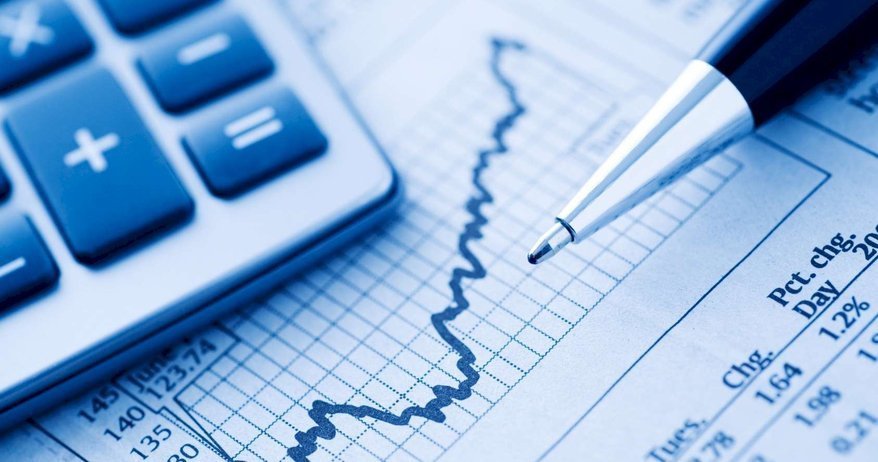
Reuters referenced a Stratistics MRC figure estimating the size of the business intelligence industry around $15.64 billion in 2016. It follows that AI would find its way into the business intelligence world. In our previous report, we covered 6 use-cases for AI in business intelligence. As of now, numerous companies claim to assist business leaders in the finance domain, specifically, in aspects of their roles using AI.
We researched the space to better understand where AI comes into play in business intelligence in the finance industry and to answer the following questions:
- What types of AI-based business intelligence applications are currently in use in the finance industry?
- What tangible results has AI-based business intelligence applications driven in finance?
- Are there any common trends among these innovation efforts? How could these trends affect the future of finance?
This report covers vendors offering software across three applications:
- Predictive Analytics
- Sentiment Analysis
- Customer Analytics
This article intends to provide business leaders in the finance space with an idea of what they can currently expect from Ai in their industry. We hope that this article allows business leaders in finance to garner insights they can confidently relay to their executive teams so they can make informed decisions when thinking about AI adoption. At the very least, this article intends to act as a method of reducing the time business leaders in finance spend researching AI companies with whom they may (or may not) be interested in working.
Predictive Analytics
RapidMiner
RapidMiner is a Boston-based company founded in 2007. The company offers a software called RapidMiner Platform, which they claim can help financial service businesses predict a customer’s lifetime value using machine learning.
RapidMiner claims users can integrate the RapidMiner platform with their existing enterprise sales systems, such as CRMs. The RapidMiner Platform can be input with data from CRMs or customer transactional data. Then, RapidMiner Platform uses machine learning to find patterns in the customer’s data, such as their purchase history and social media posts, to predict their possible next purchase. The system then provides customer service representatives at banks with the customer’s predicted lifetime value on the RapidMiner dashboard.
According to RapidMiner’s website, their predictive analytics platform can be used for several applications in banking, such as:
- Identifying patterns in a customer’s data to determine if they might have a high lifetime value
- Using that prediction to determine if other users also might have a high lifetime value
- Identifying major customer service issues from feedback forms or customer interactions with the bank through emails, calls, messages, and social media.
Below is a short 3-minute video demonstrating how Rapidminer Platform works:
RapidMiner claims to have helped PayPal improve customer satisfaction levels. RapidMiner claims PayPal deployed their platform to analyze a large quantity of customer feedback forms in several languages, including English, German, Portuguese, Spanish, Russian, Slavic, Chinese, Japanese and Korean. According to RapidMiner, PayPal was then able to identify the top clients and top promoters and detractors from the feedback data every month. The integration reportedly lasted around two to three months.
RapidMiner also lists Austria’s mobile phone service provider, Mo-bilkom Austria, as one of their past clients.
We could find no clear evidence of previous AI project or academic experience from RapidMiner’s executive leadership team. However, the company has raised over $36 million in funding so far, including from Ascent Venture Partners, Longworth Venture Partners, and NGP Capital.
Data Robot
DataRobot is a Boston-based company with over 400 employees. The company offers the Automated Machine Learning platform, which they claim can help banks and other lenders predict risk in lending or detect fraud using natural language processing (NLP).
The platform is reportedly trained on historical loan repayment records and social media data to predict a risk score for the likelihood of a customer defaulting on credit card payments. The company claims users can log into a dashboard showing columns and cells showing the key aspects of any particular loan application, including a risk score. DataRobot claims their platform can also clean and parse the data automatically and use machine learning models to predict the risk for each case. The system generates a score for each customer which factors in the customer’s credit history, social media profile, and personal information.
For instance, the system might raise a red flag if a customer has had a history of delayed repayments on an existing loan or if the customer’s transactions are in different geographical locations. The system then provides a risk analysis score and graphical representation of the analysis on their dashboard.
Below is a short 1-minute video demonstrating how DataRobot’s software works:
DataRobot claims to have helped Crest Financial predict credit defaults. Crest Financial’s two-person data science team was having trouble creating solutions for internal use due to how long it was taking them to prototype and test AI models. They deployed the DataRobot software, which reportedly generated risk models that outperformed the progress made by Crest’s data science team. According to DataRobot, Crest was then able to identify customers in high-risk markets, detect anomalies in customer transactions that might be fraudulent, and predict the likelihood of default for loan applicants. No statistics were provided in the case study.
DataRobot’s also lists Evariant and DonorBureau [some] of their past clients.
Gourab De is VP of Data Science at DataRobot. He holds a PhD in Biostatistics from Harvard University. Previously, he served as a data scientist at Ginger.io.
Domo
Domo offers a software which they claim can help financial institutions businesses gain business intelligence insights from data collected by business software, such as CRMs, using machine learning.
Domo claims users can integrate data from their enterprise financial systems, such as SalesForce, NetSuite, SAP, Concur, or Quickbooks into the Domo software. Then, Domo can analyze the data coming in from across the systems using data science to identify key metrics for the company, such as profitability from SAP data combined with SalesForce or NetSuite data. The system then provides an overview of several company performance indicators on a dashboard, and users can look at each of the metrics in more detail.
Below is a short 7-minute video demonstrating how Domo works:
Domo claims to have helped Check Into Cash establish robust KPI’s for indicating the real-time performance of loans. Check into Cash was using weekly manual reports for measuring the performance of their short-term, small-dollar credit solutions. According to Domo, Check Into Cash was then alerted about lower returns on loans, yet there was no quantifiable result mentioned for the project.
Domo also lists DHL, MasterCard, eBay, and National Geographic as some of their past clients.
Although Domo seems to have employed data scientists, we were unable to find any C-level executives with AI experience on the company’s team.
Sentiment Analysis
Amenity Analytics
Amenity Analytics is a New York-based company that offers a cloud-based text analytics API service, which they claim can help banks and financial institutions analyze customer sentiments using natural language processing.
Amenity Analytics claims users can integrate their text AI service into their existing software network. Employees at financial institutions might sign into a web portal to see customer sentiment insights (such as positive responses for an online banking system) generated by the software from real-time and historical social media data. Then, the software parses through social media mentions for the finance company and uses NLP and machine learning to extract key customer sentiments by scoring each post.
The software identifies the most relevant topics of discussion, such as customer feedback, from the data, and assigns a score and topic label to posts based on the number of positive or negative words or phrases in it. The system then provides an output in the web portal for the key topics of discussion and which discussions were positive or negative.
Below is a short 3-minute video demonstrating how Amenity Analytics’s software works:
The company does not seem to make any case studies available reporting success with their software.
Amenity Analytics lists Intel and Time Warner as some of their past clients.
Ronen Feldman is Chief Data Scientist at Amenity Analytics. He holds a PhD in Computer Science from Cornell University. Feldman is also currently serving as head of the data science department at the Hebrew University Business School and as an AI expert for the European Commission.
Customer Analytics
Avanade
Avanade is a Seattle-based company with over 12,000 employees. The company is a joint venture between Microsoft and Accenture that leverages the Cortana Intelligence Suite. The company offers a predictive analytics software which they claim can help financial institutions automate, digitize, and streamline compliance processes and help create custom trading tools using data science and machine learning.
Avanade claims businesses can work with their team to build analytics platforms that can give their employees perspective and insight on their business. A financial firm might leverage Avanade’s solution to gain insights from customer data and transactional data to help drive more growth. Avanade software uses NLP and machine learning to extract and identify trends from the customer personal data and transactional history data, such as ways to improve regulatory compliances or predict customer behavior to improve engagement. The system then provides a list of these recommendations as alerts on the Avande dashboard.
Below is a short 3-minute video demonstrating how Avanade’s software works:
Avanade seems to have worked in projects with several companies across industry sectors and includes some in their case studies. Avanade claims to have helped DNV GL, a Norwegian accredited registrar and classification society, to develop a data management structure for business intelligence insights. Avanade claims analysts at DNV GL were using Power BI for their business intelligence analytics and were facing challenges such as unintended data loss.
DNV GL engaged Avanade to deliver a report with recommendations for creating a governance model aimed at reducing data loss risk and helping employees make the right decisions about how and when to share internal enterprise data. Avanade also claims their detailed report enabled DNV GL to increase the number of Power BI users including non-technical staff.
Avanade also lists Business Development Bank of Canada and DNV GL as some of their past clients.
Tim Groulx is Director and Lead Artificial Intelligence Architect at Avanade. We could not determine whether or not he a PhD or MS, although he has previously served as a senior data architect at Microsoft and Nielson, where he worked on data management projects.
Takeaways for Business Leaders in Finance
Based on the companies we researched for this report, it seems as though predictive analytics applications in finance seem to have the most traction. Financial institutions can predict risk or customer behavior to directly affect revenue and several established use-cases are now available for this application.
DataRobot seems to have the most traction. The company has raised $124.6 million in funding so far including a $67 million Series C round from New Enterprise Associates. This might be down to the fact that DataRobot has several well-documented case studies which clients can easily access. The key for AI vendors to expand their business in this space seems to be in clearly communicating the ROI for financial institutions.
In the next two to five years, advancements in NLP, text mining, and machine learning might make it possible for financial institutions to fully utilize all the structured and unstructured data they collect. AI systems can be used to sift through vast amounts of data rapidly to gather insights leading to improvements in customer service, trading strategies, predicting risk etc.
In the near term, financial institutions should not expect to easily be able to automate their business processes or gain business intelligence from their data without embarking on a lengthy integration process starting with managing and organizing their data. This might additionally require discussions with vendor support representatives and a large upfront costs. The largest enterprises may have the budget and staff to parse the technology, but based on our research, it is as of right now accessible to companies that would be able to afford AI applications.
Header Image Credit: epho