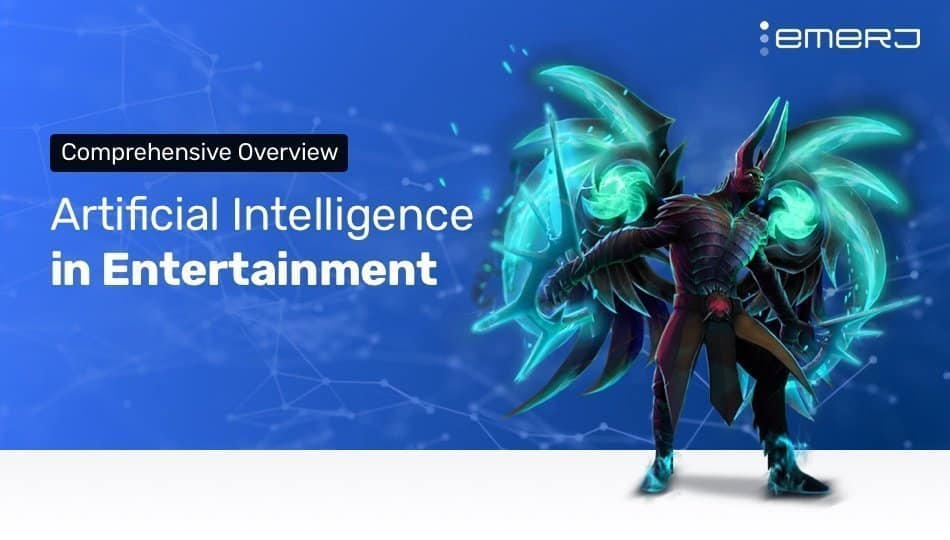
The entertainment and media (E&M) industry is a diverse sector composed of multiple segments including film, television and media streamed online. By 2021, the U.S. E&M industry is projected to reach $759 billion in revenue, increasing at a compound annual growth rate (CAGR) of 3.6 percent.
Despite the anticipated growth, there are concerns about a revenue declines in more traditional market segments. As a result, industry analysts such as PwC argue that user experience must take increasing priority and AI is among leading emerging technologies poised to positively contribute to this effort.
To gauge the emerging role of AI in the E&M industry, we researched this sector in depth to help answer questions business leaders are asking today, including:
- What types of AI applications are currently in use in the entertainment and media industry?
- How has the market responded to these AI applications?
- Are there any common trends among these innovation efforts – and how could these trends possibly affect the future of the entertainment and media sector?
In this article we break down applications of artificial intelligence in the entertainment and media industry market to provide business leaders with an understanding of current and emerging trends that may impact their sector. We’ll begin with a synopsis of the sectors we covered:
Entertainment and Media AI Applications Overview
Based on our assessment of the applications in this sector, the majority of entertainment and media use-cases appear to fall into three major categories:
- Marketing and Advertising: Companies are training machine learning algorithms to help develop film trailers and design advertisements.
- Personalization of User Experience: Entertainment providers are using machine learning to recommend personalized content based on data from user activity and behavior.
- Search Optimization: Media content producers are using AI software to improve the speed and efficiency of the media production process and the ability to organize visual assets.
In the full article below, we’ll explore the AI applications of each application by section and provide representative examples.
Marketing and Advertising
Fox and IBM Watson – Morgan Film Trailer
In August 2016, IBM announced the release of the trailer for a 20th Century Fox suspense/horror film Morgan reportedly developed using machine learning. The research team trained the AI system on scenes from “100 horror movies.” Features from each of the movie scenes were categorized into what the team called “moments” and were then analyzed based on visual, audio and scene composition elements.
Once the system gained an understanding of the types of scenes found in a standard suspense/horror movie trailer, it was given the full-length film and recommended 10 moments for the Morgan trailer. A total of six minutes of footage were pulled from the 90-minute movie, resulting in a 24 hour process, from start to finish. Comparatively, the film trailer development process normally takes weeks to complete. See the complete film trailer below:
While the impact of integrating AI may have resulted in savings in trailer production costs, the estimated $8 million budget film grossed just over $7.3 million in global box office sales. However, with Morgan being the first attempt at using AI for trailer development, it is too soon to accurately determine the direct impact on ticket sales.
This application is similar to IBM Watson’s foray into sports – where the technology was used to generate a highlight reel from tennis matches by analyzing video footage and fan reactions in real time from multiple angles (we covered this other Watson use-case in our full AI in Sports article).
McCann Erickson Japan – “AI Creative Director”
In March 2016, advertising agency MaCann Erickson Japan reportedly launched an AI creative director called AI-CD ß. The company claims this is the first robotic creative director developed using artificial intelligence. AI-CD ß was officially hired on April 1, 2016 along with 11 other human employees.
The machine learning algorithm driving the AI employee was trained on data including specific elements of TV shows and about a decade’s worth of detailed information on the winners of All Japan Radio & Television Commersion Confederation’s CM Festival. Through data mining, the system can extract ideas and themes that would suit a particular client’s ad campaign.
In September 2016, AI-CD ß was pitted against McCann Erickson Japan creative director Mitsuru Kuramoto, in a friendly duel. Tasked with developing a spot for a Japanese mint brant, promoting “instant, long-lasting refreshment that lasts for 10 minutes,” each entry was submitted for judging by a nationwide poll.
The poll results reportedly showed that Kuramoto won the majority of the vote coming in at 54 percent while AI-CD ß earned the remaining 46 percent. While this isn’t the ideal outcome for the AI creative director, the narrow margin shows promise for the robot’s future efforts.
However, it is important to acknowledge the inherent limitations of AI in generating original ideas without human assistance. Tony McCaffey, PhD a cognitive psychologist and computer scientist by training, conducted research reportedly demonstrating mathematical proof that computers are limited in their ability to perform creative tasks. He is among analysts who argue that while repetitive tasks can be more efficiently handled using AI, when it comes to creativity, human and computer collaboration is most effective.
Personalization of User Experience
Netflix – Machine Learning Workflow Management
When it comes to on-demand entertainment, personalization of the user experience has shifted from a luxury to a user expectation. For example, according to its 2016 annual report Netflix boasts 93 million global members streaming over 125 million hours of TV shows and movies per day . Predicting what a user wants to watch is a key part of the company’s business model. Machine learning is reportedly integral to streamlining the diversity of user preferences.
In May 2016, Netflix announced the development of a workflow management and scheduling application called Meson to reportedly manage its various machine learning pipelines that “build, train, and validate personalization algorithms” responsible for providing video recommendations.
For a deeper look into recommendation systems, readers may find Use Cases of Recommendation Systems in Business – Current Applications and Methods to be a useful resource.
IRIS.TV – Recommendation and Personalization
Reportedly driven by machine learning, IRIS.TV offers a B2B service to support companies in tracking and improving client interaction with their digital content. Examples of media company clients include Hearst Digital Media, CBS and the Hollywood Reporter.
In the 4:25 minute interview below, IRIS.TV founder Field Garthwaite discusses the platform and his company’s collaboration with IBM Watson, which helps to drive the machine learning capabilities:
Specifically, the platform aims to match content to users based on their preferences. The software integrates into the majority of video players. The algorithms “learn” what users want to watch and recommends similar content.
In one case study, the company reports that The Hollywood Reporter-Billboard Media Group generated a 50 percent increase in viewer retention over a period of three months.
Search Optimization
Zorroa – Machine Learning for Visual Asset Management
Zorroa offers a platform for managing visual assets that reportedly integrates machine learning algorithms to allow users to perform content searches within large databases. Documents are imported into what is called an “analysis pipeline.” The pipeline is composed of processors which tag each visual asset. Algorithms are trained to recognize specific components of visual content which can then be organized and catalogued to deliver robust search results. Examples include:
- Face Recognition (find faces and decide if they are known, if so tag the asset with a name)
- Image Classification (use a neural network to classify the image into a set of predefined categories)
Once the analysis pipeline is operating, the process continues with workflow management steps allowing users to scale up the process for large datasets and fine tune categorization of visual assets. The image below depicts a visual representation of the process:
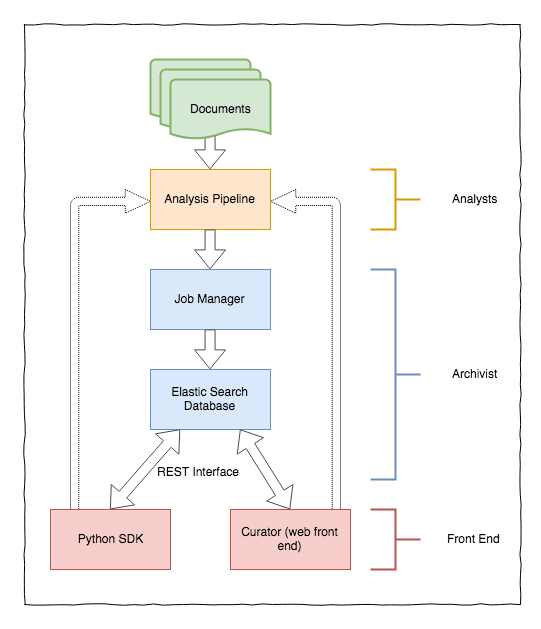
In April 2017, Zorroa Corporation announced the launch of its machine learning platform that would reportedly allow users to perform searches and run analytics on visual assets found within large databases. Zorroa calls the tool the Enterprise Visual Intelligence (EVI) platform.
The company claims that Sony Pictures Imageworks uses Zorroa EVI to “analyze and monetize millions of visual assets” that have been developed over the years. For example, in one case study, Sony Pictures claims that a specific video search that would normally take 27 hours was completed in just 3 minutes using the EVI platform.
The short 58 second video below demonstrates how the video search was performed in the EVI platform:
In another example, the company reports that it assisted a client in the oil and gas industry tag and organize images and PDFs to complete a mergers and acquisitions (M&A) valuation. Zorroa’s claims it reduced the processing time from 3 months to 1 month and increased the discoverable visual assets from 10 percent to 90 percent.
Currently, it appears that the company is targeting clients in the E&M and oil & gas industry. A platform of this kind would technically be useful for any company where organizing and accessing large databases of visual content is a regular task.
Concluding Thoughts
Emerging applications of machine learning in the entertainment and media industry are being applied to multiple areas of importance for companies including marketing, advertising and content production. Innovations poised to deliver the greatest benefit over time will save industry professionals time and improve efficiency of the production process.
We will provide our thoughts on which applications in this article appear to hold the most promise based on our research. generally ranking each from most viable to least viable:
Personalization of the User Experience
Leaders and emerging competitors in the on-demand entertainment space are leveraging machine learning to sustain their ability to personalize content at scale for every client. Now that personalization is becoming a standard client expectation, AI is poised to be an integral strategy for keeping pace with consumer demand.
Companies focused on personalization of the user experience are appearing to deliver value for their clients in the on-demand entertainment space. As competition increases in this sector, evidenced by YouTube’s marketing push of YouTube Red, machine learning will become increasingly important.
Search Optimization
Zorroa Corporation’s Enterprise Visual Intelligence (EVI) platform is meeting a practical need for film production houses, helping to improve the process of searching for specific visual assets. If the EVI platform consistently delivers the results reported by Sony Pictures, it has the potential to be widely adopted in the film industry.
Marketing and Advertising
In contrast, the current lack of data on a correlation between machine learning in film trailer developement and ticket sales makes it challenging to predict how quickly other films may follow suit. However, if the effort for the Morgan film was intended more as a marketing strategy, media buzz was indeed generated.
Nevertheless, the film industry desires tools which reduce production time and costs. Therefore, we can anticipate feedback in the coming years on which of these applications may prove most useful. These data may substantiate the value AI could bring to the industry in multiple capacities of the production process.
The challenge of implementing AI for more creative tasks, as in the case of McCann Erickson Japan’s AI creative director, may require more research before competing firms make investments in this technology. For optimum results, seasoned creative directors will need to be continually involved in the AI training and improvement process which may cost advertising agencies employee time.
Header image credit: dw.com