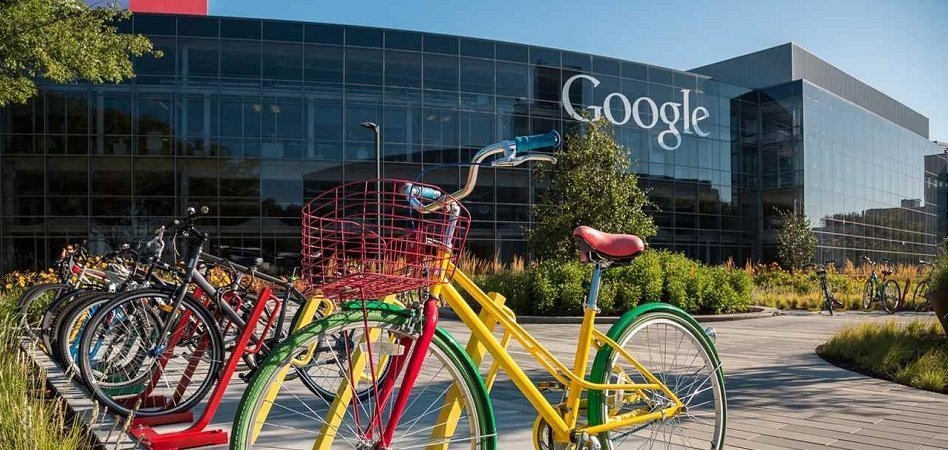
If one tunes into social media, it's easy to be convinced that every AI startup is making a lot of money, making a big difference in the companies that they're working with, and driving real revenue. However, as we've mentioned many times here at Emerj, most AI applications are really in pilot mode.
There's nothing wrong with that, but even companies that have raised $50 million are still very much iterating on what their product really is, how they have to sell it into the enterprise, who their exact customer is, and what their core value proposition is. They're still filling these things out in large part, and they're living off venture money, not off of revenue by solving real problems for their clients.
It won't always be that way, but these are the relatively early days of AI, and this is very much the state of affairs. There are really two groups of people who are making all the money when it comes to the fruitful use of artificial intelligence, either as a buzzword or as ...
You've landed on exclusive content for Emerj Plus Members
Emerj Plus Membership
In-Depth Analysis
Consistent coverage of emerging AI capabilities across sectors.
Exclusive AI Capabilities Matrix
An explorable, visual map of AI applications across sectors.
Exclusive AI White Paper Library
Every Emerj online AI resource downloadable in one-click
Best Practices and executive guides
Generate AI ROI with frameworks and guides to AI application