervention. BNP claims their software tool can monitor trades executed by investment managers or their clients in real-time and prompt users with a notification when a trade with a higher risk of manual intervention is detected and suggests a list of alternative trading channels for faster executions.
We could not find any demo video from BNP showing evidence of how the Smart Chaser platform uses machine learning to assist investment managers with trade matching. However, it seems that a user, such as an investor or advisor, could log into the platform and see trade match suggestions based on predictive data.
How Predictive Analytics Vendors can help Investment Banks
Katana from ING
Dutch banking services provider ING was founded in Amsterdam in 1991. In December 2017, the Dutch bank ING launched a new tool called Katana, purportedly designed to help bond-traders make better and faster pricing decisions. According to ING:
“Katana uses predictive analytics to help traders decide what price to quote when buying and selling bonds for their clients, based on historic and real-time data.”
Katana was developed by ING’s Financial Markets Global Credit Trading team in London. ING believes that the platform can learn from historical and real-time trading data and predict a statistical forecast which traders can use in addition to their natural intuition.
The ING infographic below depicts some of the major features which ING highlights within in the Katana interface (interested readers can see the full infographic here):
Image from ING
ING claims that pilot testing of their Katana platform for traders in the emerging markets (EM) desk in London showed that they were able to quote prices faster for 90% of trades, reduce the cost of trading and traders quoted the best possible price more frequently. At the time of this report, there was no information on what the time frame was for this pilot or what were the original times that traders took to arrive at quotes.
ING has not disclosed clients that have used this specific program.
Kortical
Kortical was founded in 2016 in London and currently has around 7 employees. The company offers an AI platform called The Kore, which the company claims can help businesses with predictive analytics.
When analysts and data researchers log into The Kore platform, they can click “Create a Project for Sales Forecasting.” Once the project is created, a user can upload data in an Excel sheet directly from their computer. From there, data analysts can add “parameters” to organize and clean the data. The program then directs the analyst, showing them how to produce what it claims is the most appropriate machine learning model needed to analyze their data. The analyst can then use other training tools to further teach machine learning algorithms to predict future outcomes, according to Kortical.
We could find no specific instances of successful case studies apart from those mentioned on Kortical’s website. We highlight one case-study where the company claims to have aided an investment bank with speeding up their trading risk-prediction process.
- The investment bank needed to calculate the predicted ROI on almost 5 million trades each night which was being done through 80,000 computers. The bank was using human analysts to help classify the trades in ways that might help make this process faster but was constrained by the sheer number of trades.
- Kortical claims they used The Kore to create a predictive model that could predict how long it might take to calculate the ROI for each trade using historical data recorded by the human analysts from the investment firm. (For example, they could be identifying patterns such as trading done through the investment firms trading desks seeming to take longer to predict an ROI for.)
- Kortical claims that the investment bank had an improvement of 30 percent in the overall time taken for the risk prediction process after a period of three days with the platform continuing adjust the model for adapting to further changes.
Although there was no information on what other measures the investment bank took during the same time to speed up risk prediction, or what the original time required for the process was using human analysts. There was also no reference of how long it took to reach the study’s end result after implementation.
Kortical CEO Alex Allan has previously earned a Bachelor’s degree in Cybernetics and Artificial Intelligence and a PhD in Data Science from the University of Reading. He also served as the Chief Data Officer for the London-based startup Datamind. We could find no evidence of Kortical having worked with marquee companies in AI realted projects.
Automating Market Data Collection for Investment Firms
Sigmoidal
Sigmoidal was founded in New York in 2016 and has around 18 employees today. The company offers consulting services for businesses looking to leverage AI in the finance, construction, military, and technology spaces.
Sigmoidal helps businesses by providing consultation, software development, development operations (DevOps) and data tagging services for AI integration. For example, the company claims that they can help investment firms optimize the process of obtaining market information to identify investment opportunities using AI algorithms (like document classification or named entity recognition). This might enable the investment firm to detect certain changes in the market developments without any human intervention. We explain in further detail below:
- An investment management firm looking for information regarding changes to the management structure of firms represented on the New York Stock Exchange ( NYSE) might traditionally accomplish this task through human research analysts who scour through business news pages and social media to collect a list of organizational changes in NYSE companies.
- AI platforms like Sigmoidal can potentially help automate the information-collection using ‘web scrapers’ to first aggregate data from the different sources. The platform then uses AI text classification algorithms to filter out the information that is most relevant to the investor’s needs. (See Figure 1 below – source)
- Sigmoidal claims that their platform also uses AI for named entity extraction where details like the company name, position can be identified from text in the data collected. (See Figure 2 below)
The two figures from the company below give a look at what features of this platform looks like for its users.
Figure 1:
Figure 2:
Images from Sigmoidal
Sigmoidal also claims to have developed a trading platform that uses machine learning to potentially track a user’s account activity and understand the individual patterns in how the owner of the account might spend, invest, and make financial decisions; The platform can then use this data to create personalized investment advice to users. Although we could find no evidence of any robust case-studies or instances of their platform being used for trading applications.
The company also claims to have used a Long Short-Term Memory recurrent neural network (LSTM) which could potentially improve predictions for the expected returns of assets in the fund. Additionally, the platform was reportedly also able to estimate an asset’s risk level, although at the time of writing there was no evidence of what results were obtained purely due to the platform’s features.
Although the company claims to have worked in projects with NASA, DARPA, NVIDIA, Microsoft, PwC, Goldman Sachs, and Intel we couldn’t find much evidence of said collaborations. Sigmoidal’s current Head of Artificial Intelligence Marek Bardoński has earned a bachelor’s degree in Computer Science from the University of Warsaw, Poland and has previously served as the Senior Deep Learning Research Engineer at NVIDIA.
Predictions and Suggestions
Near-Term Impact of AI in Investment Banking
Investment banking seems to be ripe for AI applications due to the existence of historical trading records going back many years. In the near term we expect the following trends to impact investment banks:
- AI might help automate and optimize tasks like trade processing and routing in most investment banks since at larger scales, these are problems that become impractical for human traders and investment managers to tackle.
- AI can potentially help investment banks predict risks in trading much faster than human analysts and might also be ubiquitously applied to automating data collection (like market developments from the web) in the next five years.
- Using AI to analyze market development and identify systematic trading strategies are already being used in exchange traded funds (ETFs) and many AI platforms might emerge in the near future that are tailored towards assisting traders and investors with choosing the best strategy.
Take-Away for Business Leaders in Investment Banking
- Investment banks need to be mindful of the fact that although they may have access to historical trading data, this data might be useless unless properly ‘cleaned’ and ‘tagged’ so that it can be used to train any AI models.
- There have been instances of AI vendors offering consulting services for investment banks (Example Sigmoidal). Banks with little previous experience in AI integrations might do well to understand the scope of services that vendors provide before embarking on any project.
Header image credit: Pixabay