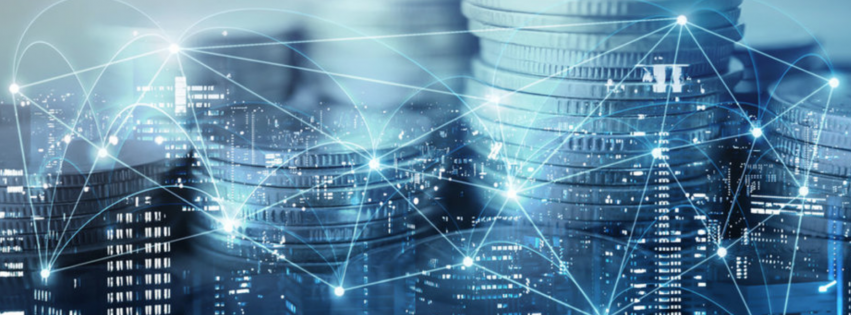
In their recent Worldwide Spending on Cognitive and Artificial Intelligence Systems report, IDC estimated that banking investments in cognitive and AI systems in 2018 might total around $4.0 billion. Many AI vendors claim to offer software for numerous finance use cases, such as credit scoring, insurance underwriting, debt collection, fraud detection, and more recently, financial regulations, or so-called regtech.
In this report, we’re going to take a broader view than our previous reports, but focus on three applications in particular:
- Business Intelligence and Analytics for Algorithmic Trading
- Business Intelligence and Analytics for Report Generation
- Chatbots for Customer Service
Report Generation
Yseop
Yseop is a Paris-based company with 58 employees. The company offers a software called Yseop Compose, which it claims can help banks and financial institutions automate report generation using natural language generation, or NLG. Natural language generation is the process of generating natural language text from data which is usually stored in the form of machine-readable databases.
Yseop claims users can install their software on enterprise computers and input it with financial data, data for risk analysis, compliance data, and other kinds of internal business data. The software uses a tool called the Yseop Rules, which purportedly helps users contextualize their information.
A team of subject-matter experts from the client company works alongside Yseop developers to help organize, classify, and understand the context behind the data that is fed to the software. For example, the bank’s financial analysts might tag parts of an internal business report as relevant to taxation. Once the software learns through this “tagged” data input, it can identify and correlate more such content over time.
The Yseop Rules tool allows users to create a custom dashboard where enterprise employees can enter values for business metrics. For example, a client might want to measure the total assets held in certificate deposits and want the data to be represented in narrative text for use by non-technical or non-data scientist employees.
Yseop Rules allows the client to create a dashboard listing the relevant factors that might affect this metric, such as the directional change in asset value and a slider for the strength and duration of the change. The client can then generate unique narrative reports for different values entered for the business metrics.
Then, Compose uses these business metrics and the context gained from subject matter experts to convert data into a readable report. The system then provides a full report that can be accessed on the Yseop Compose dashboard or extracted in the form of word docs or PDFs. In the example considered this would be a narrative report on the deposits, savings, equity and other factors related to the total assets held as shown in the figure below:
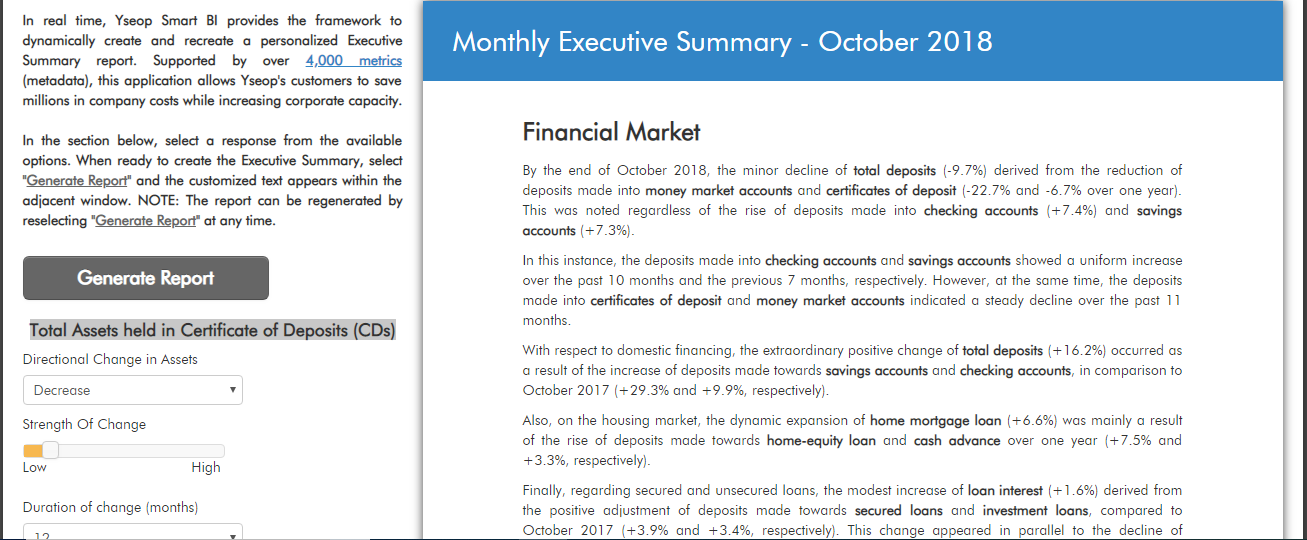
Yseop provides an online demo for their natural language generation software. Interested readers can see how their software generates bespoke reports for a company by using an example topic.
The company also provides Yseop Savvy, which can automatically convert graphs and charts from excel files into natural language reports.
Below is a short 4-minute video demonstrating how Yseop Savvy works:
Yseop does not make available any case studies reporting success with their software.
We could not find mentions of enterprise level clients for Yseop, although they list Accenture, Tableau, and Qlik as some of their partners.
Alain Kaeser is Chief Science Officer at Yseop. He holds an MS in Computer Science from Pierre-and-Marie-Curie University, Paris and a degree in Mathematics from the École normale supérieure Paris-Saclay. Previously, Kaeser served as Professor of Computer Science at École normale supérieure Paris-Saclay and was the founder of AI startup Cognitive Relation.
Narrative Science
Narrative Science is a Chicago, Illinois-based company with more than 100 employees. The company offers a software called Quill, which it claims can help financial institutions generate narrative reports from structured data, such as sales records, using natural language generation.
Narrative Science claims users can integrate the Quill software as a part of their existing business workflows such as automatically generating narrative sales reports for regional managers. According to Narrative Science, human analysts and subject matter experts are needed to train Quill to learn the formats and context involved in the existing reports generated by humans.
Then, the data, tagged and annotated by the experts, allows Quill to “understand” which parts of the reports are the most relevant and generate reports that are comparable to the existing historical records in terms of sentence lengths, structures, and word variabilities. The system then provides a narrative report that can be understood by non-technical employees and can be presented as a word doc or PDF.
According to Financial Crimes Enforcement Network (FinCEN) regulations, banks and financial institutions are required to file a Suspicious Activity Report (SAR) with the organization upon detecting any suspicious transactions or money laundering event within 90 days of such activity. Narrative Science claims to have helped an unnamed US bank automate the generation of SARs by working alongside the bank’s fraud detection team.
The Quill software was first input with existing SAR reports and trained by the combined team to understand context and formats for the SAR. The software was then used to automatically generate SARs, enabling the bank’s 20 person investigations team to handle around 3,500 SAR reports every month. According to Narrative Science, this resulted in the bank saving $225,000 annually and reducing the time taken for generating each SAR from 4 hours to 1.5 hours.
Narrative Science also lists Credit Suisse as one of their past clients.
We could not find evidence of any C-level executives on the team with previous educational or work experience in AI projects, but they have raised $43.7 million in funding.
Algorithmic Trading
Kavout
Kavout is a Washington-based company with 22 employees. The company offers a solution called Kai Score, which it claims can help investment and equity firms predict the performance of securities in a portfolio using predictive analytics, big data, and machine learning.
Kavout claims their software can help investment and equity firms analyze large amounts of data, including SEC filings, technical indicators, price patterns, news, blogs, analyst reports, and social media feeds. Kavout claims their software can then identify complex patterns in the financial markets to predict the performance of an asset.
The company offers this service through a web portal where brokers can log in to view stock performance notifications customized for them based on their trading history.
Users can access a dashboard on the portal which offers insights on the predicted performance of the user’s preferred stocks. Then, Kavout’s software applies machine learning algorithms to categorize and assess the impact of the identified financial patterns and trends. The video below explains how this is achieved in more detail:
According to Kavout, users can then access predictive insights and an actionable numerical rank called a Kai Score for each of their preferred stocks. Higher Kai Scores indicate a higher probability of a particular stock outperforming its historical trends in the next one to three months.
Investment firms might use the Kai Score to assess which stocks to pick for a particular portfolio. Additionally, the score can be used to support the investment firms existing quantitative models for algorithmic trading.
For example, the screengrab below shows the predicted Kai scores for a number of different portfolios of stocks in the S&P500:
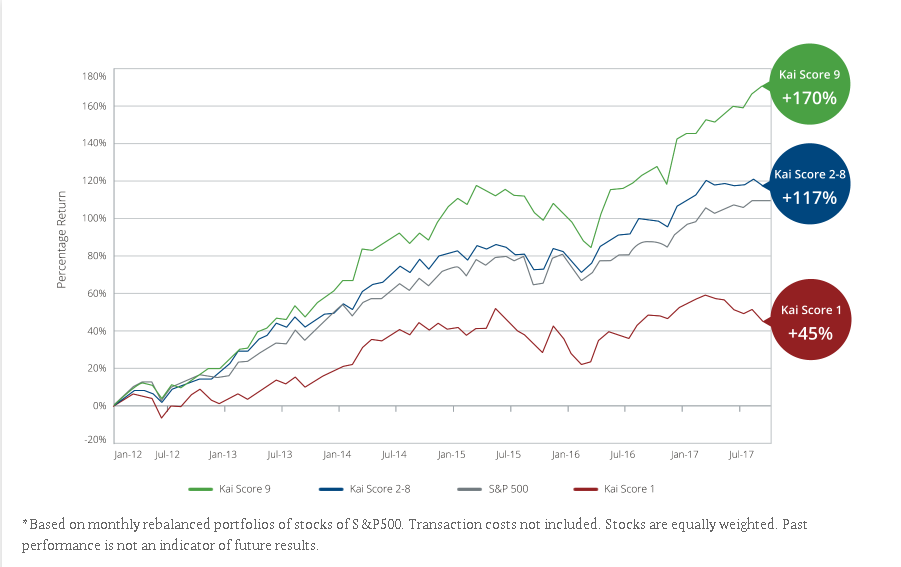
In the first 4 minutes of the video below, CEO Alex Lu, demonstrates how the Kai Score works:
Kavout does not make available any case studies reporting success with their software.
Kavout does not list any major companies as clients, but they were identified by Nvidia as one of the Top 5 Early Stage AI Startups in China at GTC China 2017.
Alex Lu is CEO at Kavout. He holds a PhD in Computer Science from the University of Maryland from. Previously, Lu has also served as Senior Software Engineer at Google, Principal Program Manager at Microsoft and Engineering Director at Baidu.
Customer Service
Kasisto
Kasisto is a New York-based company founded in 2015. The company offers a conversational AI platform called KAI, which it claims can help banks and financial institutions develop chatbots to improve customer experience using NLP.
According to Kasisto, users can deploy their chatbot on messaging apps and websites such as Facebook or Whatsapp. Banks and financial firms can input KAI with historical customer transaction records or account details to help train the conversational agent.
Then, KAI software uses natural language processing techniques to understand the context behind customer queries. Kasisto claims their software achieves this in four steps: the software first uses natural language processing to take in search queries and requests from users and understand what is being said. Then an AI interpreter tool which is trained by subject matter experts is used to understand the context around the user inputs to the chatbot.
The software also includes an AI reasoner tool, which identifies ambiguous statement, asks follow-up questions, and manages the flow of the conversation. Lastly, the software uses natural language generation to retrieve the information requested by the user from a knowledge database.
This enables banks to offer several services through the chat interface, such as product discovery, allowing customers to apply for loans, and answering customer queries on transaction and account details. In cases where the chatbot is unable to retrieve information, the conversation is automatically switched to a human customer service representative.
Below is a short 3-minute video demonstrating how KAI works in a collaborative project with Mastercard:
Kasisto claims to have helped JP Morgan’s treasury services division offer clients a chatbot that can answer customer queries. JP Morgan deployed the chatbot solution to help its customers navigate through their website’s 1,200 pages in order to accomplish tasks such as sending wire transfers or exporting data from multiple accounts to determine balances. According to Kasisto, the chatbot is still being tested by JP Morgan in a pilot project.
Kasisto also lists DBS and Mastercard as some of their past clients.
Sasha Caskey is co-founder and Chief Technology Officer at Kasisto. He holds an MS in NLP from the University of Columbia in New York. Previously, Caskey served as a software engineer at IBM Research.
Personetics
Personetics is a London-based company founded in 2010. The company offers a software called Assist, which they claim can help banks and financial institutions create conversational agents aimed at improving customer experiences using NLP.
Personetics claims banks can integrate the Assist chatbot into websites, mobile apps, Facebook Messenger, or Amazon’s Alexa. The Assist chatbot can be trained using historical customer transaction records, such as emails, customer feedback forms, and calls transcripts.
The chatbot then uses NLP to “understand” customer queries and take them to the right landing page for the bank’s services. Personetics works with banks to first create a knowledge database for all the customer interaction historical data from the bank.
This knowledge database converts the data into a machine-readable format, which can be queried by the chatbot to return links or narrative text replies to the user. The chatbot also analyzes customer interactions to recommend other products and services the customers might be likely to use.
Below is a screenshot from Personetics showing an example conversation for how Assist works:
Personetics claims to have helped Royal Bank of Canada deploy the NOMI Find & Save, a chatbot on their mobile app. NOMI Find & Save was designed to identify patterns in customer transaction data and automatically allocate some funds to save up for the user. According to Personetics, customer engagement with Royal Bank of Canada mobile app increased by 20% within the first five months of deployment.
We were unable to find any mention of enterprise-level companies on Personetics’ website nor in any of their press releases, but they are backed by Carmel Ventures, Sequoia, and Lightspeed Venture Partners.
We were unable to find evidence of C-level executives with AI experience on the company’s team, although the company claims that COO and Co-Founder David Govrin has expertise in machine learning and analytics algorithms. We could not verify this on Govrin’s LinkedIn page.
Takeaways for Business Leaders in Finance
Based on our research, business intelligence and analytics seems to be the business function with the most traction for AI in finance currently, with applications such as automation of report generation, predictive analytics, and text and data analysis.
As of now, the top four AI applications in FinTech are most likely algorithmic trading, fraud detection, portfolio management, and insurance and loan underwriting.
In the customer service horizontal, AI is actively being used by large investment banks and finance companies to handle the massive customer query and feedback volumes they receive. Additionally, these chatbots can offer some basic banking services, such as allowing customers to make payments or check their account balances.
Customer service might still be a softer benefit for larger finance companies than a hard-line return on investment by using AI to find new revenue pockets. In this sense, companies looking to implement AI for automating business functions might need to be prepared for a three to six month integration period along with the challenges of hiring good Ai talent and the high costs involved in AI projects.
Header Image Credit: Govtech