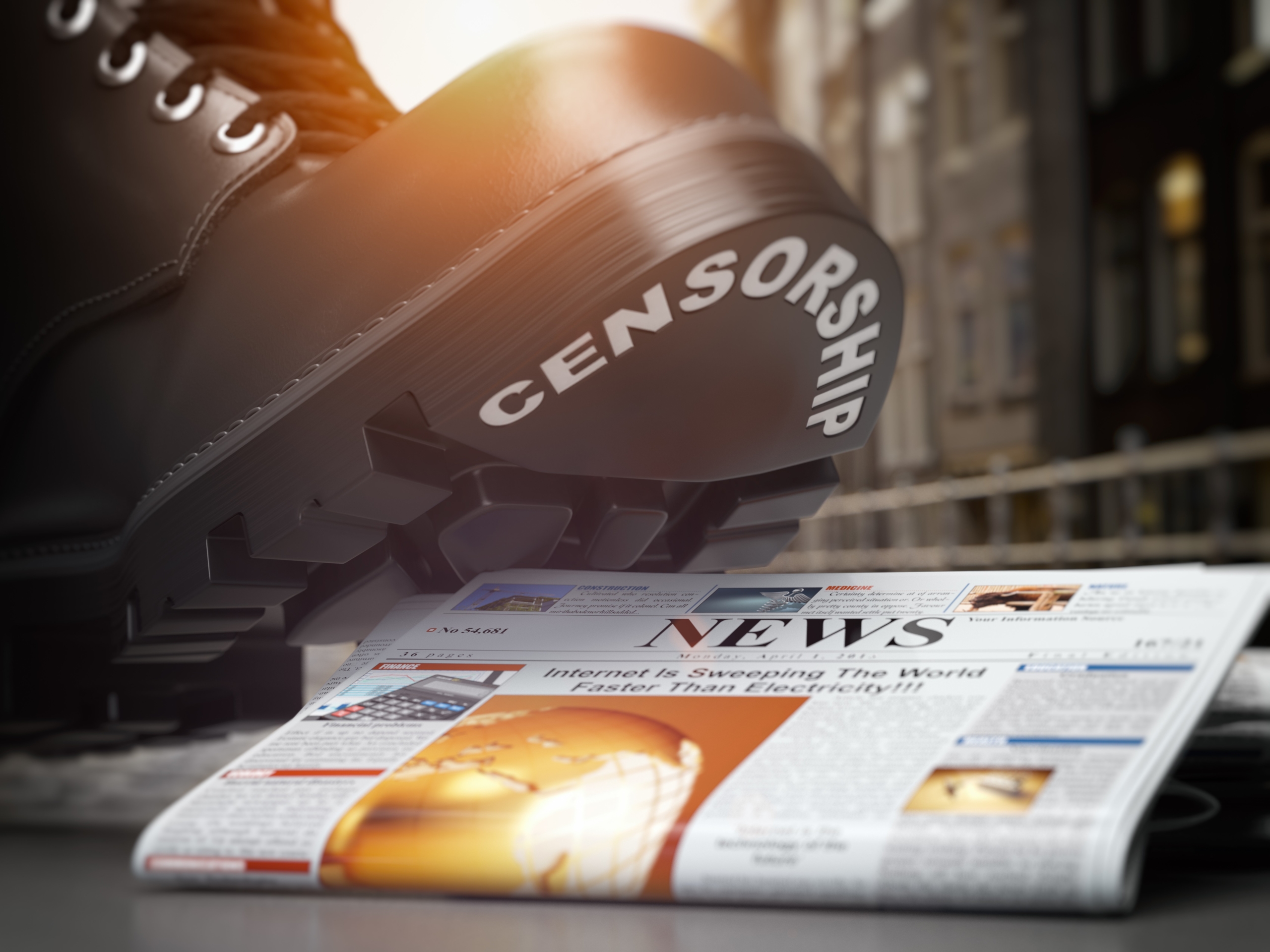
A 2017 report by the Pew Research Center found that 69 percent of the American public use some type of social media, like Facebook, Twitter, Instagram, and others.
According to Hootsuite’s 2018 Social Media Trends Report, social media brands will continue to use AI strategies to “prioritize personalization at scale.” This will involve using AI to leverage social data as a source of customer insights.
With the recent allegations of Russian interference in the American presidential elections and the ouster of Cambridge Analytica, British political consulting firm in the saga, social media censorship and data privacy have become de facto points of discussion in a global context.
This article presents real-world applications of AI for social media platforms like Facebook, Twitter and YouTube. We explore what is possible today with AI in social media applications and how social media platforms are using AI in dynamic censorship applications. We also look at what transferable lessons business executives can learn from these social media giants.
The company is using AI to bring suicide prevention to Live and Messenger. Aside from its official page guiding users on what to do when someone posts about suicide or self-injury, the company launched another AI-based tools (which function through integration with Facebook posts and Facebook Live) in 2015 with the help of non-profit organizations advocating suicide prevention and mental health.
According to the University of Washington’s School of Social Work (which Facebook has also worked with to develop the tool), whenever users find someone posting something troubling on the social media site, they can report it to Facebook. The company then reviews the post and offer options for the person in distress such as videos featuring real-life accounts of people who have recovered from their struggles.
The user who reported the post will also be given the option to contact the friend in distress, contact another friend for additional support, or call a suicide helpline. Aside from offering recommendations on diverting their attention to productive activities such as art, reading and cooking, Facebook will also find a self-care advisor for the person when needed.
According to Vanessa Callison-Burch, Product Manager, Facebook, the AI tool was configured using data from anonymous historical facebook posts and facebook live videos with an underlying layer of pattern recognition to predict when some may be expressing thoughts of suicide or self harm. when the system identifies a post or Facebook Live broadcast as ‘red flagged’ by using a predefined trigger value for the prediction output, those posts are routed to Facebook’s In-house reviewers who, they make the final decision of contacting first responders.
The 1 minute video below shows how real Facebook posts are read aloud by the AI system, describing the content of individual images used in posts:
Facebook claims that their proactive detection tools on average results in around 100 instances where Facebook alerted local first responders in Austin, Texas over the course of a month currently.
Facebook has also recently opened an AI research center in Montreal, Canada as a part of their As part of Facebook AI Research (FAIR). the lab will be led by Professor Joelle Pineau, who’s previous work includes developing new algorithms for planning and learning in robotics, healthcare, games, and conversational agents like chatbots.
According to Yann LeCun, Director of AI, “Human children are quick at learning human dialogue and learning common sense about the world. We think there is something we haven’t discovered yet—some learning paradigm that we haven’t figured out. I personally think being able to crack this nut is one of the main obstacles to making real progress in AI.”
For example, Facebook has tapped the blind or visually impaired audiences’ community to experience the social media platform through automatic alternative text. This technology works by allowing anyone to swipe on Facebook photos and use screen readers on iOS devices to hear a list of the items that an image contains.
According to Facebook’s press release, “This is possible because of Facebook’s object recognition technology, based on a neural network that has billions of parameters and is trained with millions of examples. Each advancement in object recognition technology means that the Facebook Accessibility team will be able to make technology even more accessible for more people.”
Here’s a short video demonstration of Facebook’s object recognition technology being used as a screen reader to enable persons with sight deficiencies to listen to descriptions of images:
For more examples of Facebook’s AI tools, you can read our previous article. Our interview with Facebook’s Hussein Mehanna, Director of Engineering of the core Machine Learning group, provides insights on how the tech giant is working to overcome tech barriers to implement personalization.
YouTube
Recommendation systems provide a way to suggest similar products (such as in Amazon), news articles (Huffington Post) and TV shows (Netflix) to users. In the case of YouTube, Paul Covington and his team of data mining experts at Google describe the world’s largest video-sharing website as one of “the largest scale and most sophisticated industrial recommendation systems in existence.”
The system is powered by Google Brain, which relies on deep learning AI. It has one neural network for gathering user information (such as watch history and user feedback) and another neural network for ranking the selected videos that are displayed.
Jim McFadden, Technical Lead for Recommendations, describes the solutions that Google Brain has brought to his team: “One of the key things it does is its able to generalize. Whereas before, if I watch this video from a comedian, our recommendations were pretty good at saying, here’s another one just like it. But the Google Brain model figures out other comedians who are similar but not exactly the same — even more adjacent relationships. It’s able to see patterns that are less obvious,” he says.
YouTube has also recently taken the initiative to combat videos with ‘terrorism related’ content which do not comply with the terms posted on its website. In a June 2017 blog post by Google’s General Counsel, Kent Walker, the company claimed they have thousands of users and engineers who review and prevent uploading of terrorism-related content. Concurrently, it introduced a machine learning tool that flags violent extremism videos and reports it to its reviewers for verification.
The website claims since its implementation, the team has reviewed more than a million videos to provide data to train the system’s flagging capabilities. The AI was able to take down 83 percent of violent extremist videos in September 2017 before its team has reviewed each upload.
Using historical data available from their team that addresses controversial content, and through human-guided expert assistance, YouTube claims that they were able to leverage machine learning to automate the initial red flagging of the content. In their blog post, they also add that, although the platform is still not perfect (it has achieved human-level accuracy or better in certain settings)
This comes after YouTube faced a huge threat from advertisers in early 2017 when the UK government and ad companies such as Havas found out that their advertisements were placed next to extremist-related videos. Some brands that pulled their ads from YouTube included Walmart, Johnson & Johnson and Pepsi. In response, YouTube said on their blog page, that they will continue to develop the accuracy of the technology while simultaneously hiring more humans to help review and enforce policies.
In May 2017, Twitter’s stocks were on a rally after Mark Cuban bought shares because the company announced it was working on AI. But news of the company exploring AI came to prominence in 2014 when it acquired Madbits, a computer vision startup in New York. The acquisition was probably made to improve Twitter’s image features leveraging on Madbits’ image search technology, according to TechCrunch, No comment was heard from Twitter since then.
In 2015, it acquired 15-month old startup Whetlab, which was developed by Harvard computer scientists. According to the Harvard School of Engineering and Applied Sciences, the five-man team created Whetlab for software engineers on visual object recognition, speech processing, or computational biology. The acquisition by Twitter, however, is viewed by Sephi Shapira, CEO of advertising company MassiveImpact, as a way to “obtain the team’s talent and to apply more AI tools to advertising to build a competitive advantage against Facebook and Google.”
A year after, it acquired AI startup Magic Pony Technology for $150 million. According to Twitter cofounder, Jack Dorsey, the acquisition will focus on developing image and video capabilities. “Magic Pony’s technology—based on research by the team to create algorithms that can understand the features of imagery—will be used to enhance our strength in live and video and opens up a lot of exciting creative possibilities for Twitter.”
After three years of acquiring AI startups, Twitter announced on a blog post in May 2017 through its software engineer Nicolas Koumchatzky, that the company is using an AI platform developed by its in-house AI engineers to rank tweets based on how interesting it will be to audiences instead of the old display using reverse chronological order.
Tweets are ranked based on numerous factors like the tweet’s recentness, the number of times it has been re-tweeted, and a user’s connection with the tweeter. “This opens the door for us to use more of the many novelties that the deep learning community has to offer, especially in the areas of NLP (Natural Language Processing), conversation understanding, and media domains,” Koumchatzky wrote.
Finally, the company announced its plans to combat hate speech in its blog. It has collaborated with IBM in March 2017 to use its AI platform, the IBM Watson, to control controversial hate speech on the website. Twitter Vice President for data strategy, Chris Moody, explains in an interview, “Watson is really good at understanding nuances in language and intention. What we want to do is be able to identify abuse patterns early and stop this behavior before it starts.”
For example, Watson could flag an account that is repeatedly tweeting at non-followers or engaging in hate speech that is in violation of the Twitter Rules. The human-review team at twitter would then take the final action on the account. As with the other social media firms, Twitter’s content review team’s input is expected to increase the accuracy of the machine learning platform over time.
The company, by using IBM Watson to identify and flag hate speech, will impose measures to control offenders by showing their tweets only to their followers or by blocking them from the site. This partnership comes on the heels of the global partnership deal signed between the two companies in 2014.
Concluding Thoughts
Interested readers can see this two and a half hour testimonial from executives of Facebook, Twitter and YouTube discussed what each of their companies was doing to combat extremism and terror-related content from their social media platforms in a senate hearing of the U.S. Parliament.
In terms of approach towards social media censorship, it seems as though most of the large social media platforms are following a similar curve of AI adoption. Human-guided AI training seems to be a common theme here and the fact that content review was already a key business function in most of these companies is a key advantage in terms of configuring the ML platforms to function more accurately, faster.
The commonalities also seems to stretch to the performance of these AI platforms in social media censorship applications in that they are still a work in progress. Although there have been setbacks (for example like with YouTube) and the application has proved to have a steep learning curve, there is a sense of coming progress towards making these systems far more efficient in the future.
Header image credit: Adobe Stock