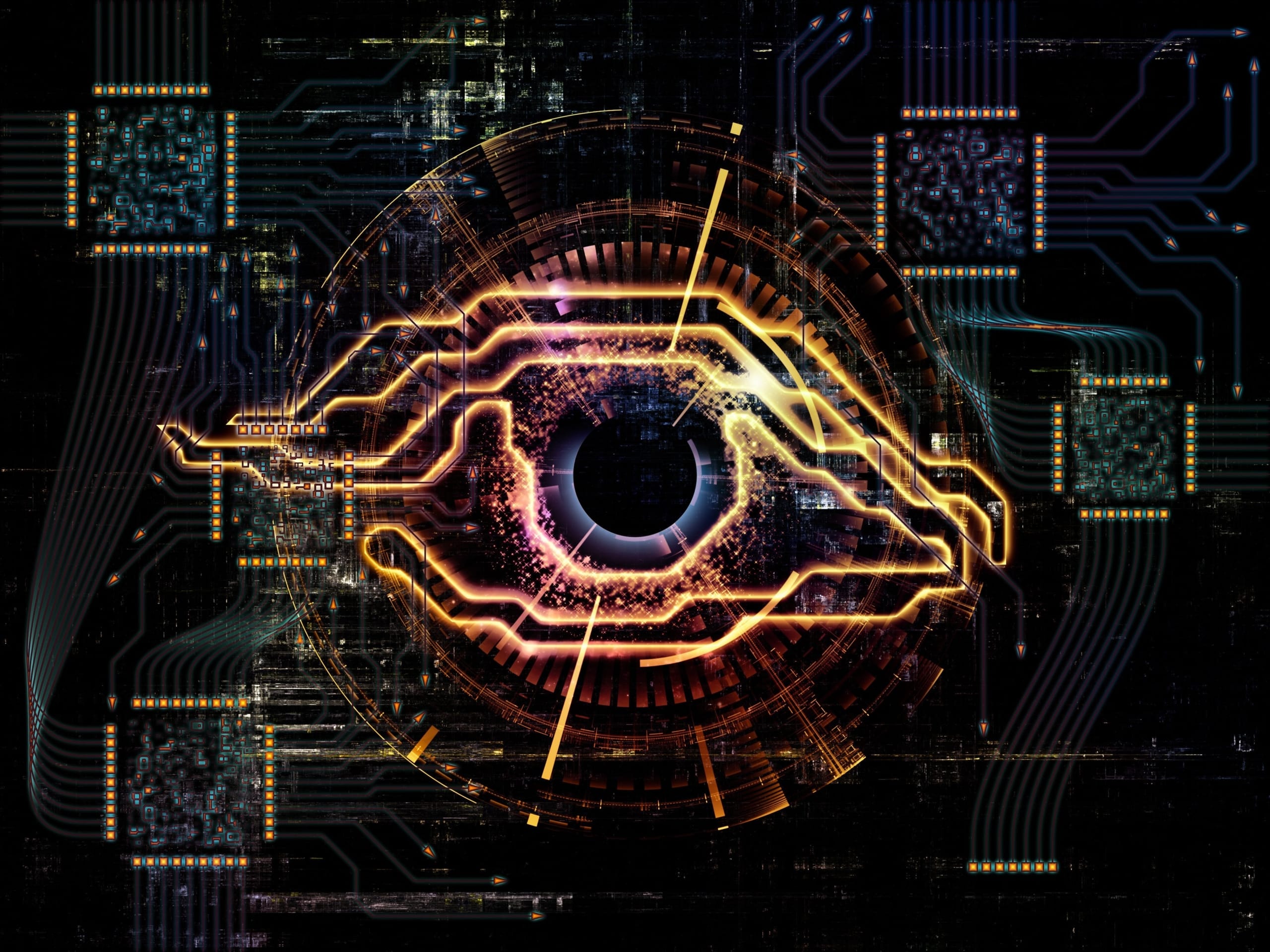
Episode summary: In the last two or three years we at Emerj have witnessed a definite uptick in AI applications like predictive maintenance and heavy industry. Many exciting business intelligence and sensor data applications are making their way into “stodgy” industries like transportation, oil and gas, and telecom – where machine vision has countless applications.
We had caught up with Massimiliano Versace, CEO of Neurala over 4 years ago in an interview about the ethical implications of AI. In this week’s episode of AI in Industry, Max speaks with us about how machine vision and drones can be used together to automate the process of facilities and heavy asset upkeep. Max walks us through potential applications in telecom and rail transportation and explains where he thinks machine vision has the strongest potential to impact the bottom line.
Business leaders who manage heavy assets or physical infrastructure should find this interview insightful, as Max explains both current and near-future applications for machine vision for maintenance and upkeep.
Subscribe to our AI in Industry Podcast with your favorite podcast service:
Guest: Massimiliano Versace, CEO, and President, Neurala Inc.
Expertise: Artificial intelligence, deep learning, neural networks
Brief recognition: Max earned Ph.Ds in experimental and cognitive psychology in 2004 and cognitive and neural networks in 2007 from the University of Trieste in Italy and the Boston University respectively. While at Boston University, Max served as the Project Coordinator and Senior Investigator for the DARPA SyNAPSE Project in 2009. Over the next seven years he served as the Director of the Neuromorphics Lab at Boston University. Max founded Neurala in 2006.
Big Idea
Max explains that the first step in AI adoption first requires a clear business case for using AI. He goes on to say that it is important for business leaders to be aware of the fact that the business case for AI is stronger in some industries or functions than others today (depending on the potential economic impact of the application, the prevalence of data, and other factors).
Max believes that in the infrastructure sector, there is a strong case of quantifiable business savings to apply AI. Max takes the example of telecom tower inspection and maintenance (a current focus area for Neurala’s work). Max tells us that AI can today look at images of the tower captured through drones from multiple angles and automatically “flag” images that look like potential maintenance needs (such as rust, damaged parts, etc).
According to Max, drones and mobile robots can capture very high-quality images and video data. A much bigger challenge for applying machine vision in industry is the scarity of skilled talent.
He believes that machine vision will be adopted by the heavy industry in three stages:
- Eliminating the need for humans to look at many hours of footage and analyze the data. (being done today)
- Automating the flow from the drone/robot operation to the analysis of the data. (two to three years in the future)
- Predictive maintenance – using historical data to predict when a part or equipment may fail (five years ahead)
Max stresses that before considering adoption businesses need to know the importance of understanding the feasibility of an AI application, especially in terms of data:
“The fantasy of AI being trained on a dataset apriori, being deployed and having a successful integration is just not going to happen. Companies have to plan on ‘learning’ being an ever-present process just like the metaphorical ‘human learning.”
Looking ahead five years into the future, Max believes that the hardware restrictions of carrying out machine vision on the edge (with the computing done on the drone itself) today may be overcome and that processes like the automation of drone navigation and imaging.
He sees a future where an operator can just ask a drone to go inspect a particular telecom tower and the drone would autonomously navigate to the tower, stop and gather only relevant information (like rusting or missing parts) and prompt necessary actions. Drones that just record video of areas in need of human attention would save a huge amount of storage space and time – and allow humans to focus only on the maintenance areas that might need attention.
He closes out by saying that although today only large companies with lots of consistent assets and a significant budget to handle cutting-edge tech of this sort, in 10 years even the middle tier companies may start adopting machine vision for maintenance in the industry.
Interview Highlights with Massimiliano Versace from Neurala
The main questions Max answered on this topic are listed below. Listeners can use the embedded podcast player (at the top of this post) to jump ahead to sections they might be interested in:
- (3:50) What is possible today with the machine vision infrastructure space?
- (5:50) What does the process of capturing image data using drones and robots entail and how much of this system is manually done today?
- (15:47) Five years from now what are some factors that might help the adoption so machine vision?
Subscribe to our AI in Industry Podcast with your favorite podcast service:
Header image credit: Adobe Stock