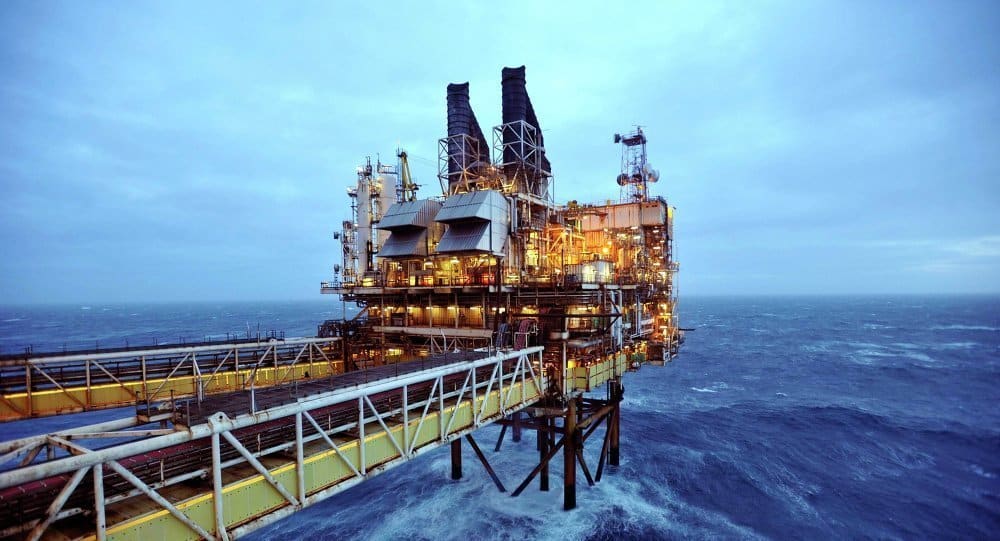
The oil and gas industry is usually divided into three major operational sectors: upstream, midstream, and downstream. Upstream involves the exploration and production of oil and natural gas. Midstream usually refers to transportation and storage stages. Downstream encompasses the various processes involved in refining and selling oil.
The increasing demand for oil and gas makes the discovery of new oil fields a high priority need for companies in this space. This operation is generally termed the Exploration and Production (E&P) or the upstream level of the oil and gas industry. Finding ways to make E&P processes more efficient and optimize operations in this field are applications where AI might help oil and gas companies.
We previously covered the applications of AI in the oil and gas sector. In this report, we focus specifically on companies that claim to assist oil and gas E&P professionals in aspects of their roles from analyzing drilling data to identifying new subsurface oil fields.
We researched the space to better understand where AI comes into play at the upstream level of the oil and gas industry and to answer the following questions:
- What types of AI applications are currently in use at the upstream level of the oil and gas industry?
- What tangible results has AI driven at the upstream level of the oil and gas industry?
- Are there any common trends among these innovation efforts? How could these trends affect the future of the oil and gas industry?
This report covers vendors offering software across the following applications:
- Oil Corrosion Risk Analysis
- Data Analysis
- Exploration
Oil Corrosion Risk Analysis
In the production stage, oil and gas companies need to store crude and refined oil in large tanks and transport it through pipelines. Corrosion by crude oil is a common risk for equipment failures in the oil and gas industry. Crude oil from oil fields usually varies in its chemical compositions and the corrosiveness of the crude also depends on the environment it is stored in.
Traditionally in the oil and gas industry corrosion engineers have learned what solution to design to prevent corrosion based on the properties of the crude and the storage area. Business leaders in the oil and gas industry might be familiar with the Great Crew Change, referencing the large age gap in the oil & gas workforce, where most engineers and geoscientists are either over 55 or under 35.
What this means in real-business terms is that oil and gas firms have not had the best process to capture and transfer the knowledge from veteran engineers in a repeatable manner. Digitizing this knowledge and delivering maintenance insights to new engineers might now be possible with AI.
Maana
Maana is a Palo Alto California-based company with over 100 employees. The company offers a software called Computational Knowledge Graph, which it claims can help oil and gas companies reduce unplanned maintenance from oil corrosion using predictive analytics.
According to Maana, oil and gas companies can deploy their Knowledge Platform for use in production operations. The platform can be used to aggregate knowledge from maintenance data, such as refinery incident reports and data about the physical properties of different types of crude that were collected by maintenance engineers over time.
The platform reportedly uses machine learning to analyze the data and identify factors that led to corrosion in previous situations. Maana claims their platform could then summarize the insights gained from this analyses in a web portal dashboard that allows engineers to search for the required information about corrosion risk as shown below:
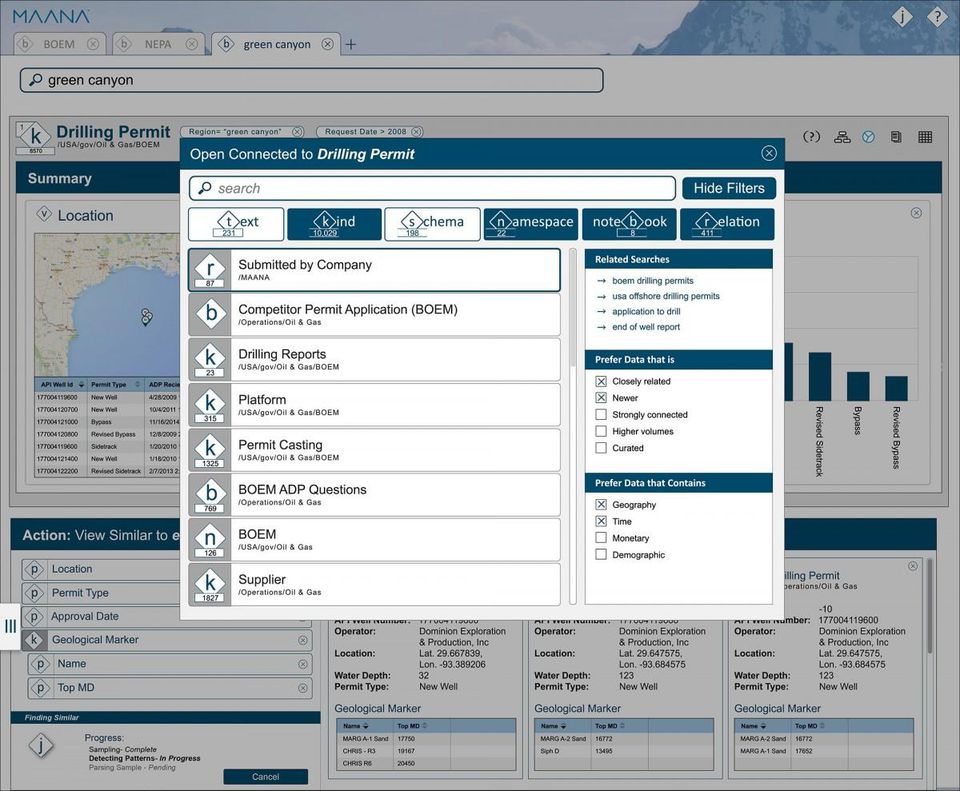
Maana claims to have helped an unnamed oil and gas company develop an analytics solution to predict corrosion risk. The oil firm deployed the Knowledge Platform and created a team that included Maana AI engineers and corrosion engineers from the client.
The team built a searchable database using historical maintenance data called the Crude Flex Knowledge Application, which captured the expertise of veteran experts. According to Maana, corrosion engineers at the oil and gas firm can now use it to identify the types of crude they are working with and discover risks, lessons learned and risk mitigation strategies based on the historical records. The project eventually resulted in reduced maintenance costs and unplanned downtime, although we could find no further concrete evidence for this.
We were unable to find any mention of enterprise-level companies on Maana’s website nor in any of their press releases, but they have raised $71.2M in funding and are backed by Accenture Technology Ventures, CICC, Eight Capital, Sino Capital Group.
Steven Gustafson is the Chief Scientist at Maana. He received his PhD in Computer Science from the University of Nottingham, UK. Previously, Dr. Gustafson led the Knowledge Discovery Lab at the General Electric Global Research Center in Niskayuna, New York, and worked on large-scale data, semantics, ontologies and text mining, and pattern search and discovery.
Data Analysis
Large oil and gas companies operate out of several different locations globally. Quick access to their data records might be a vital part of their business processes. In many cases, these data records might need to be digitized and assessed to identify issues such as missing or incomplete records or unindexed records before they can be properly utilized.
AI tools can help oil and gas companies digitize records and automate the analysis of geological data and charts, potentially leading to the identification of issues, such as pipeline corrosion or increased equipment usage.
Alejandro Betancourt, who holds a PhD in Interactive and Cognitive Environments from the Eindhoven University of Technology and leads the analytics team at Columbian oil and gas company Ecopetrol seemed to suggest that the oil and gas industry has collected data over the years that might have been curated and analyzed by human experts, making it ripe for feeding into AI systems.
A large part of this data includes exploration, production and reservoir data logs, such as seismic surveys, well logs, conventional and special core analyses, fluid analyses, static and flowing pressure measurements, pressure-transient tests, periodic well production tests, records of the monthly produced volumes of fluids (oil, gas, and water), and records of the monthly injected volumes of EOR fluids (water, gas, CO2, steam, chemicals).
Oil well operators usually generate daily reports on inspection and maintenance operations at the oil field. In most cases, these reports might be unstructured or semi-structured documents on paper or in the form of PDFs. Researchers claim that oil and gas companies can use AI technologies such as computer vision and natural language processing to extract data from these documents automatically.
Baker Hughes and Nvidia
Baker Hughes, a GE oilfield services company (BHGE) and NVIDIA recently announced a partnership to use AI-based analytics to help oil and gas companies gain insights from their data. This includes seismic data, well logs, data from sensors in machinery, and supply chain data that can be input to AI software to gain insights aimed at reducing the cost of exploring, extracting, processing, and delivering oil.
According to Baker Hughes, oil and gas companies collect large amounts of ultrasound data, production process data, drilling operations data, pipeline inspection data, and seismic data from their plants every day. Oil and gas firms may need a way to better utilize this data and gain insights to improve operational efficiency.
Baker Hughes claims to have developed a deep-learning based analytics software that helps employees in oil and gas firms make better business decisions based on the data they are collecting. For instance, the company claims well operators can use their solution to analyze large amounts of production and sensor data, including pump pressures, flow rates, and temperatures to predict which of their equipment and parts might fail.
Baker Hughes also claims to help oil and gas firms optimize their operations for different environmental conditions. For example, seismic data images can be turned into 3D maps to help human analysts make better reservoir predictions aimed at improving the efficiency and safety of drilling and production operations. The system then provides outputs in a dashboard signifying insights gained from the data, such as 3D visualizations of the seismic data or a list of equipment and parts in the production plant that are at high risk for failures.
We could find not a video from Baker Hughes or Nvidia demonstrating how their product works. We also must state that the partnership between Nvidia and Baker Hughes seems to be in its beginning stages, and we could not find any case-studies or evidence of AI projects that the companies have worked on with oil and gas firms.
Shyam Sivaramakrishnan is Director of Analytics Advocates at Baker Hughes. He holds a PhD in Mechanic Engineering from the University of Minnesota-Twin Cities. Previously, Sivaramakrishnan served as Technology Project Manager at GE Global Research.
Total Oil and Google Cloud
Total Oil recently announced an agreement with Google Cloud to jointly develop an AI system to analyze subsurface data aimed at improving their E&P processes.
Total’s E&P process involved mapping and analyzing the seismic activity in a potential oil field. Traditionally, much of the data collected by the company, such as seismic records, were image files that geologists analyzed manually. Total wanted to automate the digitization of this analysis and create a searchable portal for their employees where this could be used to identify patterns indicating any economic liabilities a new field might bring to the company.
The seismic and subsurface data charts were collected in the form of image files and were taking too long for manual staff to analyze. Moreover, these data charts were stored in several different locations, usually the premises of the oil field or in the cloud in different formats. An example of a seismic data chart is presented below, courtesy of Analytics Magazine:
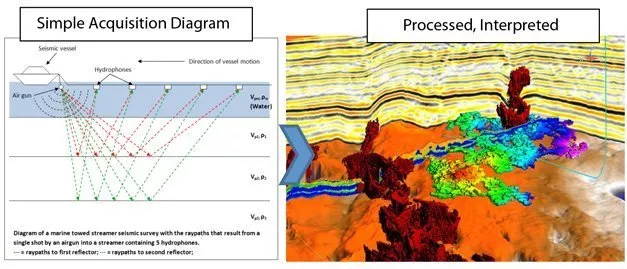
Total and Google are collaborating on this project to develop AI systems that can help geologists at Total interpret subsurface images from seismic studies faster using computer vision. The aim of the project was to develop a software that could analyze the image files, correlate the findings with information extracted from technical documents and summarize this information into a queryable AI assistant that can answer questions on the subsurface data in natural language.
According to the press release, a multidisciplinary team including geologists from Toal and machine learning experts from Google Cloud are working alongside each other at Google’s Advanced Solutions Lab in California to develop the software.
Total claims to have applied machine learning to other exploration and production activities, including predictive maintenance for turbines, pumps, and compressors at its industrial facilities production profile forecasting; automated analysis of satellite images; and analysis of rock sample images. That said, we could not find more information on these projects.
Total exclusively uses their AI systems internally, and so there are no available case studies for the software. Additionally, information on the person who is in charge of the project does not seem to be available.
Exploration
ExxonMobil and MIT
ExxonMobil is working with researchers from MIT to automate the hydrocarbon exploration process by jointly developing deepwater exploration robots. Professor Brian Williams from MIT and his team at MIT’s Computer Science and Artificial Intelligence Laboratory (CSAIL) had previously worked on NASA’s Curiosity rover and designed the artificial-intelligence software that helped the rover actively explore its surroundings.
The team from MIT is working with engineers from ExxonMobil to apply similar AI software for underwater exploration robots. These robots will be used to automate the natural seep detection and characterization process. Traditionally, oil and gas firms used a team of divers and human geological analysts engaged in the exploration of underwater anomalies. With the autonomous underwater robot, ExxonMobil might be able to allow their human employees to stay out of harm’s way.
The robot collects seismic data and is equipped to learn how to perform more accurately using machine learning. ExxonMobil claims these robots will move slowly a few feet above the ocean floor to detect and analyze naturally seeping hydrocarbons.
This application of AI might be able to help oil and gas companies reduce exploration costs and time. The main objective for ExxonMobil’s work with MIT, however, seems to be in gaining experience in advanced technologies. Hans Thomann, Senior Scientific Advisor at ExxonMobil and a visiting scholar at MIT says:
“We work with MIT on a spectrum of programs, intentionally opening the aperture of our lens to look at things we normally wouldn’t look at. We focus on fundamental science, working with experts across diverse fields at the university to gain deep knowledge and advance those fields.”
Takeaways for Business Leaders in Oil and Gas
Oil and gas companies could potentially gain insights to improve business outcomes in their upstream processes by using AI software. This would involve feeding the software with the curated data records and information from data sources that could include structured documents, PDFs, handwritten notes, audio, or video files.
These applications can help oil and gas companies drive business value in the following areas:
- Production optimization – Example, Computer vision to analyze seismic and subsurface data faster
- Downtime minimizing – Example, Predictive maintenance for oil and gas equipment
- Reservoir understanding and modeling – Example, Predicting Oil Corrosion risks to reduce maintenance costs
Business leaders in oil and gas need to be aware of the fact that despite the seemingly large number of uses for AI in the oil and gas industry, it is a challenging area for many companies in the space due to the technical complexity of the analysis and the computational resources required.
Based on the use-cases we researched, it seems as though AI can help oil and gas businesses make better use of their historical data. That said, the proliferation of AI in oil and gas can still be termed nascent as compared to healthcare or finance. Large oil and gas firms like Total seem to have followed a strategy of collaborating with Enterprise level AI companies. Applications like predictive maintenance have several smaller AI vendors offering products and services, but the industry seems to have a shortage of well-established use-cases and reports on successful ROIs.
Header Image Credit: Sputnick News