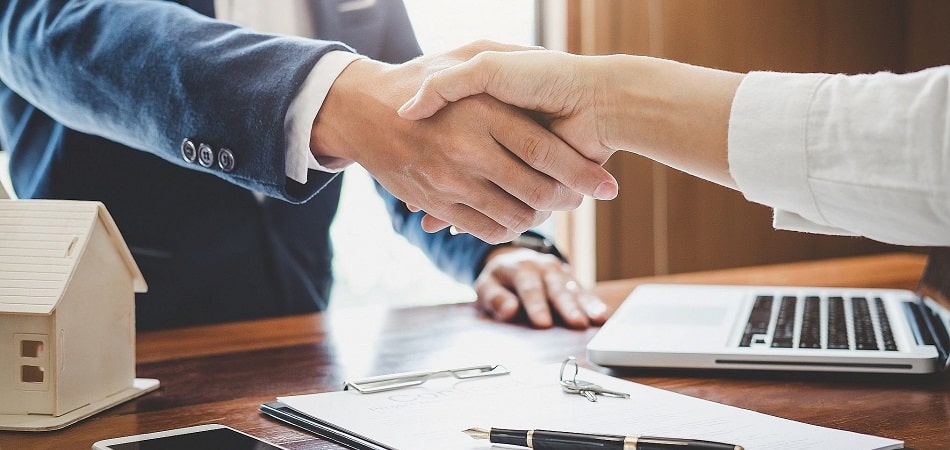
Much of the discussion surrounding AI in banking is focused on retail banks, with applications such as chatbots and payment fraud detection. But there exist AI capabilities that stand to benefit investment banking clients specifically as wealth management departments begin to adopt them.
In this article, we provide a brief overview of two AI use-cases in investment banking:
- Trade Process Automation: Using AI to optimize stock trades for individual clients.
- AI-Enabled Search and Discovery for Wealth Managers: Using AI to pull up all of the information an investment bank has on a client to facilitate better wealth management.
We begin our explanation of the benefits of AI for investment banks with trade process automation and how investment banks can benefit from optimizing their trades.
Trade Process Automation
Investment banks could leverage AI to automate trade processing for their clients. This could allow for faster trades, and some solutions could identify possible investment strategies for the customer to consider. Additionally, this type of software in some cases may be able to execute trades over multiple markets.
A trade automation solution such as this would require a machine learning algorithm in order to match buy/sell orders from traders to brokers, stock exchanges, or other trading systems that can fulfill the order. Many electronic routes limit liquidity and increase costs for the trader, but the algorithm could improve over time to find the cheapest and safest way to execute trades.
One example of this type of solution is Man AHL’s quantitative investment management platform. The company claims to use machine learning to optimize trade routing from three possible channels. These channels include:
- Internal execution algorithms: Man AHL’s trade execution and routing algorithms, which likely include trading strategies they have researched to be effective and broadly applicable.
- External dealer algorithms: Algorithms provided by third-party companies that may have more expertise in a certain business area, such as big box retail stock trading.
- The firm’s trading desk: The company’s client’s own trade processing department, provided the algorithm has determined it to be the best course of action and this has been accepted by a human monitor.
According to Man, their platform can direct trades based on historical trading data and calculate the probability of each outcome for each execution route in terms of cost and liquidity. It can also purportedly automatically route trades as an investment firm’s traders execute them. Each trade order is sent through the AI platform, which routes the trade in the most efficient manner. Usually, this means splitting the order into multiple smaller trades.
Below is a 6-minute video that explains how Man AHL’s fund manager uses AI for finance:
AI-Enabled Search and Discovery For Wealth Managers
Wealth managers could also use AI-enabled search and discovery applications, also known as intelligent search, in their day-to-day work. The high-touch, customer-focused nature of wealth management requires wealth managers stay on top of their clients’ investment preferences so they can make the best decisions for them. Wealth management departments could benefit from an AI application that pulls up all of a client’s information in one accessible view.
Citibank, for example, worked with AI-enabled search vendor Attitvio to provide bankers at its private banking arm with a “complete, unified view of their customers” within an Ipad app. Citi’s private bankers were able to better offer clients upsells and client retention offers, which the case study claims increased Citi’s revenue share.
Attivio claims it integrated its search application into Citi’s software stack within three months and that it unified a variety of data sources into one singular place, including product information from the bank’s internal database and customer information from the bank’s CRM.
Header Image Credit: Avivchen