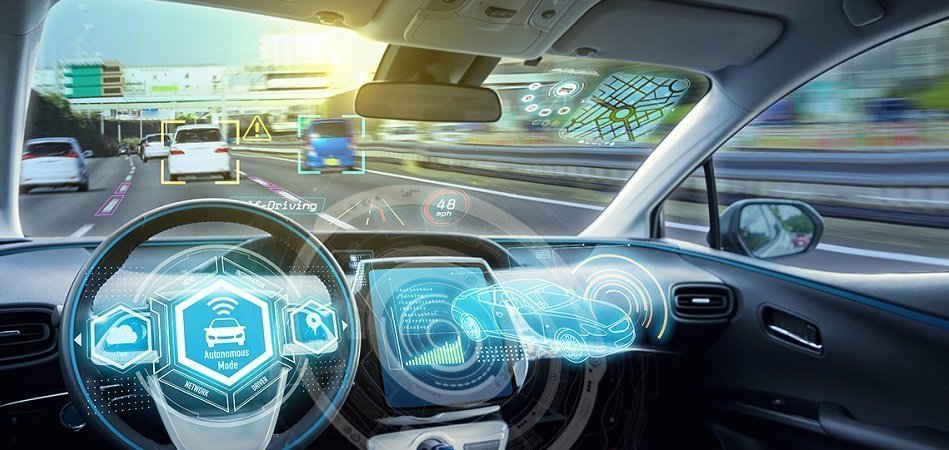
In this article, we explore the applications of AI software within the automotive industry from production and manufacturing to insurance and transportation. We will discuss the equipment involved in collecting and analyzing data along with the potential value they offer to manufacturers, shared mobility companies, insurers, and drivers.
We begin our overview of AI in the automotive industry with how machine vision technology could improve the robots that car manufacturers use to build vehicles and maintain quality control.
Car Manufacturing
The car manufacturing process is a multifaceted business in itself, so it follows that there would be numerous areas where one can find applications for AI technology. Factories can monitor the condition of production equipment and heavy machinery with IoT sensors and predictive maintenance.
Each sensor is attached to a piece of equipment and collects vibrational data whenever the equipment moves or is used. Changes or anomalies in these vibrations can indicate damage or natural wear over time and help business leaders prevent equipment failure by predicting it and performing necessary maintenance before the assembly line needs to stop out of emergency.
Computer vision also has many applications at the factory level. This includes quality control during production. Factories can leverage this type of software to detect flaws or inconsistencies across produced cars and highlight areas where the new car may need to be worked on further before going to market.
For example, the computer vision software of a camera stationed at the end of the assembly line could find flaws in a car’s paint job or identify parts that were not attached correctly, such as windshield wipers.
In addition to the full automation of manufacturing processes, computer vision is also useful in making factories safe for human employees and robotic processes to coexist. The ability to detect all objects in the factory environment is essential to creating AI software that can collaborate with human employees.
This is because as machine learning algorithms get more advanced, the opportunity to create more general use robots for the factory setting will arise.
Companies such as Rethink Robotics are creating collaborative robots that can respond to and learn from a human instructor. The human working with the robot can guide the robotic arms through a task, which can effectively “teach” the robot the correct way to hold something, drive in a screw, or connect two parts of a car.
Supply Chain Optimization
Machine learning and big data analytics can play a role in the manufacturing supply chain in addition to predictive equipment maintenance. Business leaders can use predictive analytics to forecast spikes in demand, which would allow them to plan their procurement of materials accordingly.
This affects the ability of the factories to provide products that will be in demand in the near future, and it reduces waste and overpayment for the required resources.
Predictive analytics can also be used for quality control across the chain of production and into customer sales and aftermarket information. Manufacturers can instrument their supply and assembly chain with IoT sensors, machine vision cameras, or large data stores that are constantly logging new product information.
They can then run their collected data through a machine learning model in order to organize an all-around view of quality control for their product. Information about materials and setup can be found from supply chain data, while the procurement stage offers information about dealer sales and past transactions.
Manufacturing quality control can analyze data from IoT sensors or machine vision cameras to detect and record how factory equipment is being used and if the current process produces the best product. Research and development data is also incorporated here, as new car parts and assembly processes would likely be tested at the factory level.
Data for predictive analytics can also be found in marketing campaigns and information regarding the product’s transportation and distribution. Marketing teams with systems that are integrated into the manufacturer’s data storage can be valuable in providing customer and competitor data.
Business leaders could even look to a natural language processing solution here in order to gauge the sentiment from social media posts about the product. When it comes to transportation and distribution data, manufacturers can learn how their product is being carried to dealerships and find how many cars sustained any damage on their way to market.
It should be noted that this data cannot all be simply procured by instrumenting one’s supply or manufacturing chain. Some data may not be accessible to manufacturers at all without collaboration with a more customer-facing company.
Procuring certain types of data like marketing, customer satisfaction, distribution, and rising demand would require manufacturers to establish closer relationships with car dealerships, partnered companies, and shared mobility services. Between these companies, data could be shared in order to give each business leader more information to base their analytics projects on in the future.
Shared Mobility Services
Predictive analytics has use cases in numerous core processes for shared mobility services such as Uber or Lyft. Companies can adjust ride sharing prices in different geographical locations based on real-time predictions of supply and demand. This allows them to accurately price rides in areas with low concentrations of drivers but high concentrations of customers.
Machine learning could also be leveraged for determining the allocation of rides to specific customers. An algorithm could determine the most convenient choice of driver for each customer. This would be focused on keeping wait times as short as possible and avoiding making drivers go out of their way for rides another driver could have more easily chosen.
Shared mobility services can also use predictive analytics to create routing algorithms based on traffic flow data. This could include time-sensitive traffic flow data such as traffic levels at rush hour across different areas of a city or data from emergent events such as car accidents or road work detours.
All of this data combined would allow for a routing system that can determine the best route for the time of day while keeping track of any obstructions on the route or other routes close by. Companies could even have time estimates for other routes listed for the driver on their GPS map, giving more freedom of choice while navigating through traffic.
Shared mobility service with proprietary vehicles such as Zipcar can make use of predictive analytics in scheduling maintenance for their vehicles. IoT sensors are likely to be used for detecting wear and tear and the probability of car failure, and the company can decide which cars are at the highest priority for maintenance based on that data.
Zipcar stores and stations can also provide customer transactional data, which may be helpful in finding out why a car needs maintenance sooner than usual. If a customer broke something on or inside the car, that information would be stored along with the receipt for their transaction, giving the company more information to determine maintenance priority.
Automotive Electrification
Manufacturers of electric cars have some specific information they can glean from incorporating data analytics into their research and development. If a manufacturer is able to get data from partnered companies like car dealerships and shared mobility services, they can find information pertaining to car electrification and manufacturing. This information could be valuable for electric vehicle and battery design, and the design and implementation of charging infrastructure.
The most efficient AI application for finding this information is likely predictive analytics, because of its ability to determine possible outcomes from data points from disparate sources. This way the software can process information from electrical telematics sensors as well as numerical database information.
A company could find out the design of their electric car’s battery does not hold a charge for as long as competitors, or that customers frequently have difficulty keeping the battery charged or figuring out how to charge it at all.
Customer data from shared mobility services could also reveal problems with the charging infrastructure for the manufacturer’s model, such as not being user-friendly or prone to malfunction. Further information on malfunctions like this could also come from IoT sensors detecting the vibrations of plugging the car in or customers interacting with the equipment.
The design of the electric vehicle itself may be a concern for manufacturers looking to create new electric models based on their past experience with building and selling them. Customer information from third parties and partnered shared mobility services may be one of the most valuable data sources.
This is because it would contain the most information on how well the model works for customers and how convenient charging the model is. This information could include data about customer satisfaction, but also about driving habits and their effects on the car’s battery.
Electric car manufacturers can find information about improving their battery design beyond driving value just in how long the battery can hold a charge. They may also find inefficiencies in the shape of the battery or in its placement within the car model. Placement may also contribute to ease of charging and future changes to charging infrastructure to make it more easily usable to the average customer.
Auto Insurance Adjustment
Both predictive analytics and computer vision have use cases in automotive insurance, each of which have been explored by numerous AI vendors selling into the insurance industry and are driving business value. Companies like Nexar and TrueMotion use dashboard cameras, smartphone cameras, and IoT sensors to detect when the car is in motion.
While in motion, the cameras paired with computer vision software can detect other objects on the road, hard breaks as the car stops abruptly, and any accidents. All of these may affect insurance deductibles or premiums, and so the videos can be shared with insurance companies so that they may adjust the driver’s insurance accordingly.
In addition to instrumenting individual car rides to find incident information, machine vision can also be used to determine damage to cars as a result of a driving accident. Certain solutions allow users to upload images from all around their damaged car, and the software can detect how severe the damage is and provide an estimation of the repair costs.
In these cases, the images and estimates are also shared with a human insurance agent for approval. Before underwriting a customer’s claim, they can review the computer vision results and look at the pictures of the damage themselves. This would create a faster and more thorough underwriting process than if the insurance agent were left to analyze the car’s damage on their own.
Companies like TrueMotion have partnered with insurers to improve their telematic insurance adjustment solutions with AI. They have developed an application that can detect driving habits and hard breaks but have also worked to make it compatible with Progressive Insurance’s existing ODB telematics device.
Their telematics device, called Progressive Snapshot, served many of the same functions before smartphones became mainstream. It now remains part of their Snapshot service in case a customer does not have a smartphone or does not want their’s connected with Progressive. Progressive now collects insurance adjustment data from their ODB telematics device as well as the smartphones of users of the Progressive Snapshot app powered by TrueMotion.
Self-Driving Cars and AI Driving Assistants
The topic of self-driving car technology can seem ubiquitous in conversations about use cases for AI in the automotive industry. Companies like NVIDIA, Tesla, and Google Waymo are still working on providing fully autonomous vehicles, along with gaining the trust of the public to eventually make the technology legal across the United States.
Autonomous vehicles most commonly use computer vision to detect objects, streetlights, and pedestrians. However, they also make use of radar frequencies and LiDAR, a laser distancing system using the same operating principles as radar. This allows the machine learning algorithm behind the car to detect how far away different objects are from the car, such as other cars, pedestrians, and obstructions such as detour signs or traffic cones.
Before fully autonomous driving becomes mainstream, drivers will likely become accustomed to AI assisted driving, which is an application that leverages machine vision to detect driver activity while they are on the road.
AI driving assistant programs can help to ensure driver safety and may pose the most potential value to insurers who want to keep their customers safe and responsible.
This technology focuses on the facial movements of the driver by detecting eye movement and alerting the driver when they’ve diverted their gaze from the road. The algorithms behind these assistant programs can also track lip movements and in some cases speech.
However, detecting the driver’s speech when talking with other passengers would require a natural language processing algorithm on top of the machine vision technology.
In response to an inquiry from the New York Times, Ola Bolstrom, VP of Research, Innovation, and IPR at Veoneer, said, “Cognitive load can also be assessed using eye motions. Attention to driving can be evaluated by whether people in the car are talking to one another or thinking about what to say.”
Insurers and other companies looking to instate an AI driving assistant can also set up the system to give drivers sound alerts when they are looking down into a bag or at their phone screen to read a text message.
This way, the assistant discourages distracted driving while keeping track of the driver’s activity. The technology could also use multiple cameras to capture nearby or oncoming objects and alert the driver of them so they can react accordingly.
Header Image Credit: AARP