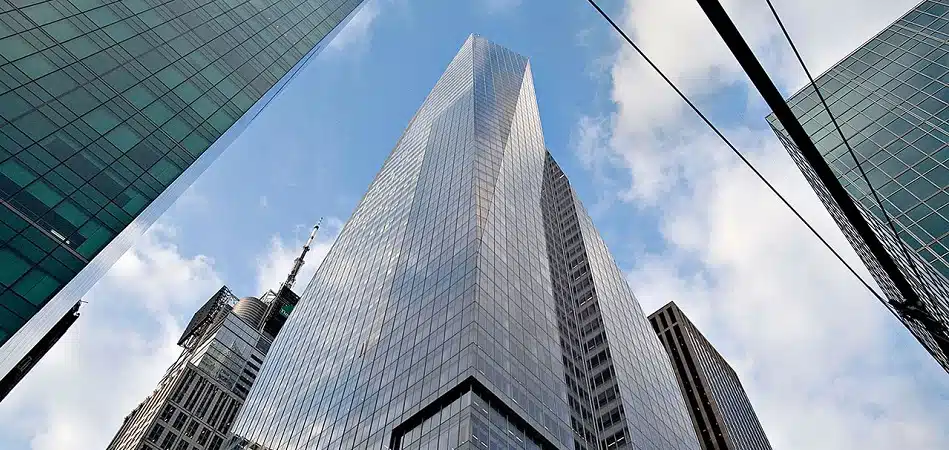
Business process management (BPM) in banking involves the automation of operations management by identifying, modeling, analyzing, and improving business processes. Many banks already have some form of BPM for various process. For example, compliance processes at most banks tend to have some form of software automation in their workflows.
This is because there is a lot of pressure in banking functions such as compliance, fraud, and risk management to develop processes that are more cost-effective. This can be very challenging for human teams to do alone.
Business process management is one way for banks to improve the efficiency of internal workflows. Simple rules-based software automation needs to be updated every time there is even a small change in the process. Any alteration in the rules means additional cost and team resources. AI techniques might help BPM software accomplish tasks better over time, which allows human employees to focus their time on more complex tasks.
Adoption of AI for BPM is still Cautious in Banking
Harvard Business Review claims to have surveyed thousands of business executives asking about how AI is being applied in their organization. The survey found that only about 8% of businesses were applying AI in their core business processes.
The results indicated that most firms have engaged in one-off pilot projects or are applying AI in an application involving only a single business process.
One of the reasons for the slower than expected adoption of AI in this space is likely because banks have not taken enough steps to reorganize their processes in a way that makes them more conducive to AI.
Currently, most banks might have data and processes for each of their business units as siloed entities. Creating and deploying BPM solutions that are cross-functional in such cases is challenging because of data formatting, handling, and access issues.
Banking leaders need to evolve in terms of their approach to risk in order to fully capitalize on any potential AI opportunities. Traditionally bankers have been risk-averse and most AI projects come with huge initial costs without much by way of clear evidence of returns.
BPM projects might also mean that existing workflows in a business could be disrupted temporarily. Any large scale AI-based BPM project in banks will require investment in data infrastructure and restructuring of data flows in the organization.
Current Use-Cases for AI-based BPM Solutions
AI for Automating Sales Analysis
Traditionally, sales analysis has been a manual process in which human employees are required to listen to customer interactions to identify how to improve brand image, upsell, acquire new customers, and strengthen customer loyalty.
A bank could use an AI-based BPM solution to automatically record, transcribe and extract the most important parts of these conversations.
The AI software could be trained to “recognize” customer sentiments by automatically categorizing the topics that customers seemed to care about the most or assess the performance of sales representatives by analyzing the tone and content of conversations. Manually doing these tasks involves listening to countless hours of conversations.
These types of AI software use natural language processing techniques to learn from historical records about what the next best action ]might be for a sales representative with regards to dealing with a potential customer.
Such software could then help sales representative by prompting them with a list of possible next steps, such as calling the customer at a certain date and time with a specific message or sending a potential customer an email with product information as soon as they make a request for information on any channel of communication.
AI for Automating Contract Management
At banks, legal teams need to read through several thousands of documents such as NDAs, logistical agreements, or compliance documents in order to identify issues for the bank that may lead to losses.
There is some evidence to support the fact that AI-based contract management can perform at an accuracy level similar to that of teams of lawyers at a much faster rate and at scale. For instance, banks can develop and train AI algorithms to identify specific issues in non-disclosure agreements.
This process would involve inputs from data scientists, legal and machine learning experts. The software is usually input with existing historical contracts that have been tagged and labeled by legal subject matter experts.
These legal experts would need to identify each specific type of contractual issue and “teach” the software to recognize these issues using several historical contracts. When the software is deployed and allowed to read through new contracts, it can automatically identify if the new contract has any of the issues pointed out by the legal experts in the past. The software can do this by associating certain chains of text with each issue.
The advantage of using the AI software for contract management lies in reducing the time spent in this task. While a human lawyer might be capable of achieving a contract review in the order of hours, AI software can potentially cut this down to the order of seconds, without having the accuracy of identification drop.
AI-based contract management tools can also be tailored to specific legal use cases, giving them added context to analyze documents. For instance, if a similar AI software were to be used to identify contractual issues in logistical documents, the software would need inputs from both legal and supply chain experts at the bank.
Vendor Profile: Blue Prism
Blue Prism offers an RPA platform which it claims can help banks and financial institutions automate business processes. The company also claims their RPA platform comes with added AI features, such as the ability to integrate computer vision or NLP into business process automation projects.
AI software brings with it the advantage of improving operational efficiency continuously over time so that given the right kind of data and right kind of algorithmic tweaking, the software can help banks completely automate tasks such as automated document digitization or automated report generation.
Below is a short 2-minute video demonstrating how Blue Prism RPA Platform collaborated with Google to incorporate machine learning capabilities in a solution:
Blue Prism claims to have worked with The Co-operative Banking Group to automate 10 internal business processes. The bank reportedly deployed the Blue Prism platform to automate 10 business processes including direct debit cancellation, account closures, CHAPS payments, foreign payments, audit reports, internet applications, and Card and Pin Pulls.
These operations were taking a lot of time and manual efforts at the bank making them expensive processes to run. The RPA software was deployed for each of these processes individually to automate internal workflows such as comparing names and addresses of customers who have opted for account closures with the bank’s internal.
According to Blue Prism, this resulted in time and cost benefits in all the business processes within 12 months of the integration.
Adoption Best-Practices for AI-Based BPM Solutions
A critical idea for business leaders in banking to understand before getting into AI-based process automation is that they need to change their mindset about how to look at technology opportunities. Many AI projects are going to be deployed before they are returning a desired result 100% of the time.
AI projects involve a lot of algorithmic tweaking, and achieving a state of high accuracy may take many months. This is important because banks cannot jump into AI projects in their core business processes and expect smooth functions across the board immediately.
Any such project will certainly result in that particular business process being temporarily hindered or forces into a downtime until the AI project is completed. Thus bankers need to develop a try-test-learn-improve mentality while considering AI in order to gain confidence in data science projects.
These BPM projects are going to impact business workflows if they are large scale and being deployed across the organization. Banks might be better off focusing on setting up their data infrastructure and moving away from data silos. Once they have the required infrastructure and skills, banks can identify areas within their existing business where AI can effectively add value.
Banks can also expect to only have a rough solution in the first iteration which they need to improve by incorporating user feedback to correct minor issues. This will also allow small AI teams at banks to develop and deploy projects much faster while still not hindering existing workflows.
These changes in mindset are very fundamental shifts from the way banking leaders have traditionally approached technology. This is probably not something that banks can change overnight and requires careful preparation, planning, and a strategy to help the workforces have a data-centric approach to business processes.
Such fundamental shifts don’t come easily. They require leaders to prepare, motivate, and equip the workforce to make a change. But leaders must first be prepared themselves before inculcating this approach in their organizations.
From our interviews with AI experts, we believe that BPM applications of AI are challenging to do at scale, but banks that successfully manage to do it will have a dominant advantage in terms of operational efficiency.
Header Image Credit: The Economist